Cost-Effective Online Contextual Model Selection
arxiv(2023)
摘要
How can we collect the most useful labels to learn a model selection policy, when presented with arbitrary heterogeneous data streams? In this paper, we formulate this task as an online contextual active model selection problem, where at each round the learner receives an unlabeled data point along with a context. The goal is to output the best model for any given context without obtaining an excessive amount of labels. In particular, we focus on the task of selecting pre-trained classifiers, and propose a contextual active model selection algorithm (CAMS), which relies on a novel uncertainty sampling query criterion defined on a given policy class for adaptive model selection. In comparison to prior art, our algorithm does not assume a globally optimal model. We provide rigorous theoretical analysis for the regret and query complexity under both adversarial and stochastic settings. Our experiments on several benchmark classification datasets demonstrate the algorithm's effectiveness in terms of both regret and query complexity. Notably, to achieve the same accuracy, CAMS incurs less than 10% of the label cost when compared to the best online model selection baselines on CIFAR10.
更多查看译文
关键词
selection,model,cost-effective
AI 理解论文
溯源树
样例
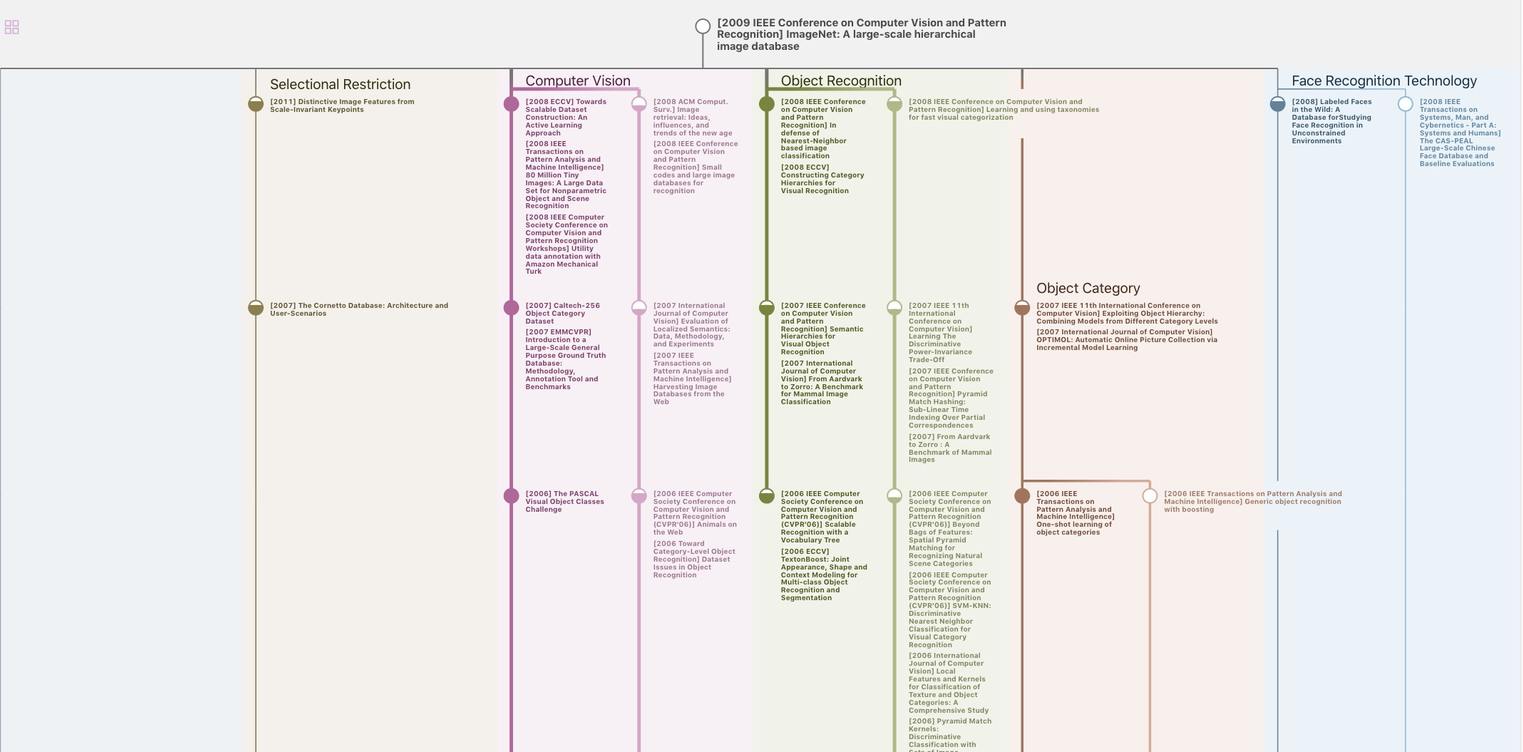
生成溯源树,研究论文发展脉络
Chat Paper
正在生成论文摘要