STAF: Spatio-Temporal Attention Framework for Understanding Road Agents Behaviors
IEEE ACCESS(2022)
摘要
On-road behavior analysis is a key task required for robust autonomous vehicles. Unlike traditional perception tasks, this paper aims to achieve a high-level understanding of road agent activities. This allows better operation under challenging contexts to enable better interaction and decision-making in such complex environments. In this paper, we tackle the problem of discriminating spatio-temporal features that capture the visual instants that require more attention. We propose a new approach called STAF (Spatio-Temporal Attention Framework) through Long Short Term Memory (LSTM) layers that uses a multi-head attention mechanism on its past cell state to focus on attributes that are relevant over time. Experiments have been carried out on two different scenarios over data from Joint Attention in Autonomous Driving (JAAD) and Honda Research Institute Driving Dataset (HDD), both datasets devoted to understanding the behavior of road agents. The evaluation and results obtained proof that the proposed "STAF" is outperforming state-of-the-art algorithms-based LSTM (Ramanishka, Rasouli, and LSTM-baseline). For example, STAF outperforms LSTM with mean Average Precision (mAP) of 13%.
更多查看译文
关键词
Roads, Behavioral sciences, Task analysis, Vehicles, Trajectory, Visualization, Long short term memory, Deep LSTM, attention mechanism, STAF, road scenes, behaviors understanding, smart mobility
AI 理解论文
溯源树
样例
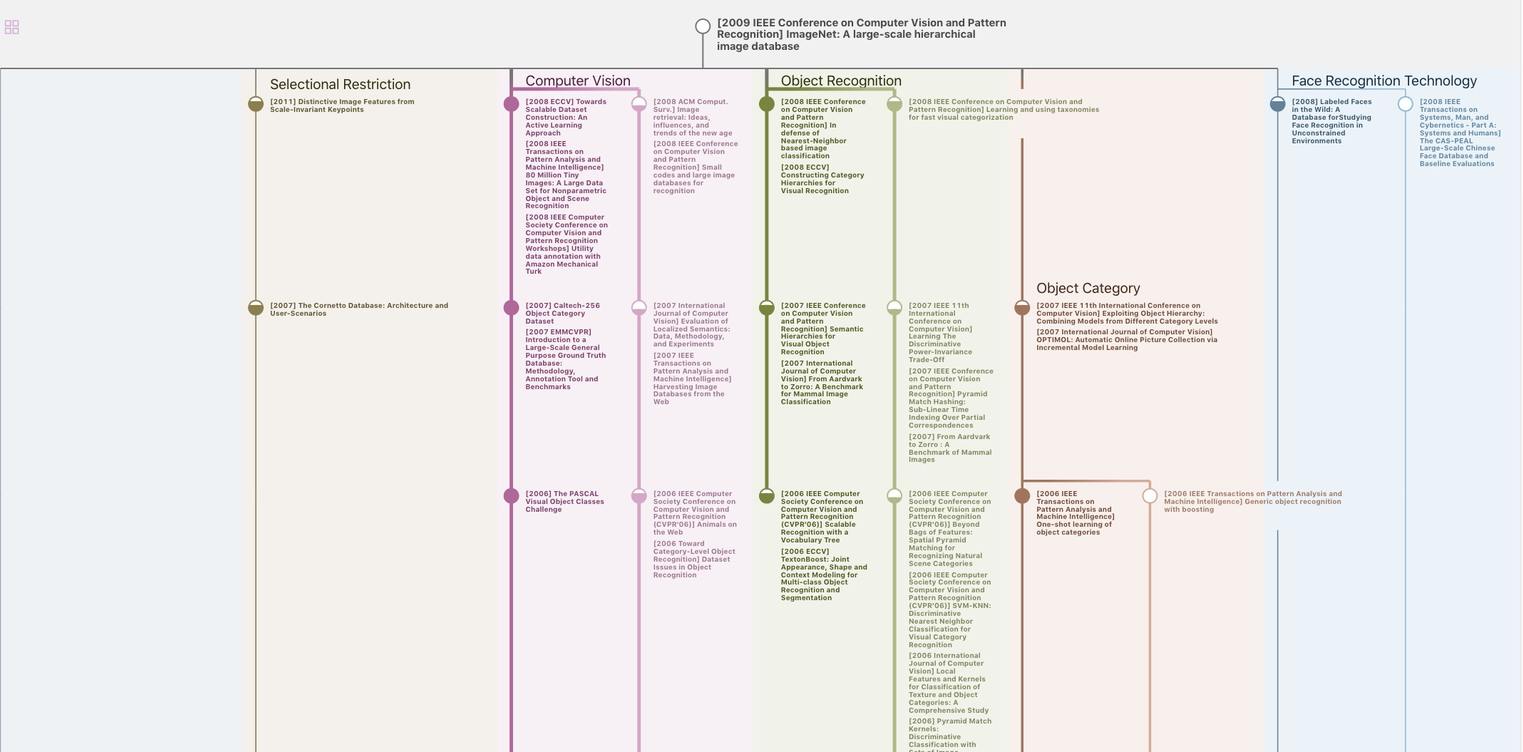
生成溯源树,研究论文发展脉络
Chat Paper
正在生成论文摘要