P-ROCK: A Sustainable Clustering Algorithm for Large Categorical Datasets
INTELLIGENT AUTOMATION AND SOFT COMPUTING(2023)
摘要
Data clustering is crucial when it comes to data processing and analytics. The new clustering method overcomes the challenge of evaluating and extracting data from big data. Numerical or categorical data can be grouped. Existing clustering methods favor numerical data clustering and ignore categorical data clustering. Until recently, the only way to cluster categorical data was to convert it to a numeric representation and then cluster it using current numeric clustering methods. However, these algorithms could not use the concept of categorical data for clustering. Following that, suggestions for expanding traditional categorical data processing methods were made. In addition to expansions, several new clustering methods and extensions have been proposed in recent years. ROCK is an adaptable and straightforward algorithm for calculating the similarity between data sets to cluster them. This paper aims to modify the algorithm by creating a parameterized version that takes specific algorithm parameters as input and outputs satisfactory cluster structures. The parameterized ROCK algorithm is the name given to the modified algorithm (P-ROCK). The proposed modification makes the original algorithm more flexible by using user-defined parameters. A detailed hypothesis was developed later validated with experimental results on real-world datasets using our proposed P-ROCK algorithm. A comparison with the original ROCK algorithm is also provided. Experiment results show that the proposed algorithm is on par with the original ROCK algorithm with an accuracy of 97.9%. The proposed P-ROCK algorithm has improved the runtime and is more flexible and scalable.
更多查看译文
关键词
ROCK, K-means algorithm, clustering approaches, unsupervised learning, K-histogram
AI 理解论文
溯源树
样例
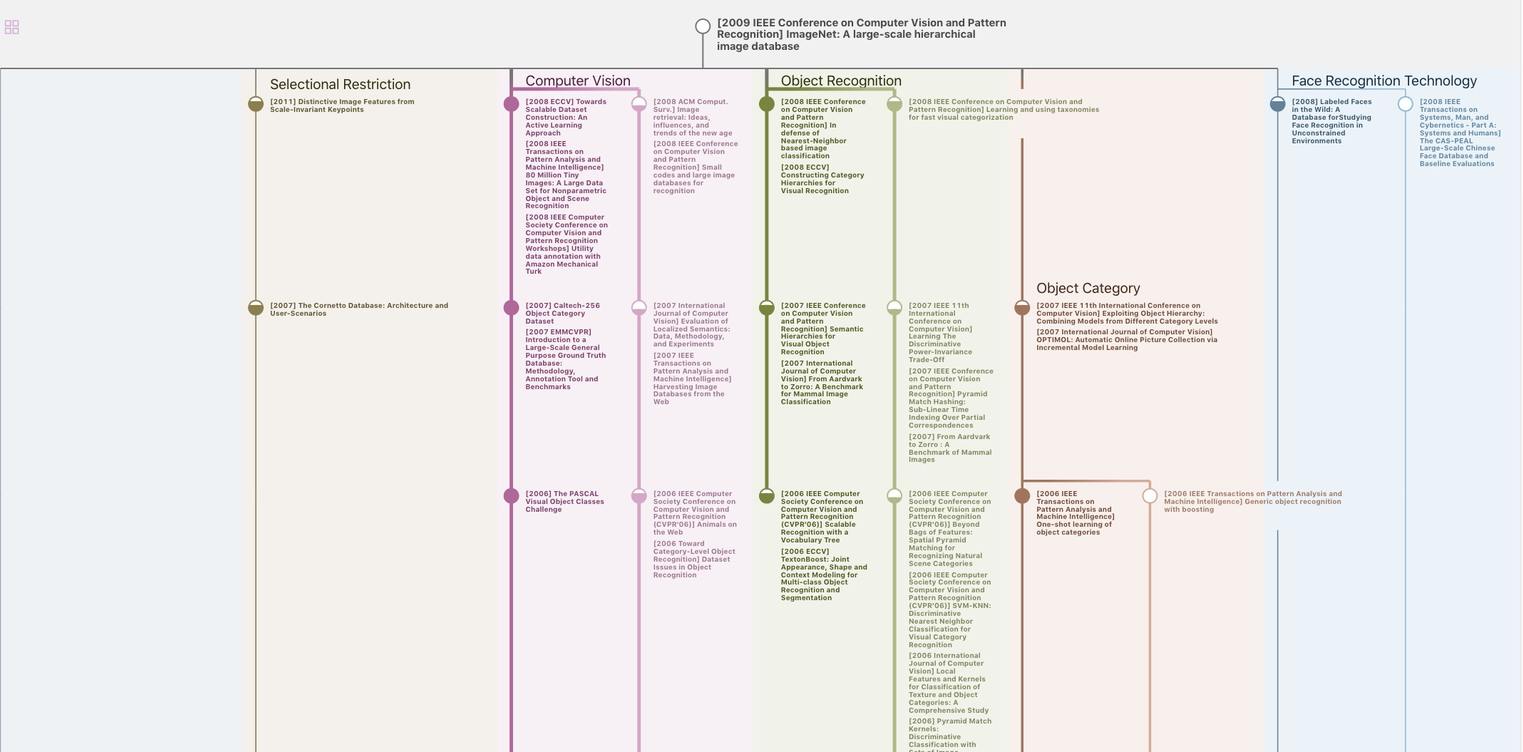
生成溯源树,研究论文发展脉络
Chat Paper
正在生成论文摘要