Batch Normalization and Dropout Regularization in Training Deep Neural Networks with Label Noise
INTELLIGENT SYSTEMS DESIGN AND APPLICATIONS, ISDA 2021(2022)
摘要
Availability of large annotated datasets in computer vision, speech understanding, or natural language processing is one of the main reasons for deep neural networks popularity. Unfortunately, such data can suffer from label noise, introduced by incorrectly labelled patterns. Since neural networks, as data-driven approaches, are strongly dependent on the quality of training data, the results of building deep neural structures with noisy examples can be unreliable. In this paper, we present preliminary experimental results on how two regularization techniques, namely dropout and batch normalization, influence vulnerability to incorrect labels. On popular MNIST and CIFAR-10 datasets we demonstrate that combination of these two approaches can be considered as a tool to improve network robustness to mislabelled training examples.
更多查看译文
关键词
Neural networks, Deep learning, Batch normalization, Dropout, Label noise
AI 理解论文
溯源树
样例
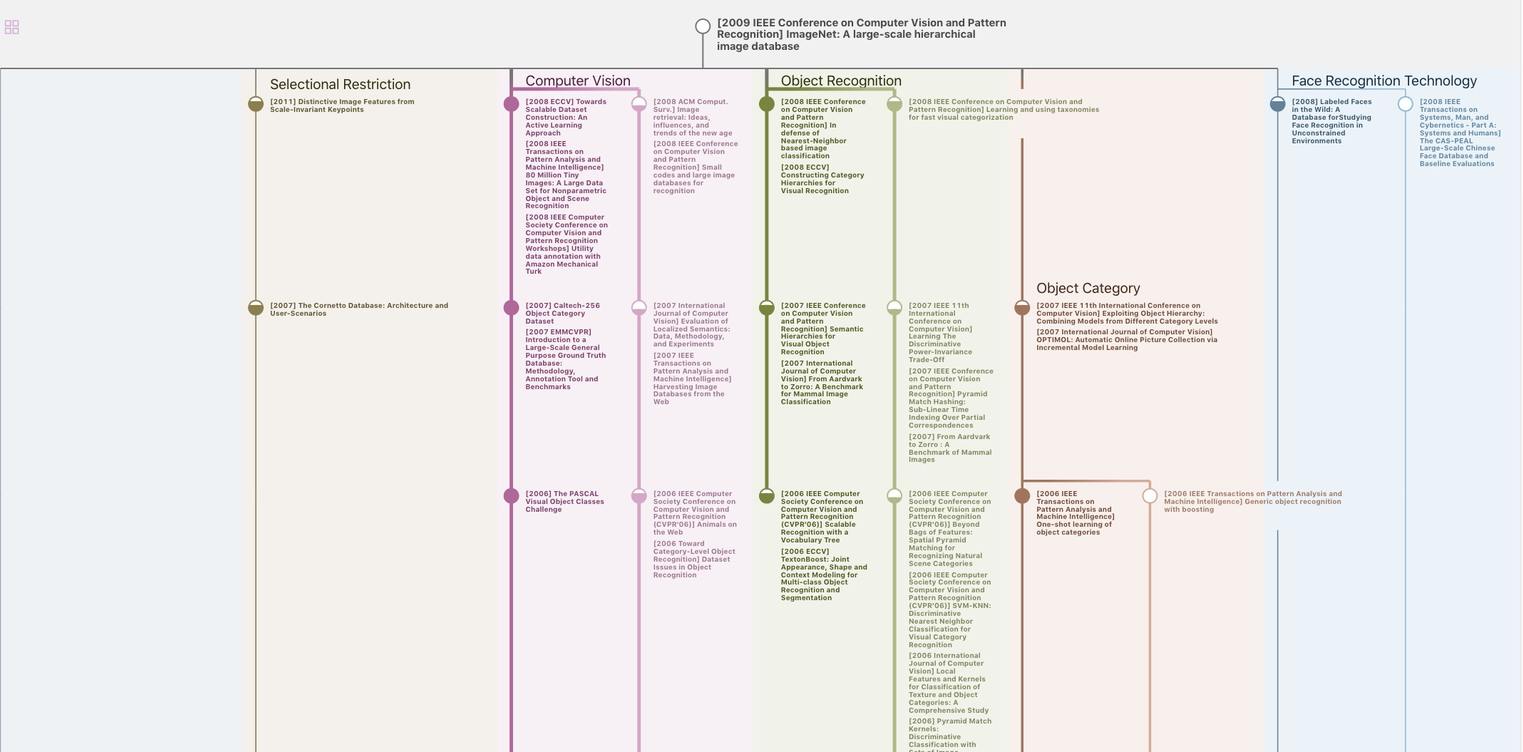
生成溯源树,研究论文发展脉络
Chat Paper
正在生成论文摘要