Self-supervised monocular depth estimation in dynamic scenes with moving instance loss
Engineering Applications of Artificial Intelligence(2022)
摘要
Estimating depth from monocular images is a powerful method to perceive valuable environmental information, which is essential for applications that require three-dimensional (3D) environmental models such as autonomous driving and virtual reality. The monocular self-supervised depth estimation method based on deep learning has made rapid progress without depth ground truth information. However, the existing methods are based on the assumption of a static world during training, and depth estimation in a dynamic environment needs further development. To solve this problem, we propose a new monocular self-supervised depth estimation method in dynamic scenes to eliminate the negative impact of moving objects in the image sequence when calculating the self-supervised loss. Specifically, for the self-supervised depth estimation framework, we propose a moving object mask based on the minimum instance photometric residual and then combine it with the mask based on instance re-projection residual in the existing instance-level moving object segmentation methods. In addition, we design a moving instance loss function to process the moving object, so that the training of the model can achieve better performance. Experiments are conducted on public datasets to verify the effectiveness of the proposed method and each of its components, and the results show that our method achieves better performance for depth estimation in dynamic scenes compared to state-of-the-art methods.
更多查看译文
关键词
Monocular depth estimation,Dynamic scenes,Moving instance loss,Minimum instance photometric residual
AI 理解论文
溯源树
样例
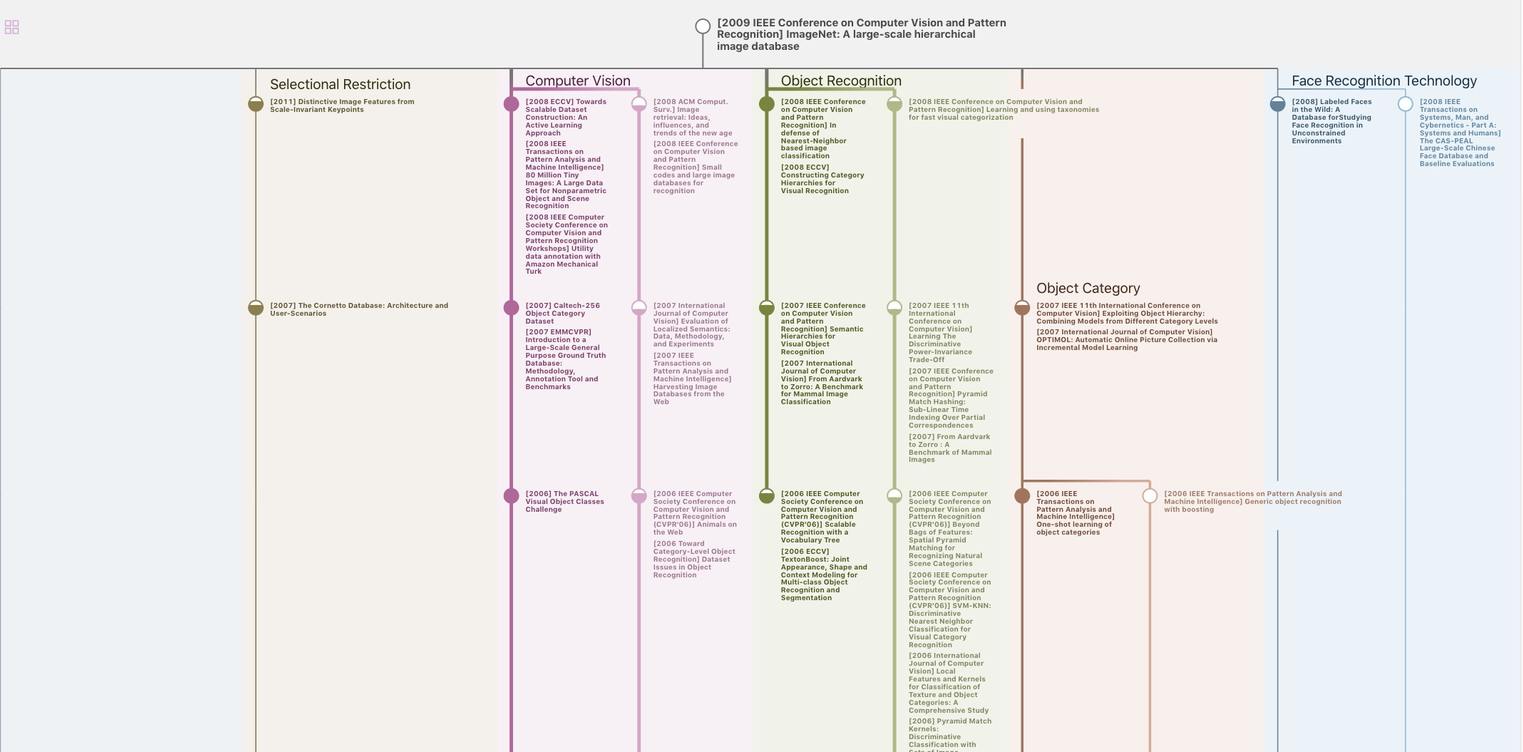
生成溯源树,研究论文发展脉络
Chat Paper
正在生成论文摘要