Admission Control for 5G Core Network Slicing Based on Deep Reinforcement Learning
IEEE SYSTEMS JOURNAL(2022)
摘要
Network slicing is a promising technology for providing customized logical and virtualized networks for the fifth-generation (5G) use-cases (enhanced mobile broadband, ultrareliable low-latency communications, and massive machine-type communications), which pose distinct quality of service (QoS) requirements. Admission control and resource allocation mechanisms are pivotal for realizing network slicing efficiently, but existing mechanisms focus on slicing the radio access network. This article proposes an approach encompassing intelligent and efficient mechanisms for admission control and resource allocation for network slicing in the 5G core network. The admission control mechanism introduces two solutions, one based on reinforcement learning (called SARA) and the other based on deep reinforcement learning (called DSARA). SARA and DSARA consider the QoS requirements of 5G use-cases, differentiate network core nodes from edge nodes, and process slice requests in time windows to favor the service provider's profit and resource utilization. Results show that SARA and DSARA overcome existing mechanisms for managing admission control and resource allocation in 5G core network slicing.
更多查看译文
关键词
5G mobile communication, Network slicing, Resource management, Admission control, Ultra reliable low latency communication, Substrates, Computer architecture, Admission control, deep reinforcement learning, fifth-generation (5G), network slicing, resource allocation, reinforcement learning
AI 理解论文
溯源树
样例
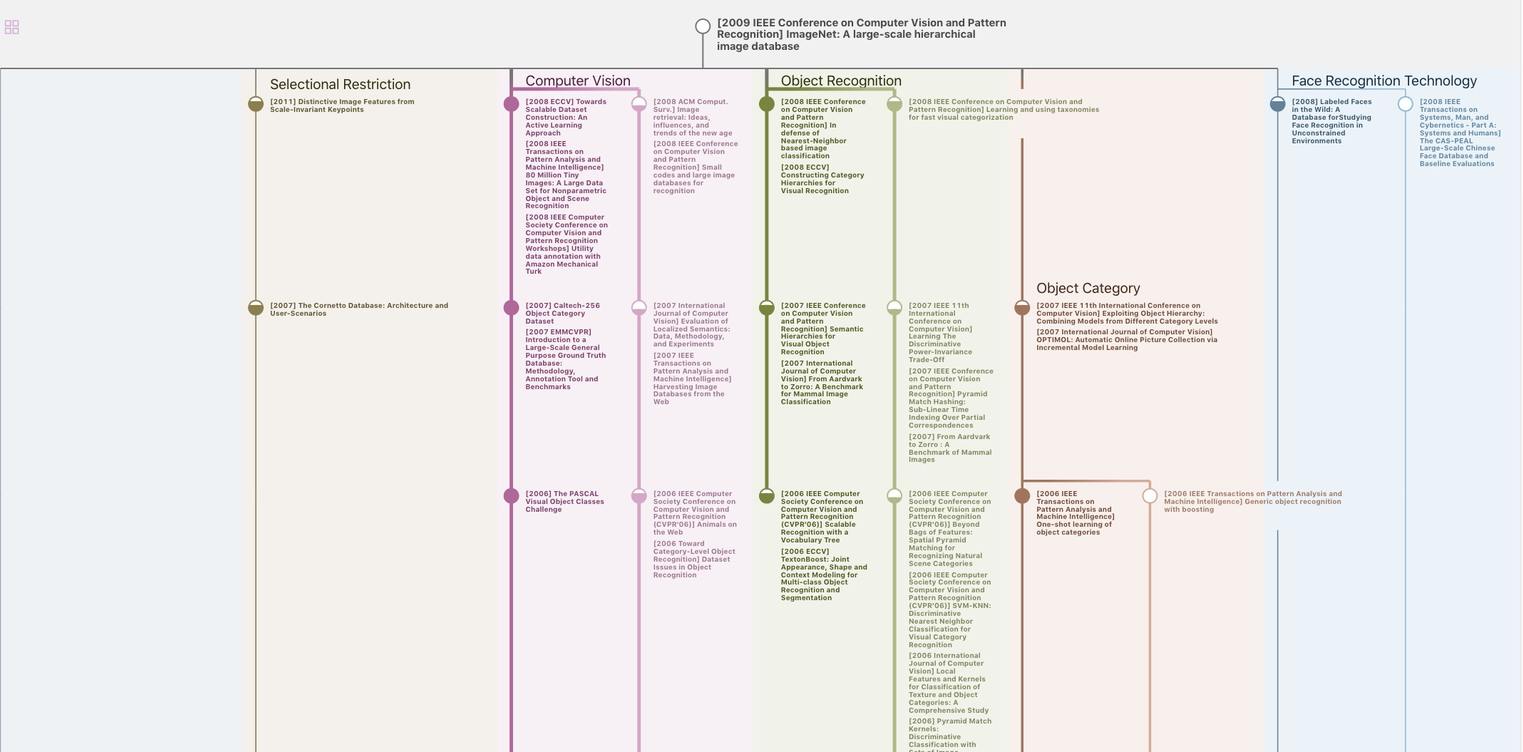
生成溯源树,研究论文发展脉络
Chat Paper
正在生成论文摘要