Spatial-Temporal Invariant Contrastive Learning for Remote Sensing Scene Classification
IEEE GEOSCIENCE AND REMOTE SENSING LETTERS(2022)
Abstract
Self-supervised learning achieves close to supervised learning results on remote sensing image (RSI) scene classification. This is due to the current popular self-supervised learning methods that learn representations by applying different augmentations to images and completing the instance discrimination task which enables convolutional neural networks (CNNs) to learn representations invariant to augmentation. However, RSIs are spatial-temporal heterogeneous, which means that similar features may exhibit different characteristics in different spatial-temporal scenes. Therefore, the performance of CNNs that learn only representations invariant to augmentation still degrades for unseen spatial-temporal scenes due to the lack of spatial-temporal invariant representations. We propose a spatial-temporal invariant contrastive learning (STICL) framework to learn spatial-temporal invariant representations from unlabeled images containing a large number of spatial-temporal scenes. We use optimal transport to transfer an arbitrary unlabeled RSI into multiple other spatial-temporal scenes and then use STICL to make CNNs produce similar representations for the views of the same RSI in different spatial-temporal scenes. We analyze the performance of our proposed STICL on four commonly used RSI scene classification datasets, and the results show that our method achieves better performance on RSIs in unseen spatial-temporal scenes compared to popular self-supervised learning methods. Based on our findings, it can be inferred that spatial-temporal invariance is an indispensable property for a remote sensing model that can be applied to a wider range of remote sensing tasks, which also inspires the study of more general remote sensing models. The source code is available at https://github.com/GeoX-Lab/G-RSIM/tree/main/T-SC-STICL.
MoreTranslated text
Key words
Task analysis, Remote sensing, Image analysis, Image color analysis, Training, Supervised learning, Histograms, Deep learning, remote sensing, scene classification, self-supervised learning
AI Read Science
Must-Reading Tree
Example
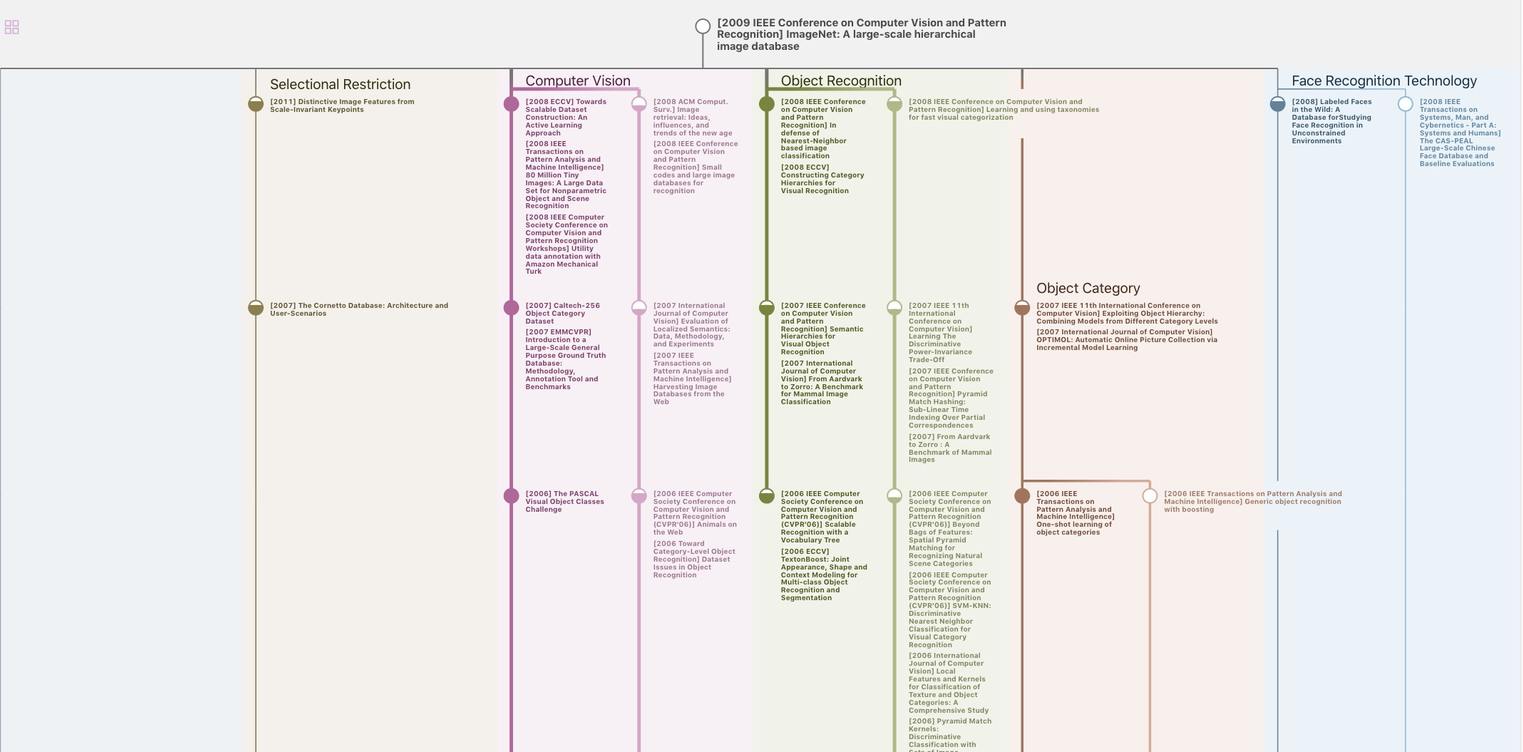
Generate MRT to find the research sequence of this paper
Chat Paper
Summary is being generated by the instructions you defined