Hybrid dilated faster RCNN for object detection
JOURNAL OF INTELLIGENT & FUZZY SYSTEMS(2022)
摘要
Object detection is a very important part of computer vision, and the most common method of object detection is the Faster region convolutional neural network (RCNN), which uses CNN to extract image features. However, the parameters to be learned in CNN are enormous and may affecting the efficiency. In this paper, hybrid dilated Faster RCNN (HDF-RCNN) is proposed to solve this problem, and the main contributions are: 1) HDF-RCNN is built through replacing the VGG16 in Faster RCNN with HDC (hybrid dilated CNN) to achieve a fast and accurate object detection algorithm, and the LeakyReLU activation function is used to increase the ability of mapping input information; 2) the portability of HDC, namely, the possibility of embedding the HDC into object detection network with independent feature extraction part is verified. The Microsoft COCO data set is used to verify the performance of HDF-RCNN, and the experiments show that, compared with the traditional Faster RCNN, the testing accuracy of HDF-RCNN is averagely improved by 7.11%, the training loss and training time are averagely reduced by 40.06% and 34.29%, respectively. Therefore, the HDF-RCNN can significantly improve the efficiency of object detection and the HDC can be used as an independent feature extraction network to adapt to many different frameworks.
更多查看译文
关键词
object detection, hybrid dilated convolution, faster RCNN
AI 理解论文
溯源树
样例
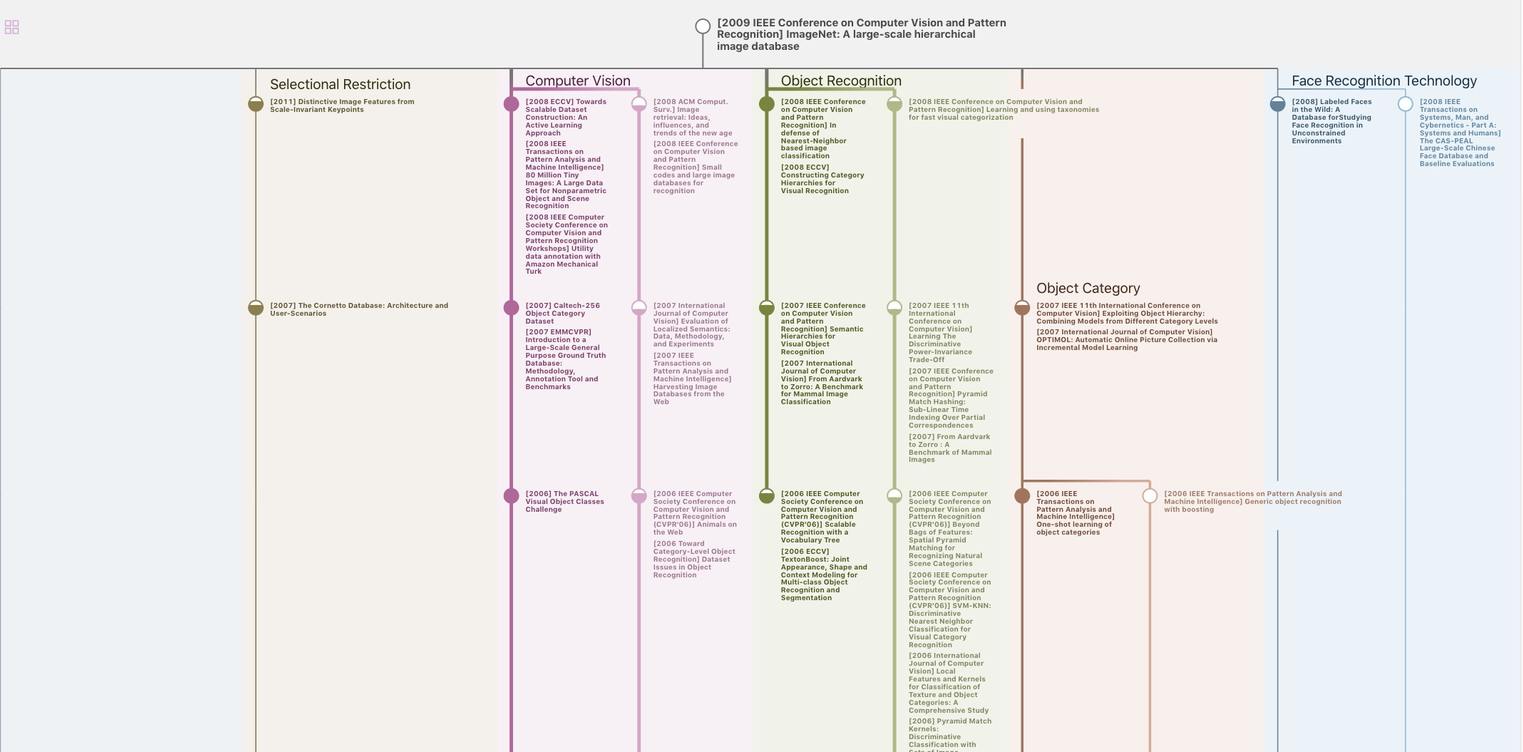
生成溯源树,研究论文发展脉络
Chat Paper
正在生成论文摘要