Prediction of phase and hardness of HEAs based on constituent elements using machine learning models
MATERIALS TODAY COMMUNICATIONS(2022)
摘要
The phase and hardness of high entropy alloys (HEA) were predicted using machine learning models trained on large experimental datasets. The chemical composition was used as the set of input features. For phase prediction, a neural network was trained on 775 experimental samples and yielded a 93.4% prediction accuracy. In addition, the hardness of HEAs has been predicted using an ensemble model trained on an unprecedented large hardness dataset of 427 samples. The model showed an average regression value of 0.88, and most of the predicted values were within the 20% error region. Moreover, sensitivity analysis suggest that HEAs hardness is affected the most by Cu, Ti, Co, and Ni concentration (respectively). Due to the testing dataset size, and the aforementioned accuracy, the phase and hardness model may be reliably used for prediction and screening purposes.
更多查看译文
关键词
High entropy alloy, Artificial neural networks, Regression model, Ensemble model, Chemical composition
AI 理解论文
溯源树
样例
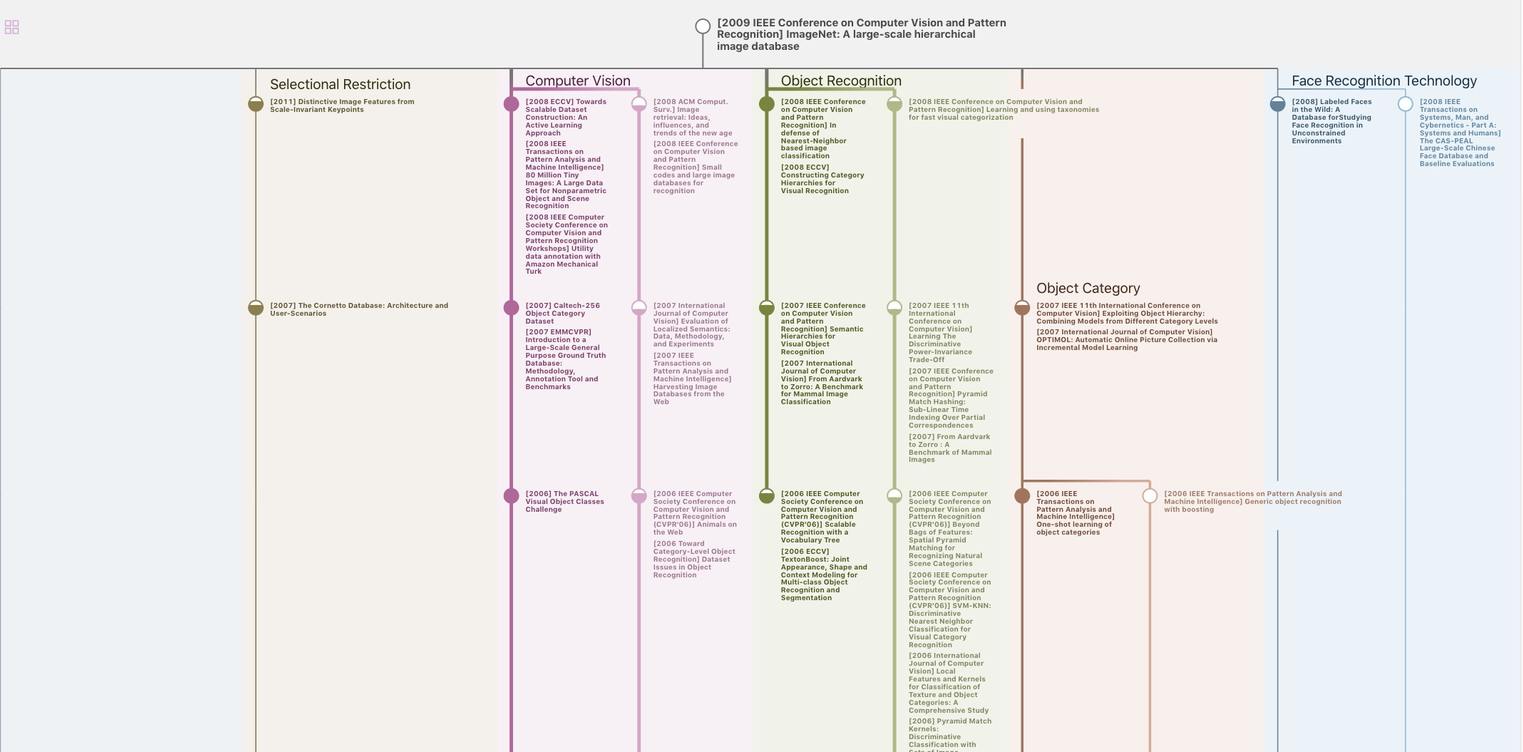
生成溯源树,研究论文发展脉络
Chat Paper
正在生成论文摘要