A Self-Supervised Deep Learning Method for Seismic Data Deblending Using a Blind-Trace Network
IEEE transactions on neural networks and learning systems(2023)
Abstract
The simultaneous-source technology for high-density seismic acquisition is a key solution to efficient seismic surveying. It is a cost-effective method when blended subsurface responses are recorded within a short time interval using multiple seismic sources. A following deblending process, however, is needed to separate signals contributed by individual sources. Recent advances in deep learning and its data-driven approach toward feature engineering have led to many new applications for a variety of seismic processing problems. It is still a challenge, though, to collect enough labeled data and avoid model overfitting and poor generalization performance over different datasets with a low resemblance from each other. In this article, we propose a novel self-supervised learning method to solve the deblending problem without labeled training datasets. Using a blind-trace deep neural network and a carefully crafted blending loss function, we demonstrate that the individual source-response pairs can be accurately separated under three different blended-acquisition designs.
MoreTranslated text
Key words
Deep learning,Receivers,Noise reduction,Convolutional neural networks,Arrays,Training,Signal to noise ratio,Seismic data deblending,seismic data denoising,seismic data processing,self-supervised learning
AI Read Science
Must-Reading Tree
Example
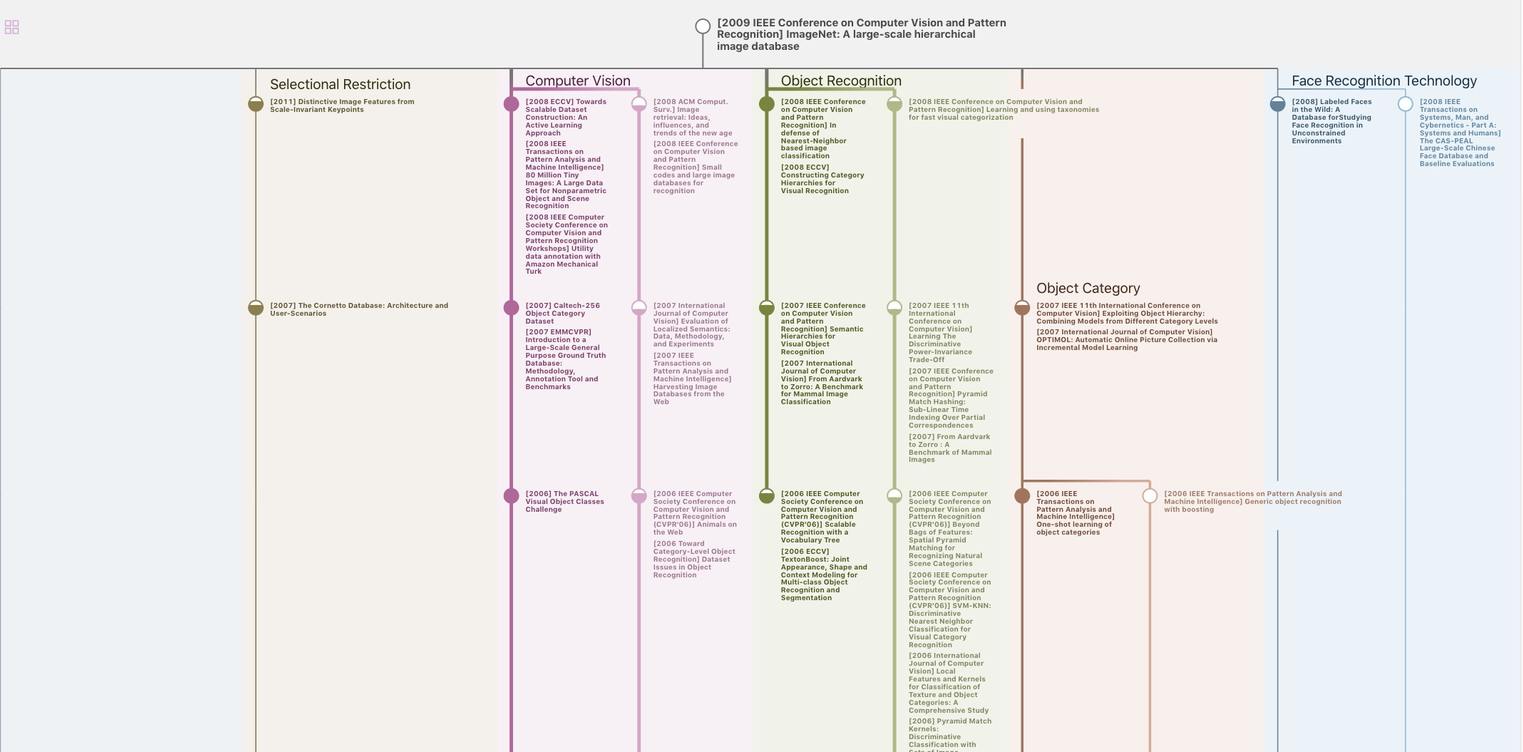
Generate MRT to find the research sequence of this paper
Chat Paper
Summary is being generated by the instructions you defined