AHSS applications in Industry 4.0: Determination of optimum processing parameters during coiling process through unsupervised machine learning approach
Materials Today Communications(2022)
Abstract
In this work, actual production data from a rolling mill has been analyzed through the concept of Self Organizing Maps (SOM) for understanding various correlations and patterns in the production data set. SOM is an unsupervised machine learning approach, thus all the process parameters and desired properties can be analyzed simultaneously. Yield Strength (YS), Ultimate tensile strength (UTS) and elongation percent properties have been chosen as the metrics on the basis of which the coil can be utilized for a specific application. This production data contains many missing parameters. Additionally, parameters like different carbon equivalents (CE) and grain size of ferrite GS are dependent of other input parameters (composition of the coils, processing temperature, etc). Therefore, it is important to understand correlations between compositions, processing parameters, structure (GS), and macroscopic properties. Through SOM approach, several correlations have been determined in the production data set which are in accordance with the findings reported in the open literature. SOM predictions have been tabulated. These predictions will be helpful in achieving the optimum properties for various AHSS grades in the industry.
MoreTranslated text
Key words
Advanced high-strength steel (AHSS),Coiling,Ultimate tensile strength (UTS),Industry 4.0,Artificial intelligence,Unsupervised machine learning,Self-organizing maps (SOM),Processing-structure-property (PSP),Integrated computational materials engineering (ICME),Carbon equivalent
AI Read Science
Must-Reading Tree
Example
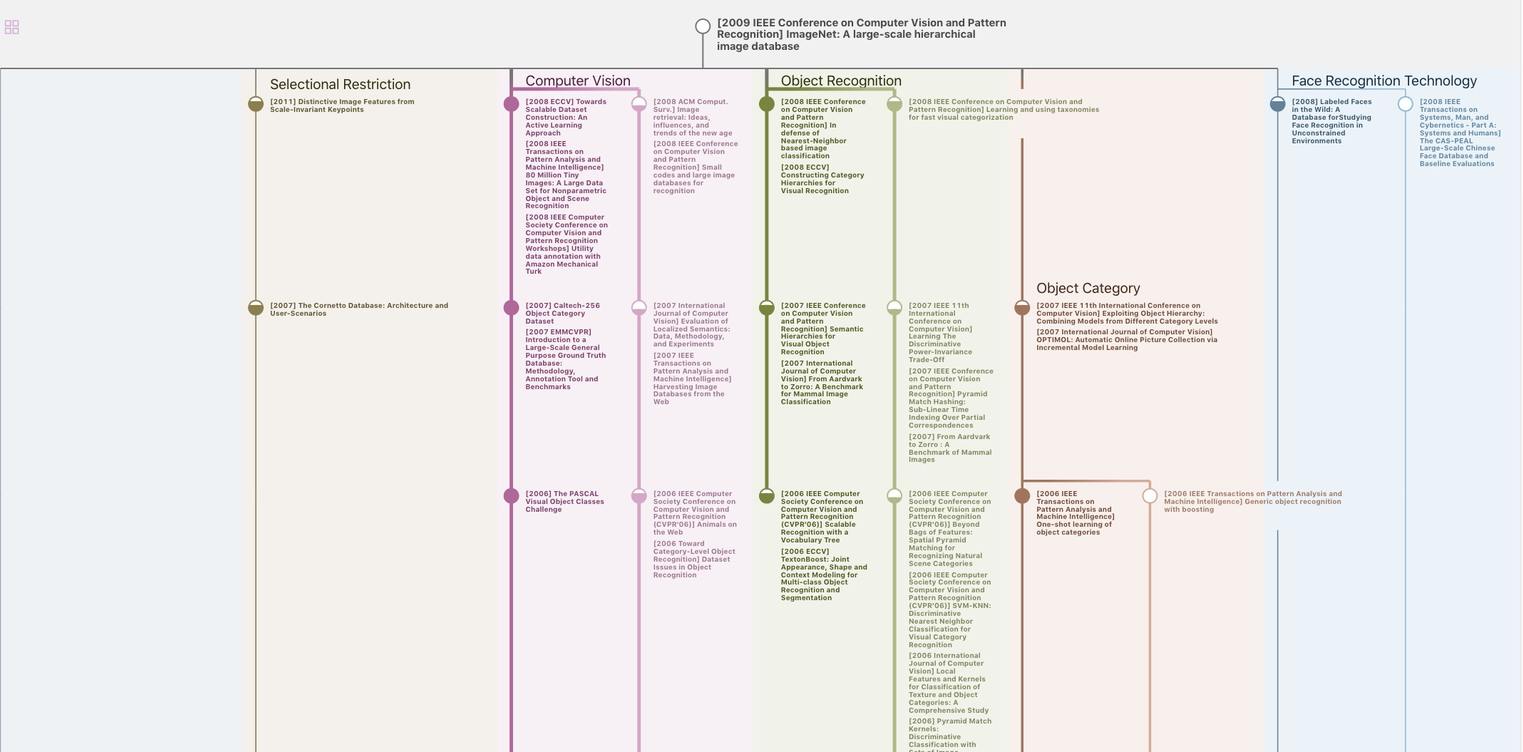
Generate MRT to find the research sequence of this paper
Chat Paper
Summary is being generated by the instructions you defined