DRIQA-NR: no-reference image quality assessment based on disentangled representation
Signal, Image and Video Processing(2022)
Abstract
Due to the characterization capabilities of deep features, image quality assessment (IQA) methods based on convolutional neural networks (CNNs) have been proposed. However, the existing CNN-based IQA does not make full use of deep features. So, we propose a novel no-reference image quality assessment based on disentangled representation (DRIQA-NR), which decomposes the deep features extracted from distorted images into content features and distortion information features. The content features are used to restore the input image. To eventually predict the quality of the image, features extracted from the restored image and the distorted image are merged with the distortion information feature. In addition, the distortion information features can also be used to improve the performance of full-reference image quality assessment. Experiments on LIVE, CSIQ and TID2013 suggest that the method proposed achieves favorable performance against other methods, with higher average Spearman’s rank-order correlation coefficient, Pearson’s linear correlation coefficient, Kendall rank-order correlation coefficient values and lower root mean square error.
MoreTranslated text
Key words
No reference, Image quality assessment, Disentangled representation, Deep features
AI Read Science
Must-Reading Tree
Example
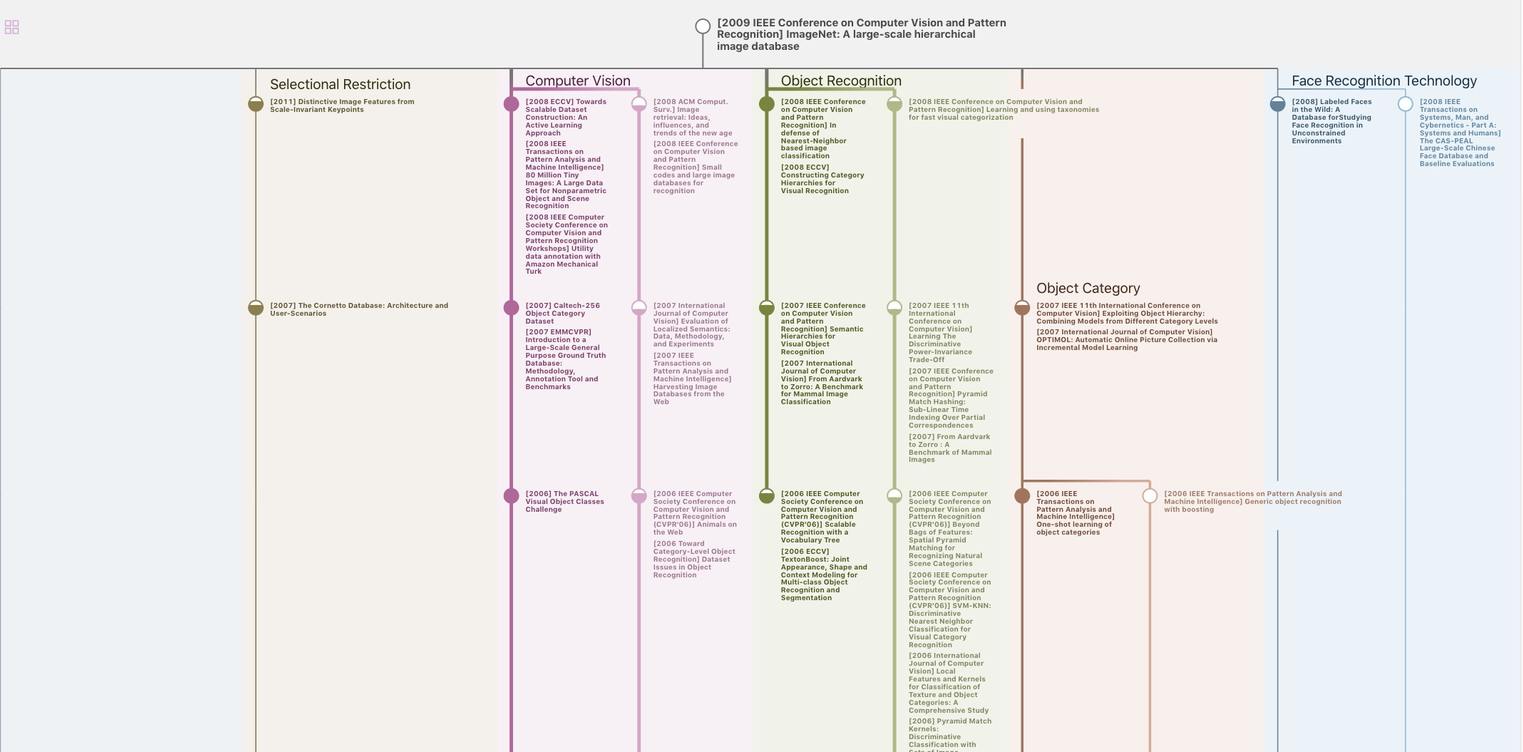
Generate MRT to find the research sequence of this paper
Chat Paper
Summary is being generated by the instructions you defined