Low-Rank Approximations for Parametric Non-Symmetric Elliptic Problems
FRONTIERS IN PHYSICS(2022)
摘要
In this study, we obtained low-rank approximations for the solution of parametric non-symmetric elliptic partial differential equations. We proved the existence of optimal approximation subspaces that minimize the error between the solution and an approximation on this subspace, with respect to the mean parametric quadratic norm associated with any preset norm in the space of solutions. Using a low-rank tensorized decomposition, we built an expansion of approximating solutions with summands on finite-dimensional optimal subspaces and proved the strong convergence of the truncated expansion. For rank-one approximations, similar to the PGD expansion, we proved the linear convergence of the power iteration method to compute the modes of the series for data small enough. We presented some numerical results in good agreement with this theoretical analysis.
更多查看译文
关键词
low-rank tensor approximations,non-symmetric problems,PGD mode computation,alternate least squares,power iteration algorithm
AI 理解论文
溯源树
样例
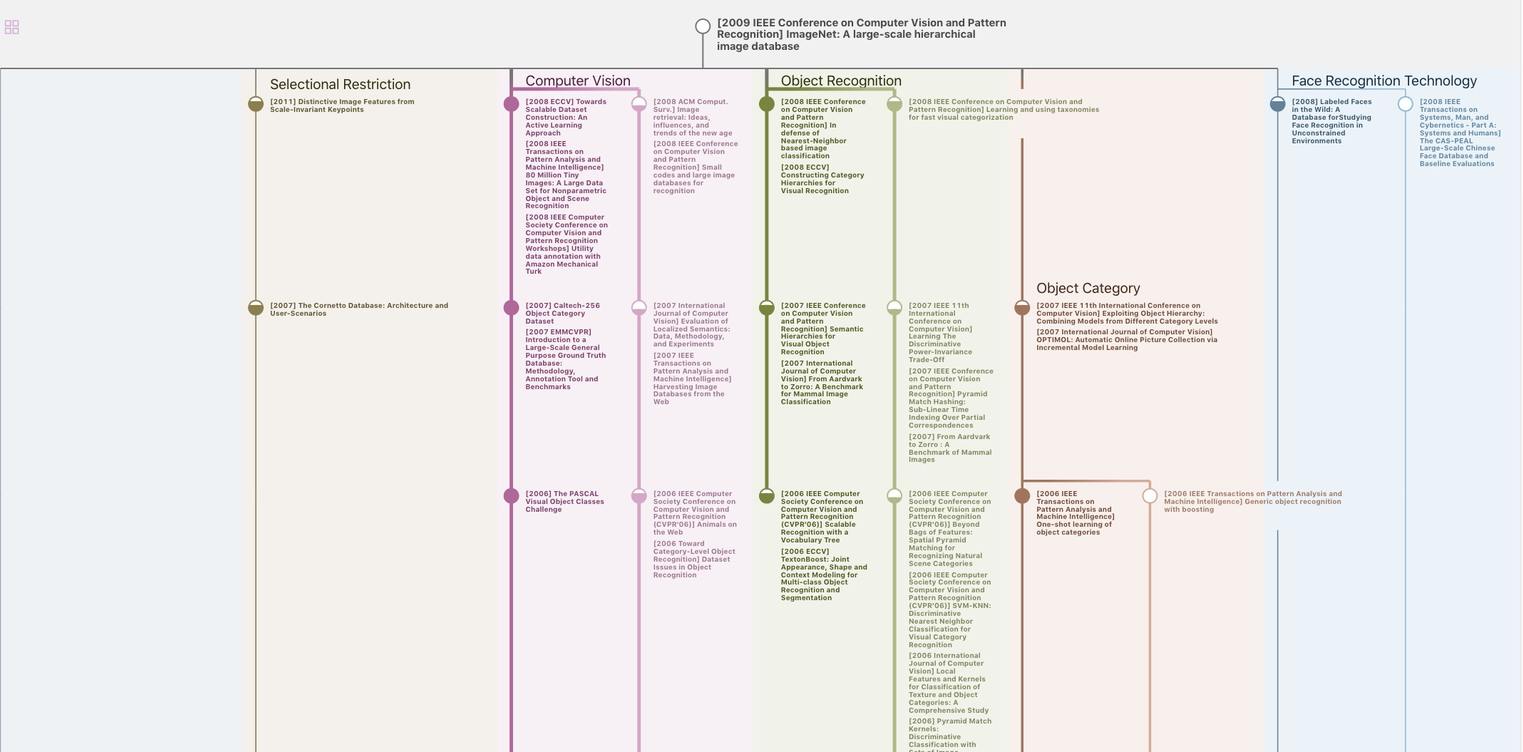
生成溯源树,研究论文发展脉络
Chat Paper
正在生成论文摘要