Feature Fusion Based Adversarial Example Detection Against Second-Round Adversarial Attacks
IEEE Transactions on Artificial Intelligence(2023)
Abstract
Convolutional neural networks (CNNs) achieve remarkable performances in various areas. However, adversarial examples threaten their security. They are designed to mislead CNNs to output incorrect results. Many methods are proposed to detect adversarial examples. Unfortunately, most detection-based defense methods are vulnerable to second-round adversarial attacks, which can simultaneously deceive the base model and the detector. To resist such second-round adversarial attacks, handcrafted steganalysis features are introduced to detect adversarial examples, while such a method receives low accuracy at detecting sparse perturbations. In this article, we propose to combine handcrafted features with deep features via a fusion scheme to increase the detection accuracy and defend against second-round adversarial attacks. To avoid deep features being overwhelmed by high-dimensional handcrafted features, we propose an expansion-then-reduction process to compress the dimensionality of handcrafted features. Experimental results show that the proposed model outperforms the state-of-the-art adversarial example detection methods and remains robust under various second-round adversarial attacks.
MoreTranslated text
Key words
Adversarial examples,detection,information hiding,second-round adversarial attacks,steganalysis
AI Read Science
Must-Reading Tree
Example
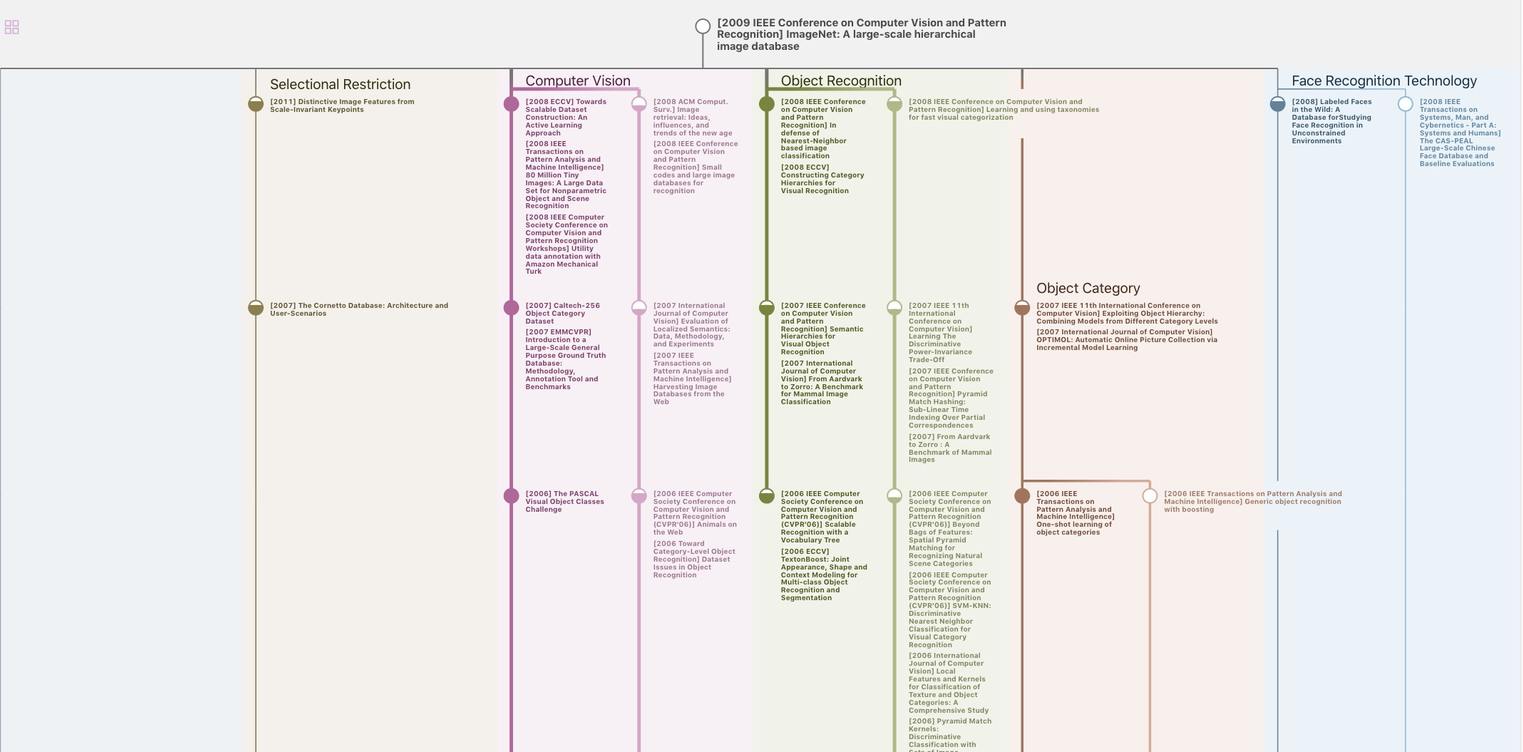
Generate MRT to find the research sequence of this paper
Chat Paper
Summary is being generated by the instructions you defined