Analysis of Catastrophic Forgetting for Random Orthogonal Transformation Tasks in the Overparameterized Regime
CoRR(2022)
摘要
Overparameterization is known to permit strong generalization performance in neural networks. In this work, we provide an initial theoretical analysis of its effect on catastrophic forgetting in a continual learning setup. We show experimentally that in permuted MNIST image classification tasks, the generalization performance of multilayer perceptrons trained by vanilla stochastic gradient descent can be improved by overparameterization, and the extent of the performance increase achieved by overparameterization is comparable to that of state-of-the-art continual learning algorithms. We provide a theoretical explanation of this effect by studying a qualitatively similar two-task linear regression problem, where each task is related by a random orthogonal transformation. We show that when a model is trained on the two tasks in sequence without any additional regularization, the risk gain on the first task is small if the model is sufficiently overparameterized.
更多查看译文
关键词
catastrophic forgetting,random orthogonal transformation
AI 理解论文
溯源树
样例
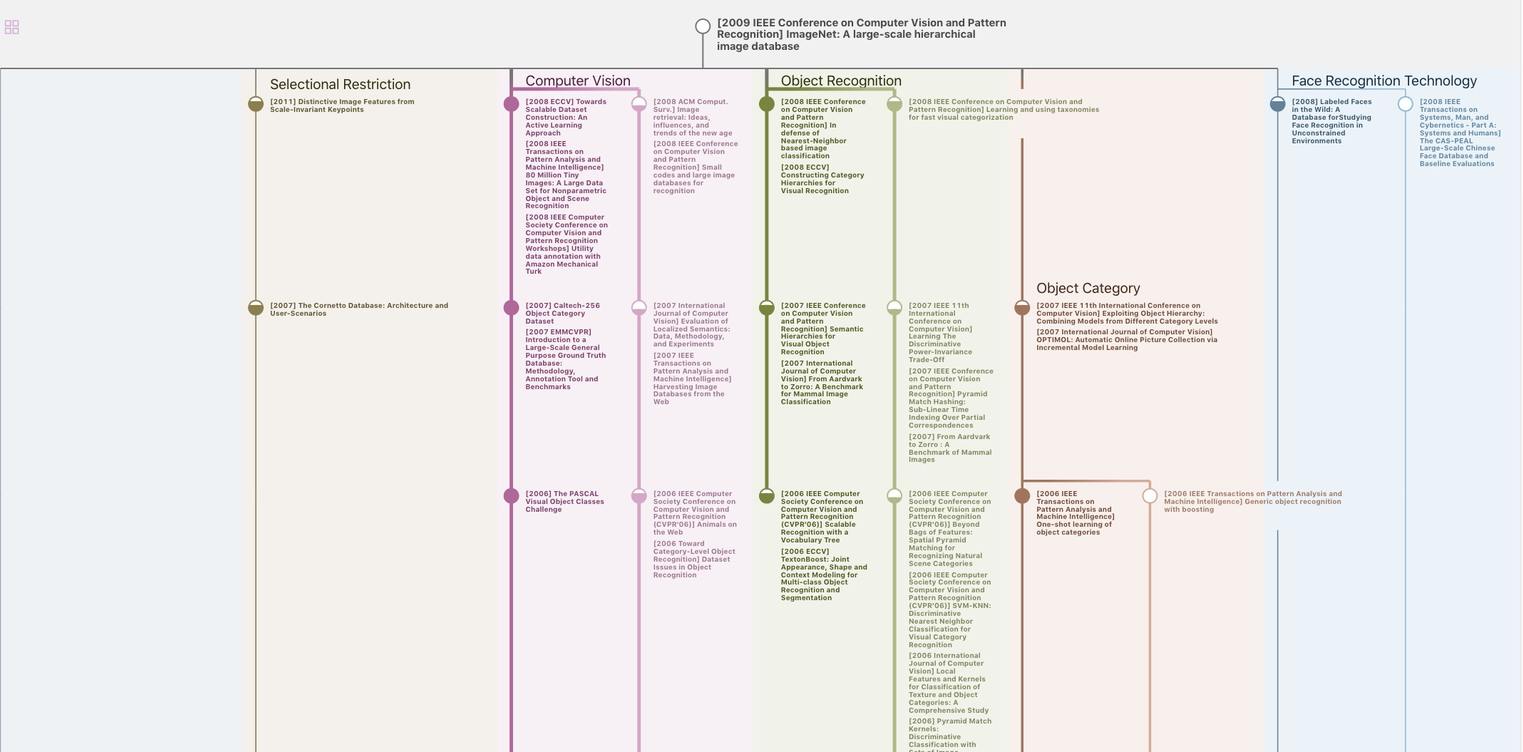
生成溯源树,研究论文发展脉络
Chat Paper
正在生成论文摘要