Urban physics and outdoor thermal comfort for sustainable street canyons using ANN models for composite climate
ALEXANDRIA ENGINEERING JOURNAL(2022)
摘要
This study is focused on the development and validation of the Artificial Neural Network (ANN) model for the prediction of hourly values of thermal comfort indices, namely - Physiological Equivalent Temperature (PET) and the Universal Thermal Climate Index (UTCI). Two ANN models are developed - one using air temperature, wind speed, relative humidity and mean radiant temperature as meteorological input parameters along with date and time. Another uses air temperature as only meteorological input parameters and date and time. The applicability of ANN model was validated for peak winter (December - February) and summer months (March May). The ANN model showed remarkable predictive ability through a high coefficient of correlation, low root mean square error (RMSE) and fitting values of true predicted rate (TPR), false alarm rate (FAR) and success index (SI). The predicted output from the validated ANN models is used to discuss the influence of optimum urban physics parameters on outdoor thermal comfort. This study establishes that air temperature can be used as the only microclimatic input parameter to predict PET and UTCI using ANN when recording other microclimatic parameters is difficult.(c) 2022 THE AUTHORS. Published by Elsevier BV on behalf of Faculty of Engineering, Alexandria University This is an open access article under the CC BY-NC-ND license (http://creativecommons.org/ licenses/by-nc-nd/4.0/).
更多查看译文
关键词
Artificial Neural Network, Outdoor, Thermal comfort, Urban, Microclimate
AI 理解论文
溯源树
样例
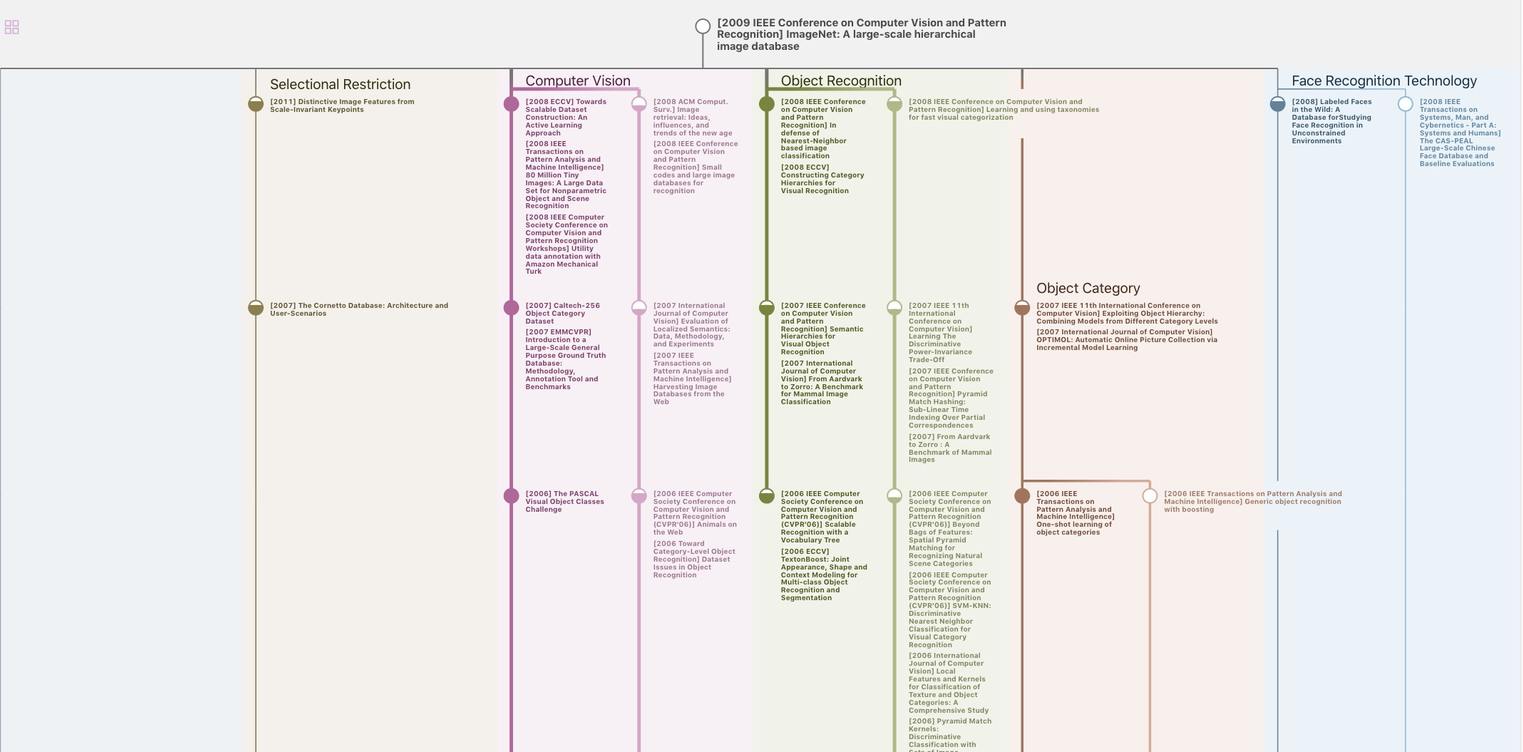
生成溯源树,研究论文发展脉络
Chat Paper
正在生成论文摘要