NQRELoc: AP Selection via Nonuniform Quantization RSSI Entropy for Indoor Localization
IEEE Sensors Journal(2022)
Abstract
Received signal strength indicator (RSSI) of WiFi access point (AP) is a primary sensor data used for indoor fingerprint localization. User sends its online RSSI to server to estimate its position by matching with RSSI fingerprints database which built in the offline phase. An important goal of indoor fingerprint localization is to increase the accuracy while reduce the storage cost. Meanwhile, APs perform different effects on target estimation and mapping metric in RSSI fingerprint localization. In this paper, a novel localization model based on the nonuniform quantization RSSI entropy (NQRELoc) is proposed to address these problems. First, to select the APs that contribute more to the localization, the nonuniform quantization RSSI entropy (NQRE) is introduced to quantify AP’s discernibility and select APs whose signals show sufficient differentiation to construct an offline fingerprints database. Then, the entropy-weighted euclidean distance (EWED) is used as a metric to measure the similarity of online RSSI vectors and offline RPs fingerprints. Finally, NQRELoc locates the target by the improved entropy-weighted K nearest neighbor (IEWKNN) algorithm, which takes the APs effect into target estimation. To validate the proposed algorithm, a large scale of experiments and simulations are implemented. The results demonstrate that NQRELoc can not only reduce the storage overhead but also improve the positioning accuracy compared to the other existing techniques.
MoreTranslated text
Key words
Indoor localization,AP selection,nonuniform quantization RSSI entropy,improved entropy-weighted KNN
AI Read Science
Must-Reading Tree
Example
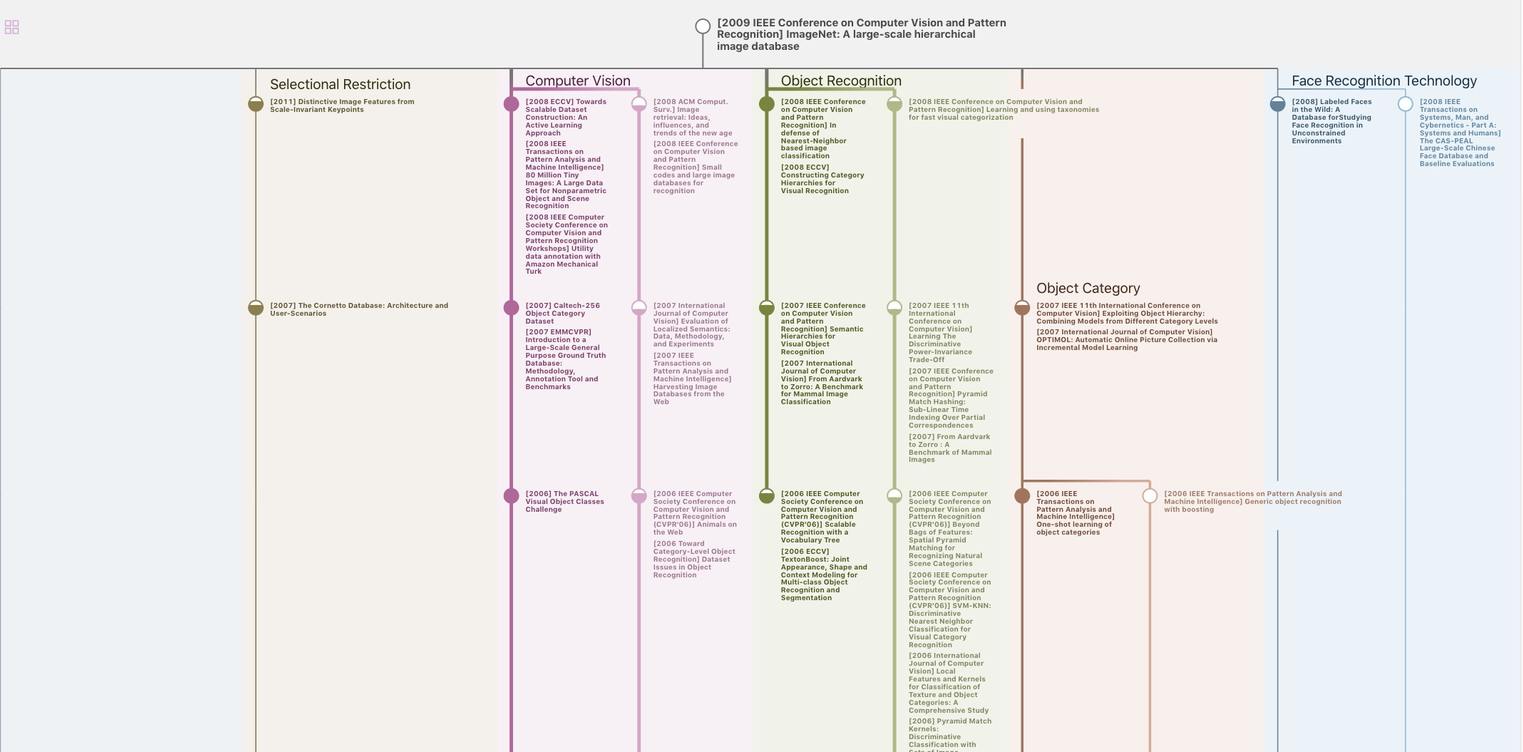
Generate MRT to find the research sequence of this paper
Chat Paper
Summary is being generated by the instructions you defined