ASQ-FastBM3D: An Adaptive Denoising Framework for Defending Adversarial Attacks in Machine Learning Enabled Systems
IEEE Transactions on Reliability(2023)
摘要
Machine learning has made significant progress in image recognition, natural language processing, and autonomous driving. However, the generation of adversarial examples has proved that the machine learning system is unreliable. By adding imperceptible perturbations to clean images can fool the well-trained machine learning systems. To solve this problem, we propose an adaptive image denoising framework Adaptive Scalar Quantization (
ASQ-FastBM3D
). The
ASQ-FastBM3D
framework combines the
ASQ
method with the
FastBM3D
algorithm. The adaptive scalar quantization is the improvement of scalar quantization, which is used to eliminate most of the perturbations.
FastBM3D
is proposed to improve the quality of the quantified image. The running time of
FastBM3D
is 50% less than that of
BM3D
. Compared with some traditional filter methods and some state-of-the-art neural network methods for recovering the adversarial examples, the accuracy rate of our
ASQ-FastBM3D
method is 99.73% and the F1 score is 98.01%, which is the highest.
更多查看译文
关键词
Adversarial example,BM3D,image denoising,machine learning,scalar quantization
AI 理解论文
溯源树
样例
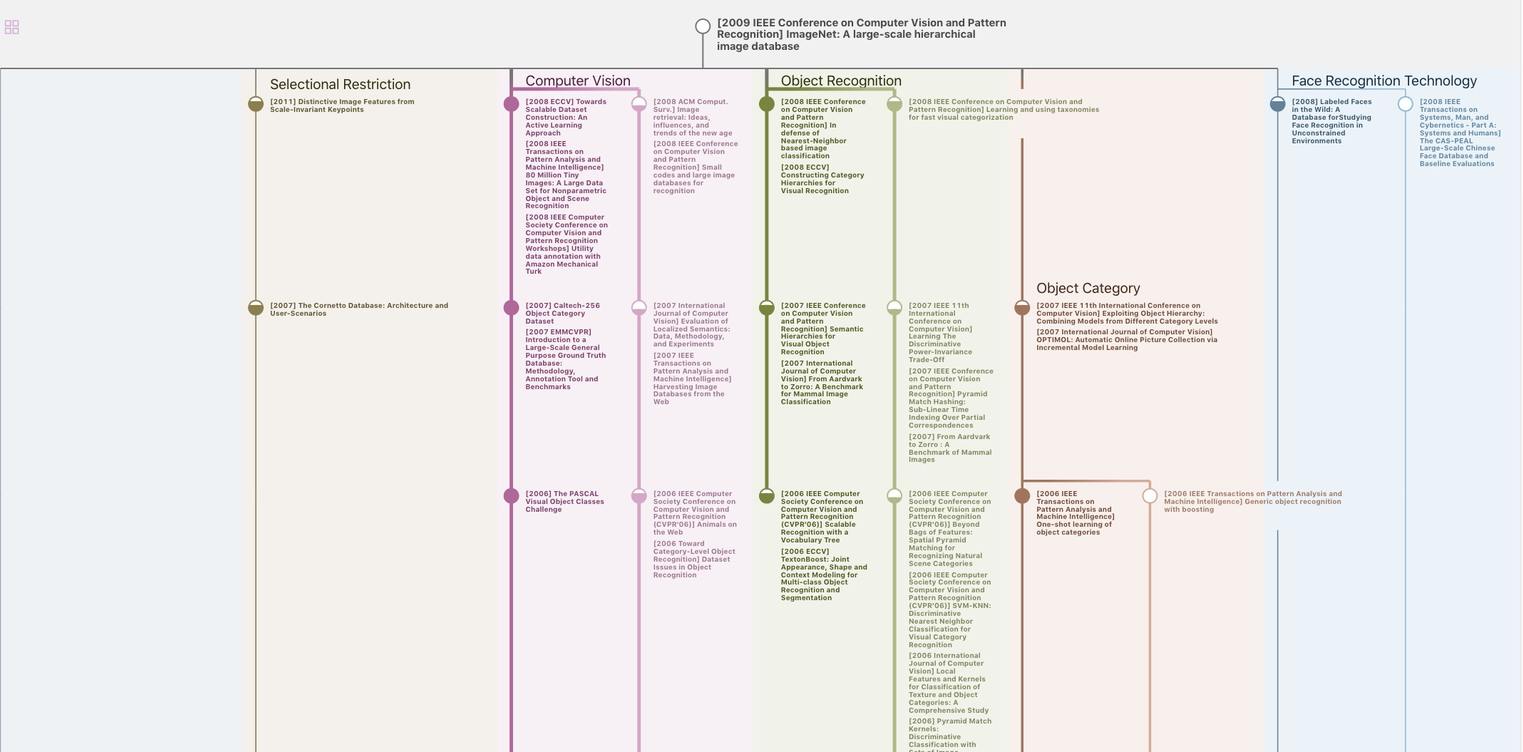
生成溯源树,研究论文发展脉络
Chat Paper
正在生成论文摘要