Utilizing community level factors to improve prediction of out of hospital cardiac arrest outcome using machine learning
Resuscitation(2022)
摘要
OBJECTIVES:To evaluate the impact of community level information on the predictability of out-of-hospital cardiac arrest (OHCA) survival.
METHODS:We used the Cardiac Arrest Registry to Enhance Survival (CARES) to geocode 9,595 Chicago incidents from 2014 to 2019 into community areas. Community variables including crime, healthcare, and economic factors from public data were merged with CARES. The merged data were used to develop ML models for OHCA survival. Models were evaluated using Area Under the Receiver Operating Characteristic curve (AUROC) and features were analyzed using SHapley Additive exPansion (SHAP) values.
RESULTS:Baseline results using CARES data achieved an AUROC of 84%. The final model utilizing community variables increased the AUROC to 88%. A SHAP analysis between high and low performing community area clusters showed the high performing cluster is positively impacted by good health related features and good community safety features positively impact the low performing cluster.
CONCLUSION:Utilizing community variables helps predict neurologic outcomes with better performance than only CARES data. Future studies will use this model to perform simulations to identify interventions to improve OHCA survival.
更多查看译文
关键词
Out of hospital cardiac arrest,Health disparities,Survival,Machine learning,Community
AI 理解论文
溯源树
样例
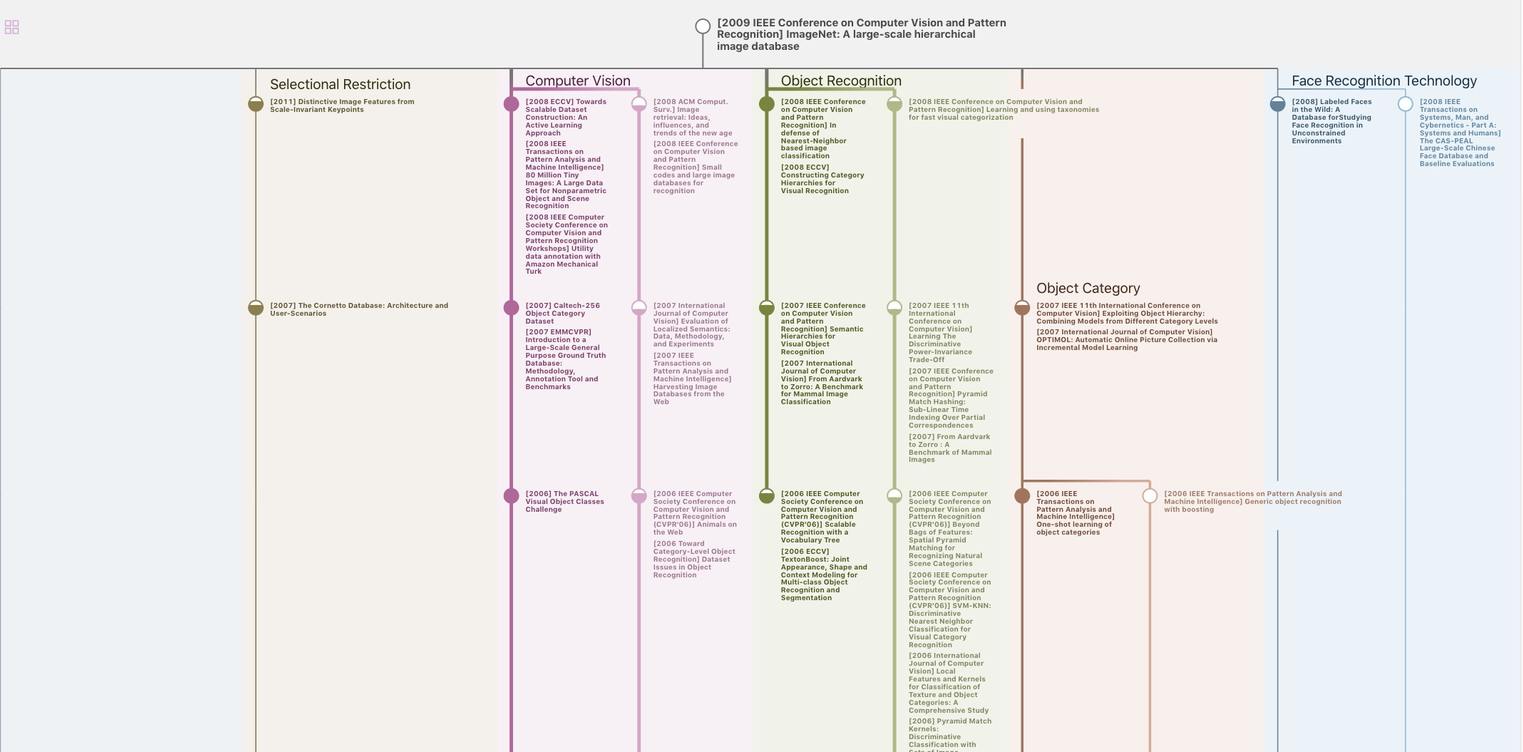
生成溯源树,研究论文发展脉络
Chat Paper
正在生成论文摘要