Fair Transfer Learning with Factor Variational Auto-Encoder
NEURAL PROCESSING LETTERS(2022)
Abstract
Recently, in the field of fair machine learning, a large number of studies have considered how to remove discriminatory information from the data and achieve fairness in downstream tasks. Fair representation learning considers removing sensitive information (e.g. race, gender, etc) in the latent space, and the learned representations can prevent machine learning systems from being biased by discriminatory information. In this paper, we study the problems of existing methods and propose a novel fair representation learning method for the fair transfer learning where the labels of the downstream tasks are unknown. Specifically, we bring a new training model with information-theoretically motivated objective which avoids the problem of alignment for learning disentangled fair representations. Empirical results in various settings demonstrate the broad applicability and utility of our approach.
MoreTranslated text
Key words
Fair transfer learning, Disentanglement, Trustworthy machine learning
AI Read Science
Must-Reading Tree
Example
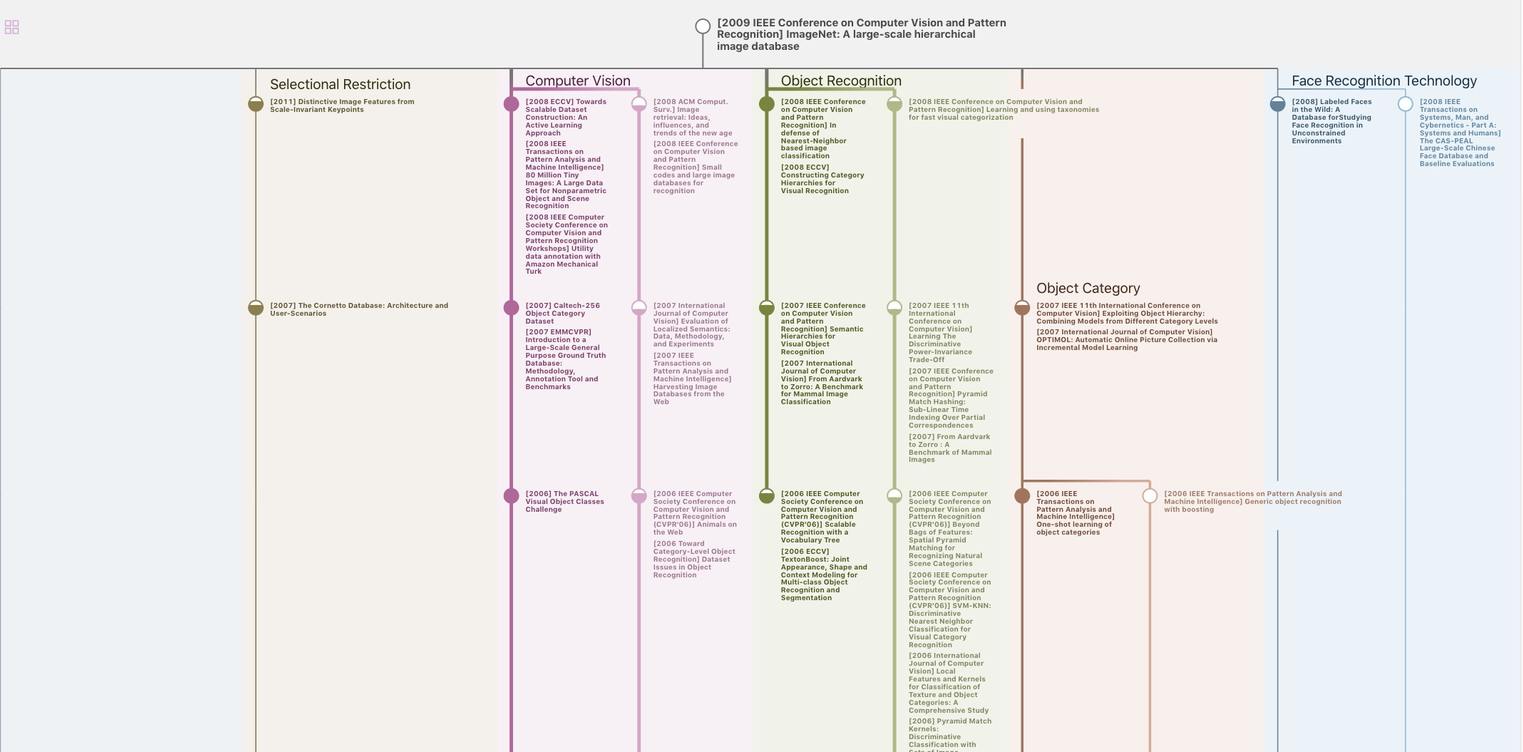
Generate MRT to find the research sequence of this paper
Chat Paper
Summary is being generated by the instructions you defined