Building Classification Using Random Forest to Develop a Geodatabase for Probabilistic Hazard Information
NATURAL HAZARDS REVIEW(2022)
Abstract
To understand the community risk from severe weather threats, two components, including weather information and community assets, are crucial. Recently, probabilistic hazard information (PHI) from the National Oceanic and Atmospheric Administration (NOAA) Forecasting a Continuum of Environmental Threats (FACETs) program has been developed to provide dynamic weather-related information between the watch and warning systems to weather forecasters, emergency management agencies, and the public. To predict community physical risks on critical infrastructure and building properties using PHI, building type information is required. This study applied a machine learning technique to predict building types using building footprint and city zoning data. We collected Oklahoma county building property data to train and test a random forest model. The result of this study showed that building footprint and city zoning data can be applied to classify multiple building types with an accuracy of 96%. The machine learning-based building classification contributed to the acquisition of building type data in the Oklahoma City, Oklahoma, metropolitan area. This geodatabase will be utilized to predict real-time critical infrastructure and building damage assessment using PHI. In addition to their importance to physical building damage assessment, the results can be utilized to develop postdisaster responses and planning.
MoreTranslated text
Key words
Probabilistic hazard information (PHI), Severe weather threats, Building classification, Random forest, Building damage assessment
AI Read Science
Must-Reading Tree
Example
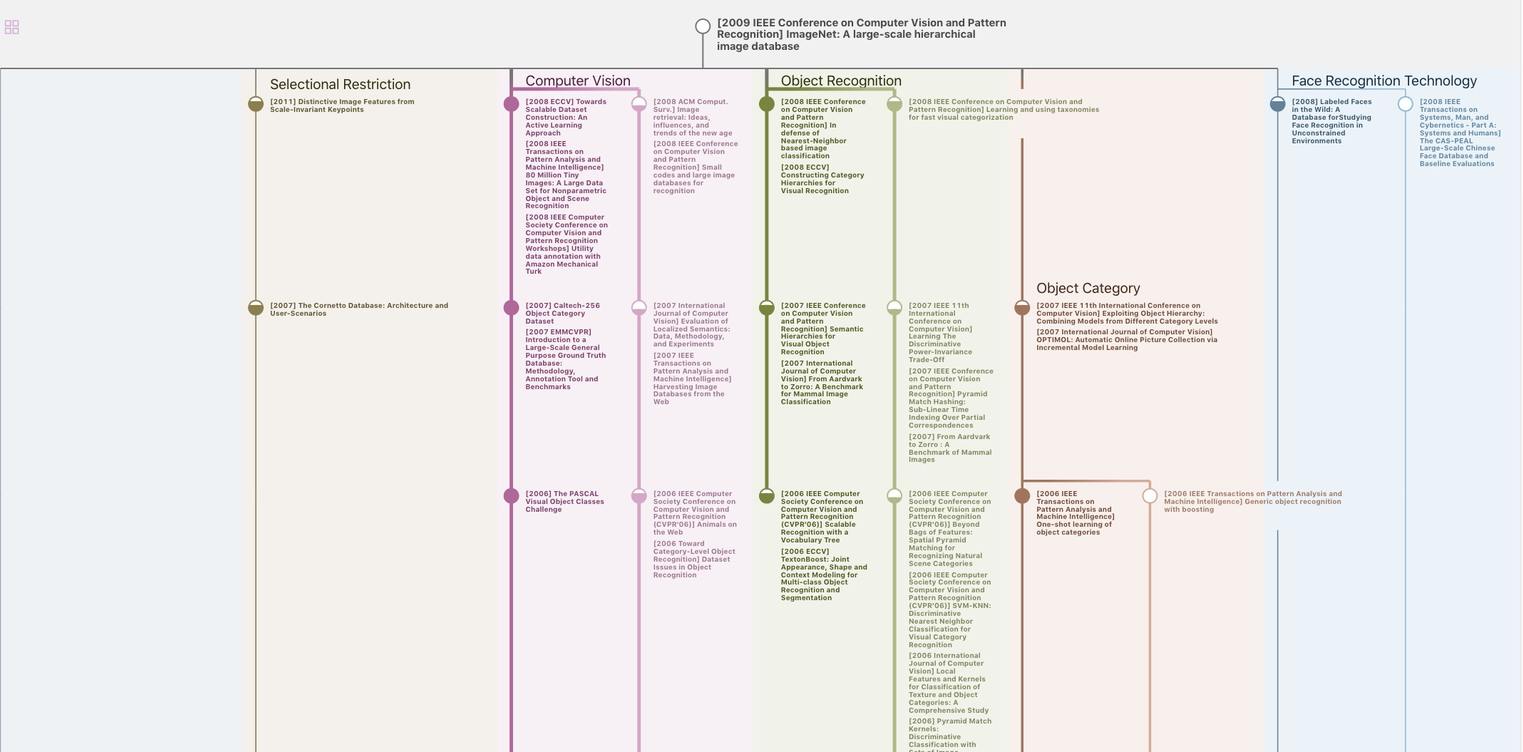
Generate MRT to find the research sequence of this paper
Chat Paper
Summary is being generated by the instructions you defined