Classifying the posture and activity of ewes and lambs using accelerometers and machine learning on a commercial flock
Applied Animal Behaviour Science(2022)
摘要
Early decision making in commercial livestock systems is key to maximising animal welfare and production. Detailed information on an animal’s phenotype is needed to facilitate this, but can be difficult to obtain in a commercial setting. Research into the use of bio-logging on sheep to continuously monitor individual behaviour and indirectly inform health and production has seen rapid growth in recent years. Much of this research, however, has been conducted on small numbers of animals in an experimental setting and over limited time periods. Previous studies have also focused on ewes and there has been little research on the potential of bio-logging for collecting behavioural data on lambs, despite clear potential relevance for production. The present study aimed to test the feasibility of deploying accelerometers on a commercial sheep flock at a key point in the annual production cycle (lambing), to validate the viability of automated monitoring of sheep behaviour in a commercial setting. Also, we aimed to develop robust machine learning algorithms that can classify both the posture and physical activity of adult sheep and lambs. We used a Random Forest machine learning algorithm to predict: two mutually exclusive postures in ewes and lambs (standing and lying), achieving average accuracies of 83.7% and 85.9% respectively; four mutually exclusive activities in ewes (grazing, ruminating, inactive and walking), achieving an average accuracy of 70.9%; and three mutually exclusive activities in lambs (inactive, suckling, walking), achieving an average accuracy of 80.8%. These performance accuracies on large numbers of individuals afford the opportunity to provide a detailed understanding of the daily activity budget of ewes and lambs. Monitoring changes in daily patterns across the annual production cycle while capturing changes in environmental conditions such as weather, day length, terrain and management could reveal key indicator metrics that may inform production and health and provide early warning systems for key issues in commercial flocks.
更多查看译文
关键词
Sheep,Lambs,Accelerometer,Machine learning,Behaviour
AI 理解论文
溯源树
样例
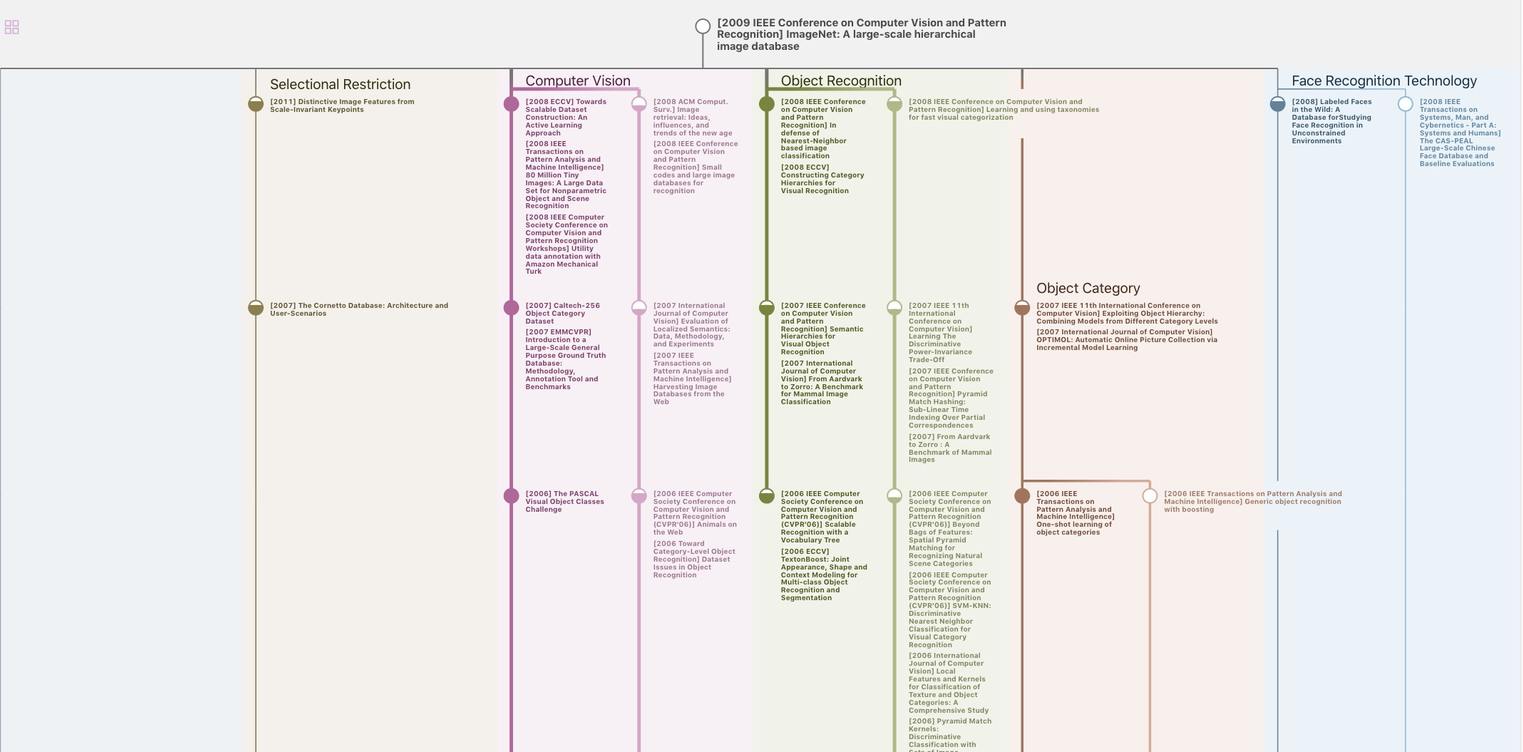
生成溯源树,研究论文发展脉络
Chat Paper
正在生成论文摘要