Model-Predictive Control Design for Power System Oscillation Damping via Excitation – A Data-Driven Approach
IEEE Transactions on Power Systems(2023)
摘要
This paper presents a novel power system oscillation damping controller design based on a data-driven model-predictive control (MPC) approach. The system dynamics are extracted from the measurements and applied to the synthesis of control actions in an equation-free manner, without requiring explicit knowledge of the underlying system model. Dynamic mode decomposition with control (DMDc), originating from the Koopman operator theory, connects the collected data with the nonlinear dynamical system modeling. The discrepancy between the model identified by DMDc and the real plant is labeled as a total disturbance. It is estimated in real time by an extended state observer (ESO) and mitigated via a linear MPC, assuming the estimate to be constant over the prediction horizon. The overall control framework is referred to as
ESO-Koopman-MPC (EKM)
. Two
EKM
-based oscillation damping controllers are proposed - a standalone PSS and an integrated AVR+PSS. The controller operation is illustrated on the single machine infinite bus system (SMIB), Kundur two-area system and IEEE 39-bus system, and the performance is compared with conventional power system stabilizers (CPSSs) under various test scenarios.
更多查看译文
关键词
Data-driven analysis and control,dynamic mode decomposition,extended state observer,model-predictive control,power system oscillation damping
AI 理解论文
溯源树
样例
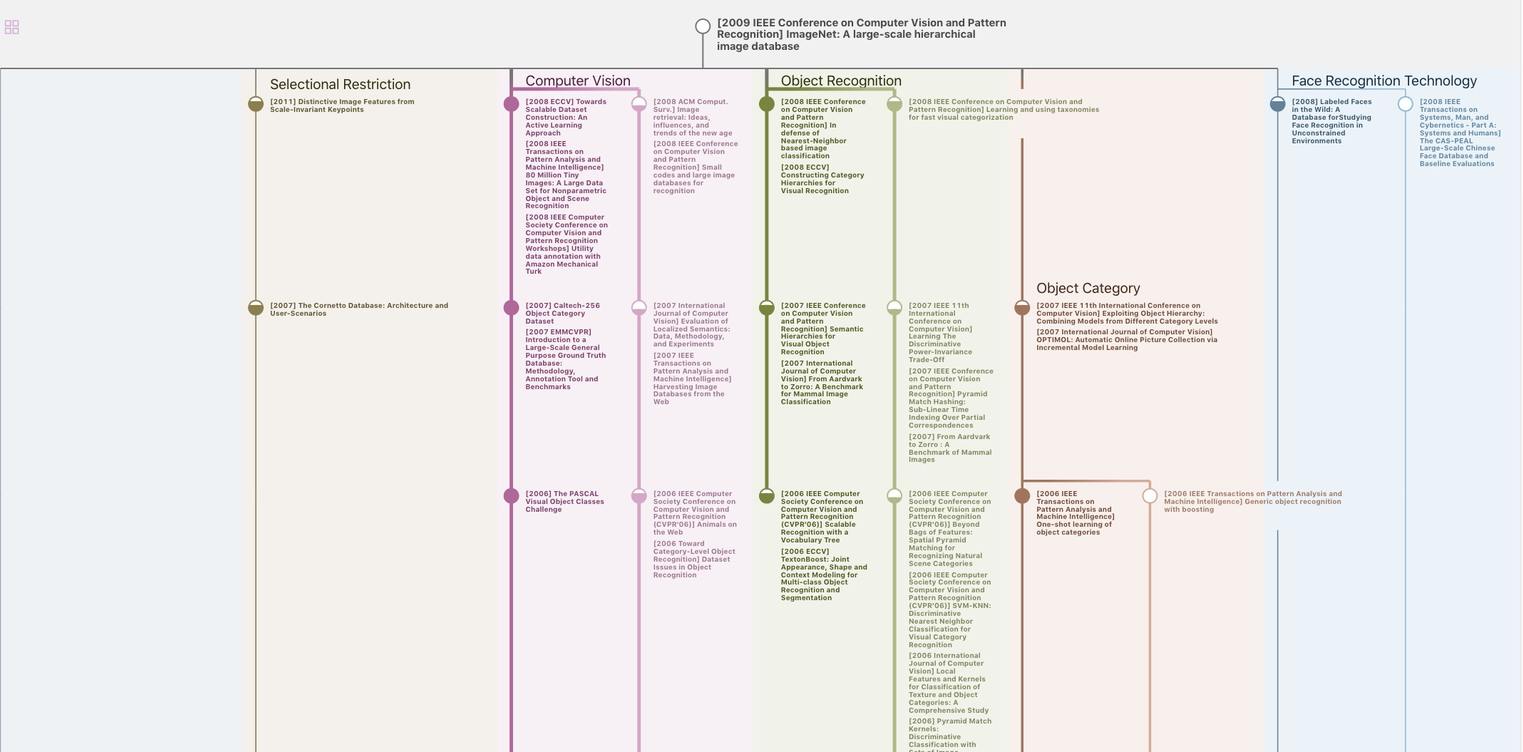
生成溯源树,研究论文发展脉络
Chat Paper
正在生成论文摘要