Battery degradation prediction against uncertain future conditions with recurrent neural network enabled deep learning
Energy Storage Materials(2022)
摘要
Accurate degradation trajectory and future life are the key information of a new generation of intelligent battery and electrochemical energy storage systems. It is very challenging to obtain accurate predictions against uncertain application conditions by using only a few known historical data. In this article, we extend the widely studied remaining useful life (RUL) prediction to the prediction of charge and discharge capacity trajectories under both fixed and random future operating conditions. This is achieved by developing a general deep learning framework cored by recurrent neural network (RNN) which integrates future current plan and few early capacity-voltage data as inputs. As a case study, we have experimented with 77 commercial batteries cycled under fixed and random operating conditions. We demonstrate that the median root mean square error (RMSE) of prediction can be within 2.4% for NMC/graphite batteries and 2.3% for LFP/graphite batteries by using 3.8% of the whole life data only. Compared with the existing methods, the proposed framework predicts more accurately and has a very balanced performance for both fixed and random future conditions. This work highlights the promise of actively forecasting the future of batteries based on RNN.
更多查看译文
关键词
recurrent neural network,deep learning,battery,degradation,prediction
AI 理解论文
溯源树
样例
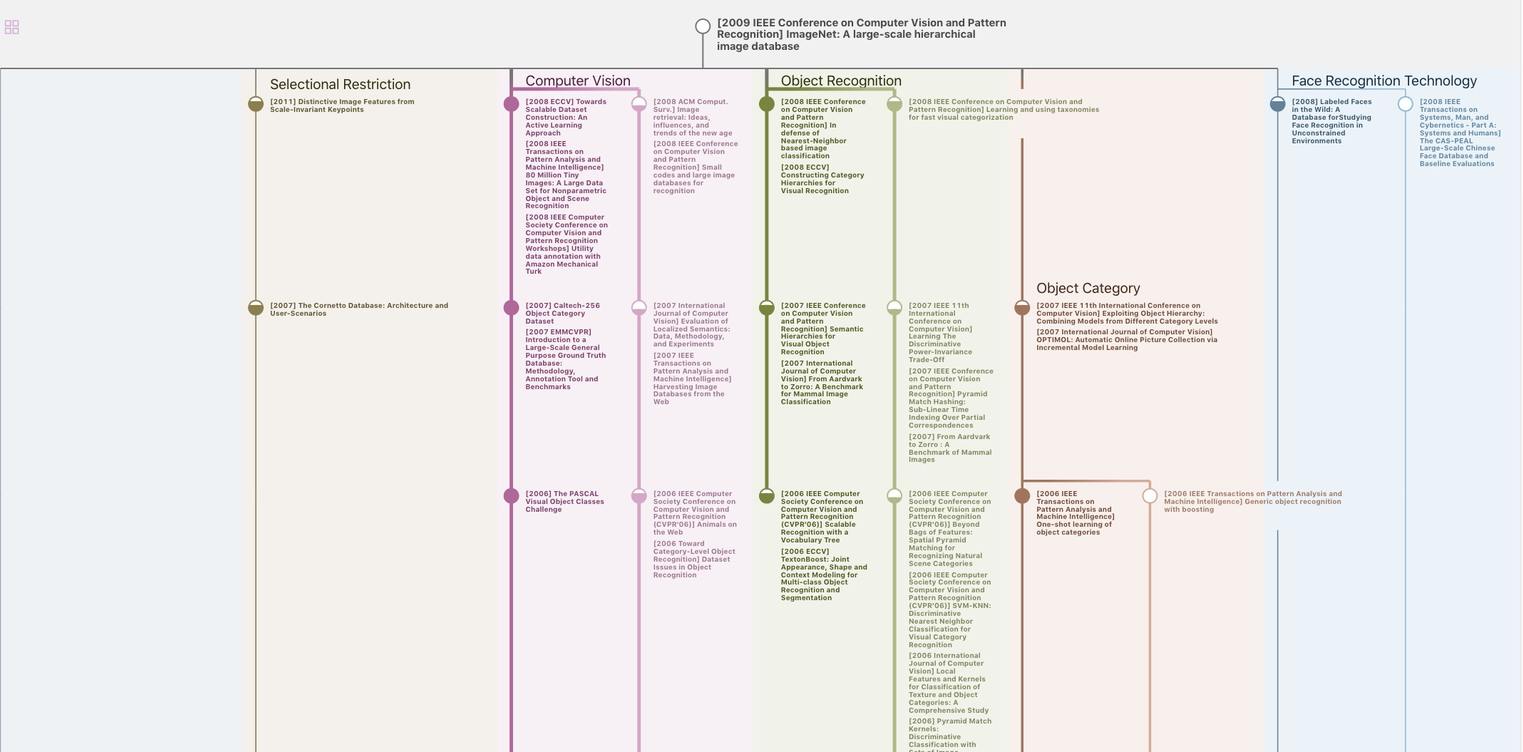
生成溯源树,研究论文发展脉络
Chat Paper
正在生成论文摘要