LTE-LAA cell selection through operator data learning and numerosity reduction
Pervasive and Mobile Computing(2022)
摘要
Long Term Evolution-Licensed Assisted Access (LTE-LAA) architecture is markedly different from traditional LTE HetNets. LTE-LAA deployments also have to contend with interference from coexisting Wi-Fi transmissions in the unlicensed spectrum. Hence, there is a need for innovative cell selection solutions that cater specifically to LTE-LAA. Further, the impact of cell selection on the performance of the existing LTE-LAA deployments should also be investigated through operator data analysis. This work addresses these challenges. We gather a large sample of LTE-LAA deployment data for three cellular operators, i.e., AT&T, T-Mobile, and Verizon, which is analyzed through several supervised machine learning algorithms. We study the effect of cell selection on LTE-LAA capacity and network feature relationships. Insightful inferences are drawn on the contrasting characteristics of the Licensed and Unlicensed components of an LTE-LAA system. Further, a cell-quality metric is derived from operator data and is shown to have a strong correlation with Unlicensed coexistence network performance. To validate the proposed ideas, two state-of-the-art cell association and resource allocation solutions are implemented. Validation results show that data-driven cell-selection can reduce Unlicensed association time by as much as 34.89%, and enhance Licensed network capacity by up to 90.41%. Finally, with the vision to reduce the computational overhead of data-driven cell selection in LAA and 5G New Radio Unlicensed networks, the performance of two popular numerosity reduction techniques is evaluated.
更多查看译文
关键词
Unlicensed networks,LTE-LAA,Coexistence networks,LTE-WiFi,Cell selection,Machine learning,Optimization,Operator data
AI 理解论文
溯源树
样例
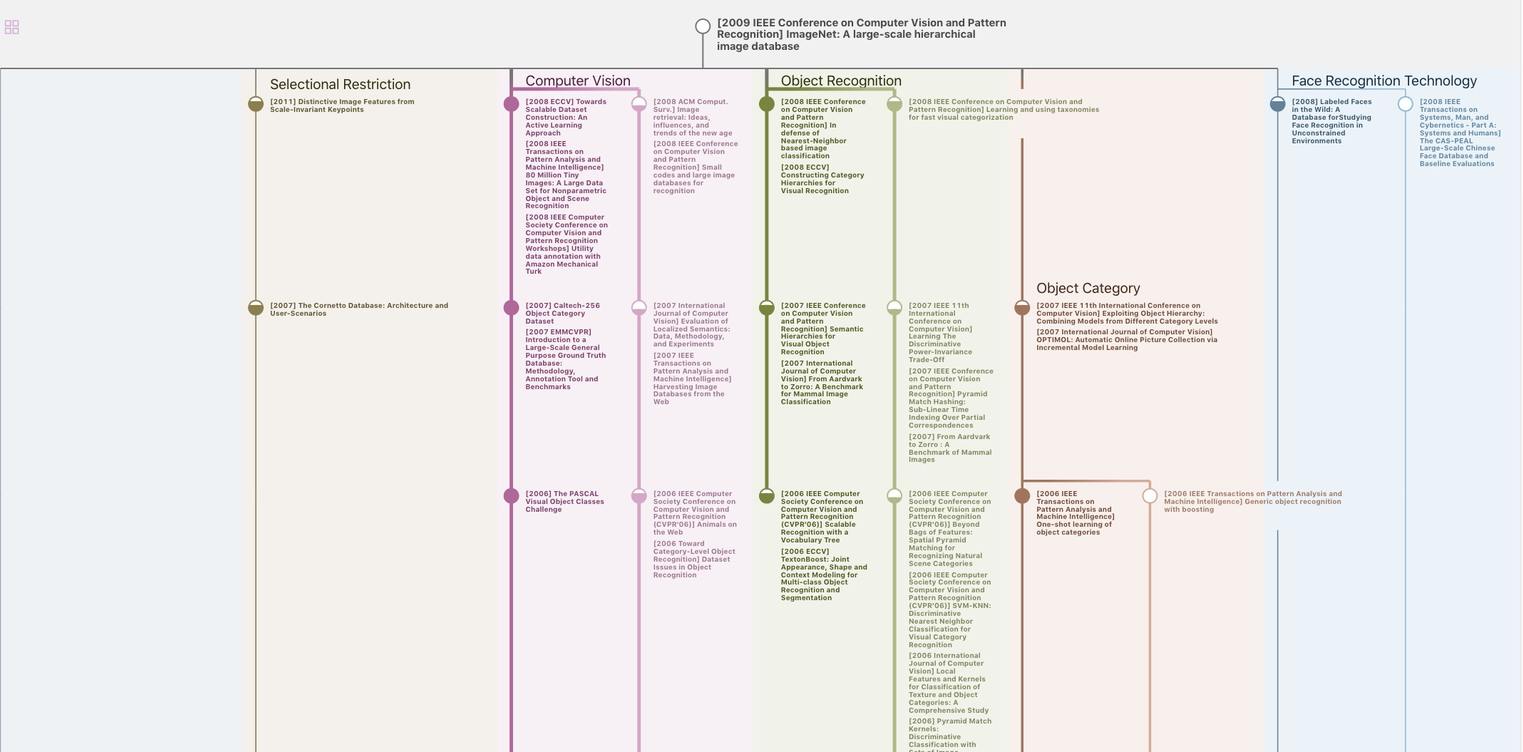
生成溯源树,研究论文发展脉络
Chat Paper
正在生成论文摘要