Image Super-Resolution Using a Simple Transformer Without Pretraining
Neural Processing Letters(2022)
摘要
Vision Transformer (ViT) has attracted tremendous attention and achieved remarkable success on high-level visual tasks. However, ViT relies on costly pre-training on large external datasets and is strict in data and calculations, making it an obstacle to running on common equipment. To address this challenge, we propose a simple and efficient Transformer namely SRT tailored for the image super-resolution (SR) reconstruction task. It is trained on a single GPU card without large-scale pre-training. At the beginning of the whole model, we introduce a convolutional stem module instead of straightforward tokenization of raw input images for low-level feature extraction and steady training. In the main Transformer learning phase, we exploit an additional head-convolution to make up for the lack of information interaction in multi-head self-attention (MHSA). Then further to strengthen the spatial correlation of neighboring tokens in MLP, a locally-enhanced feed-forward layer is thus employed to promote local dependencies. In terms of the inefficiency of Transformer, a channel reduction strategy is presented in MHSA, which dramatically reduces the complexity and space resources. Experimental results demonstrate the proposed Transformer model can rival the current state-of-the-art methods with a single GPU card.
更多查看译文
关键词
Super-resolution,Transformer,Convolutional neural networks
AI 理解论文
溯源树
样例
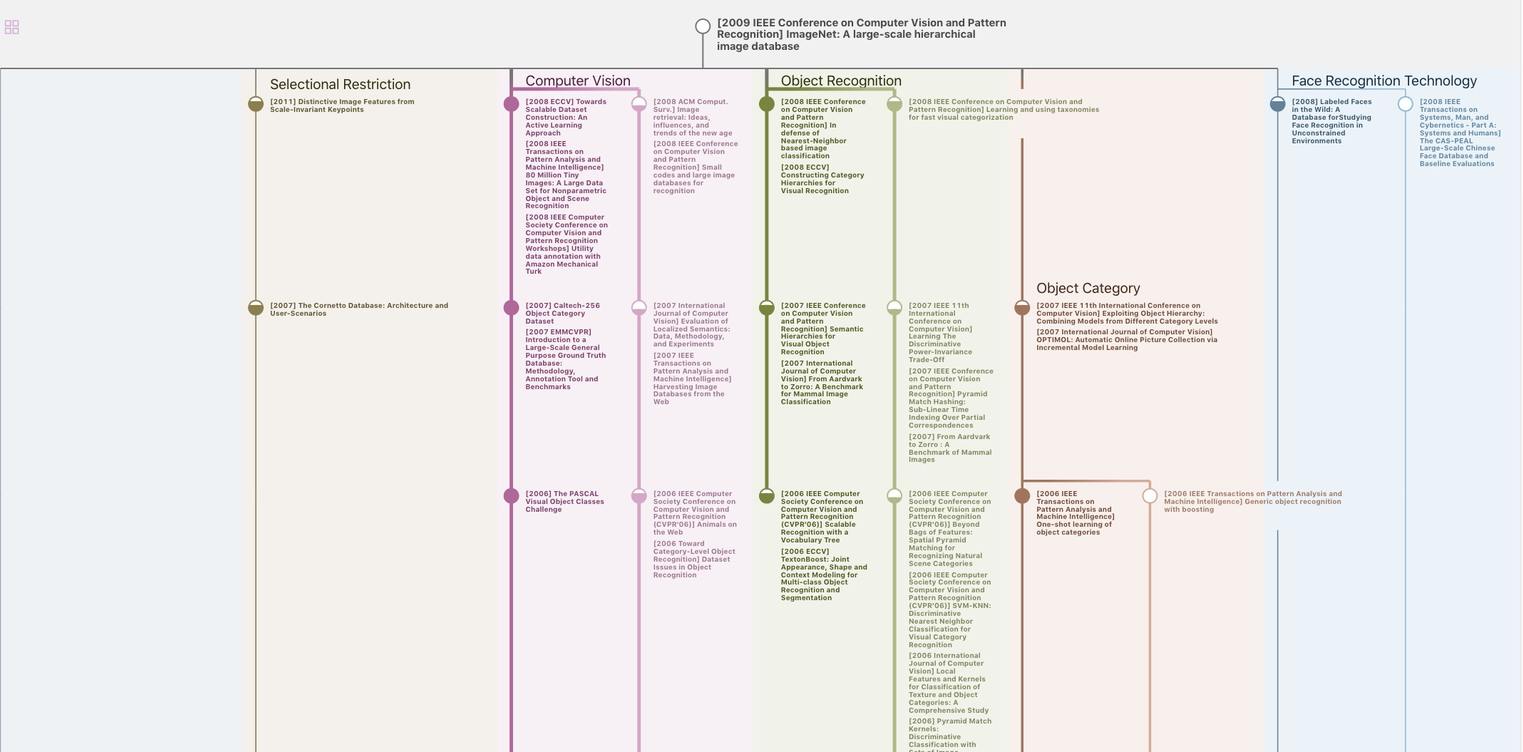
生成溯源树,研究论文发展脉络
Chat Paper
正在生成论文摘要