Rolling bearing fault feature extraction via improved SSD and a singular-value energy autocorrelation coefficient spectrum
MEASUREMENT SCIENCE AND TECHNOLOGY(2022)
摘要
It is usually difficult to extract weak fault features from rolling bearing vibration signals under noise pollution. To address this problem, a fault feature extraction approach for rolling bearings using improved singular spectrum decomposition (SSD) and a singular-value energy autocorrelation coefficient spectrum (SVEACS) is proposed. Firstly, to facilitate the determination of the optimal modal parameters in the SSD algorithm, the number of SSD layers is adaptively determined using an improved SSD algorithm based on permutation entropy. Then, the optimal modal components are selected, and the proposed SVEACS is used to determine the order of singular-value noise reduction. Finally, envelope analysis is used to extract the accurate shock characteristics of the denoised signal. The results of the experiments on simulated and real signals indicate that the proposed method can effectively extract the weak characteristics of the vibration signal under strong noise, and accurately diagnose the fault of a rolling bearing.
更多查看译文
关键词
feature extraction, rolling bearing, improved singular spectrum decomposition, singular value energy autocorrelation coefficient spectrum
AI 理解论文
溯源树
样例
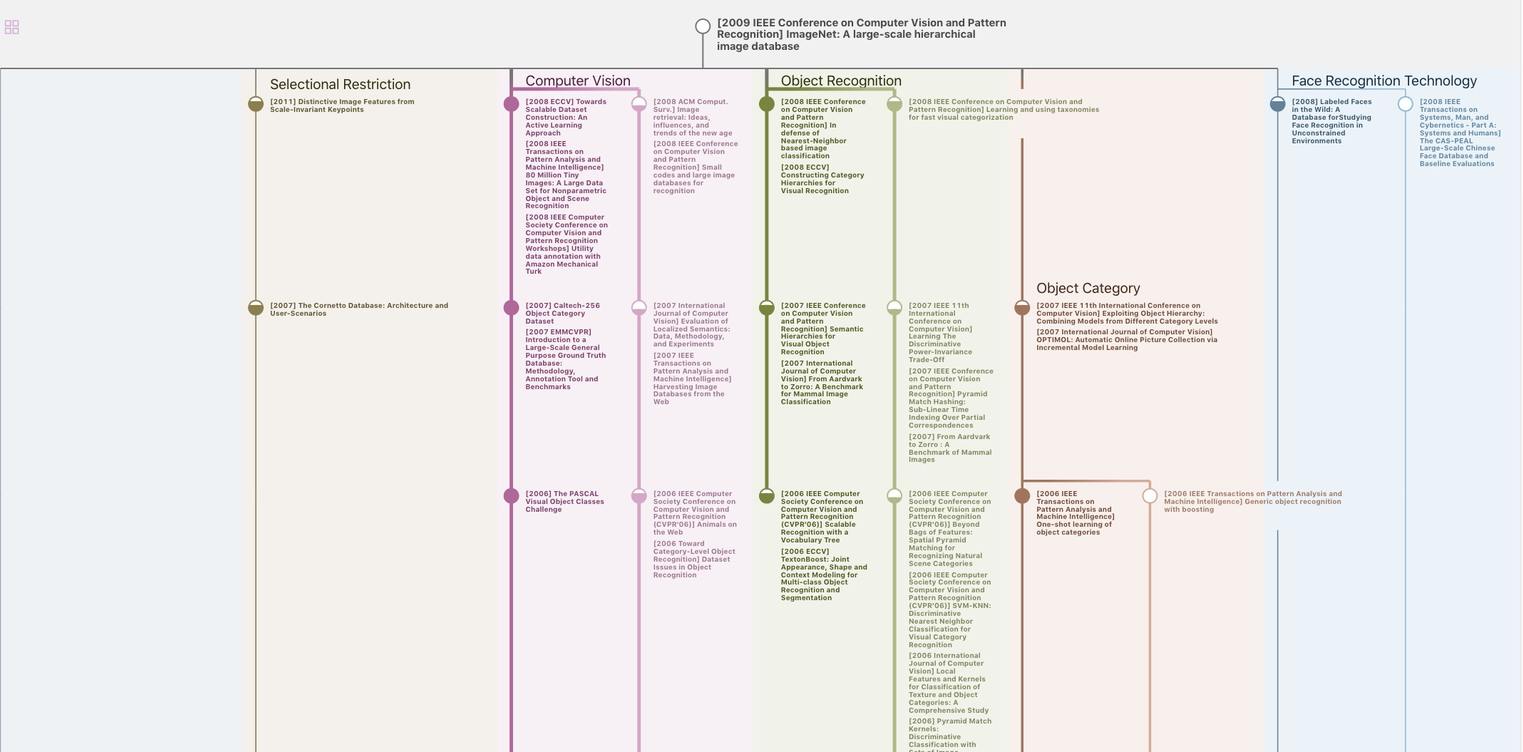
生成溯源树,研究论文发展脉络
Chat Paper
正在生成论文摘要