Using Machine Learning Approaches to Identify Exercise Activities from a Triple-Synchronous Biomedical Sensor
INTELLIGENT SYSTEMS DESIGN AND APPLICATIONS, ISDA 2021(2022)
摘要
Human activity recognition (HAR) is the method for identifying a person's activity using sensors that sense and capture movements during activity. HAR is an evolving area of research in computing, applied to sensors found in smartphones, smartwatches, and cameras. This paper illustrates how to identify some types of human activities using a BioStamp sensor that consists of an inertial sensors-based smart patch. Our dataset has five activities: stair climbing, jogging, skipping, kettlebell lifting, and passing a basketball. We employed both supervised and unsupervised learning approaches to perform HAR. For supervised learning, the dataset was divided into 67% training data and 33% testing data to train and evaluate the classification models. We evaluated the models on the accuracy, F1-score, precision, and recall. Random forest (F1-score = 97%) performed best followed by artificial neural networks (F1-score = 94%) and lastly, Gradient boosting (F1-score = 93%). We performed feature extraction on the original dataset and then performed unsupervised learning on the extracted features to obtain a Sorensen index value of 0.74, which shows good cluster separation. Geriatric or motor disease patients often use the BioStamp nPoint sensor and we demonstrate that their activities can be identified fairly accurately using machine learning approaches.
更多查看译文
关键词
Human activity recognition, Accelerometer, Gyroscope, Electrode values, Classification, Feature extraction
AI 理解论文
溯源树
样例
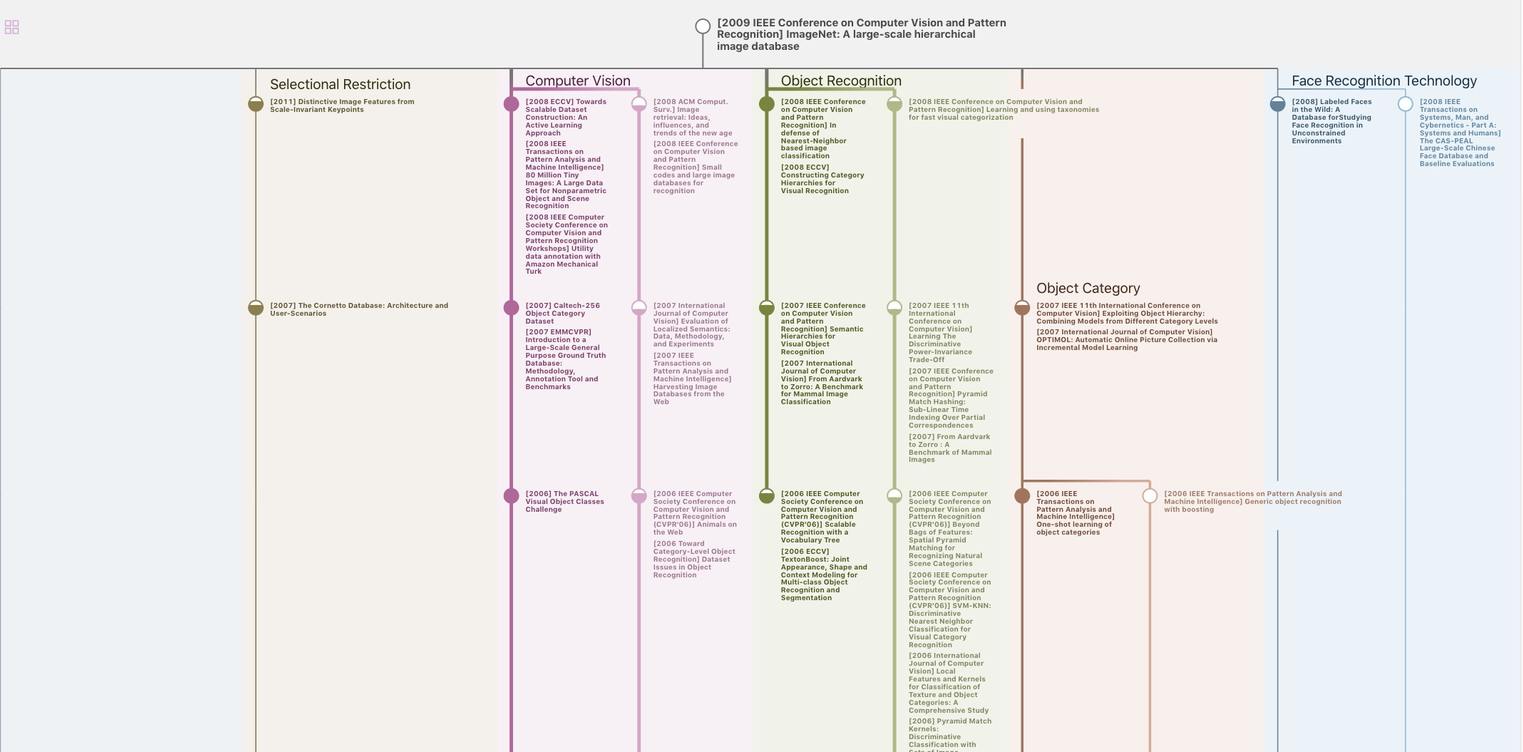
生成溯源树,研究论文发展脉络
Chat Paper
正在生成论文摘要