Skeletal Human Action Recognition using Hybrid Attention based Graph Convolutional Network.
ICPR(2022)
摘要
In skeleton-based action recognition, Graph Convolutional Networks model human skeletal joints as vertices and connect them through an adjacency matrix, which can be seen as a local attention mask. However, in most existing Graph Convolutional Networks, the local attention mask is defined based on natural connections of human skeleton joints and ignores the dynamic relations for example between head, hands and feet joints. In addition, the attention mechanism has been proven effective in Natural Language Processing and image description, which is rarely investigated in existing methods. In this work, we proposed a new adaptive spatial attention layer that extends local attention map to global based on relative distance and relative angle information. Moreover, we design a new initial graph adjacency matrix that connects head, hands and feet, which shows visible improvement in terms of action recognition accuracy. The proposed model is evaluated on two large-scale and challenging datasets in the field of human activities in daily life: NTU-RGB+D and Kinetics skeleton. The results demonstrate that our model has strong performance on both dataset.
更多查看译文
关键词
action recognition accuracy,adaptive spatial attention layer,attention mechanism,dynamic relations,existing Graph Convolutional Networks,feet,Graph Convolutional network,Graph Convolutional Networks model human skeletal joints,human activities,human skeleton joints,hybrid attention,initial graph adjacency matrix,local attention map,local attention mask,natural connections,Natural Language Processing,relative angle information,skeletal human action recognition,skeleton-based action recognition
AI 理解论文
溯源树
样例
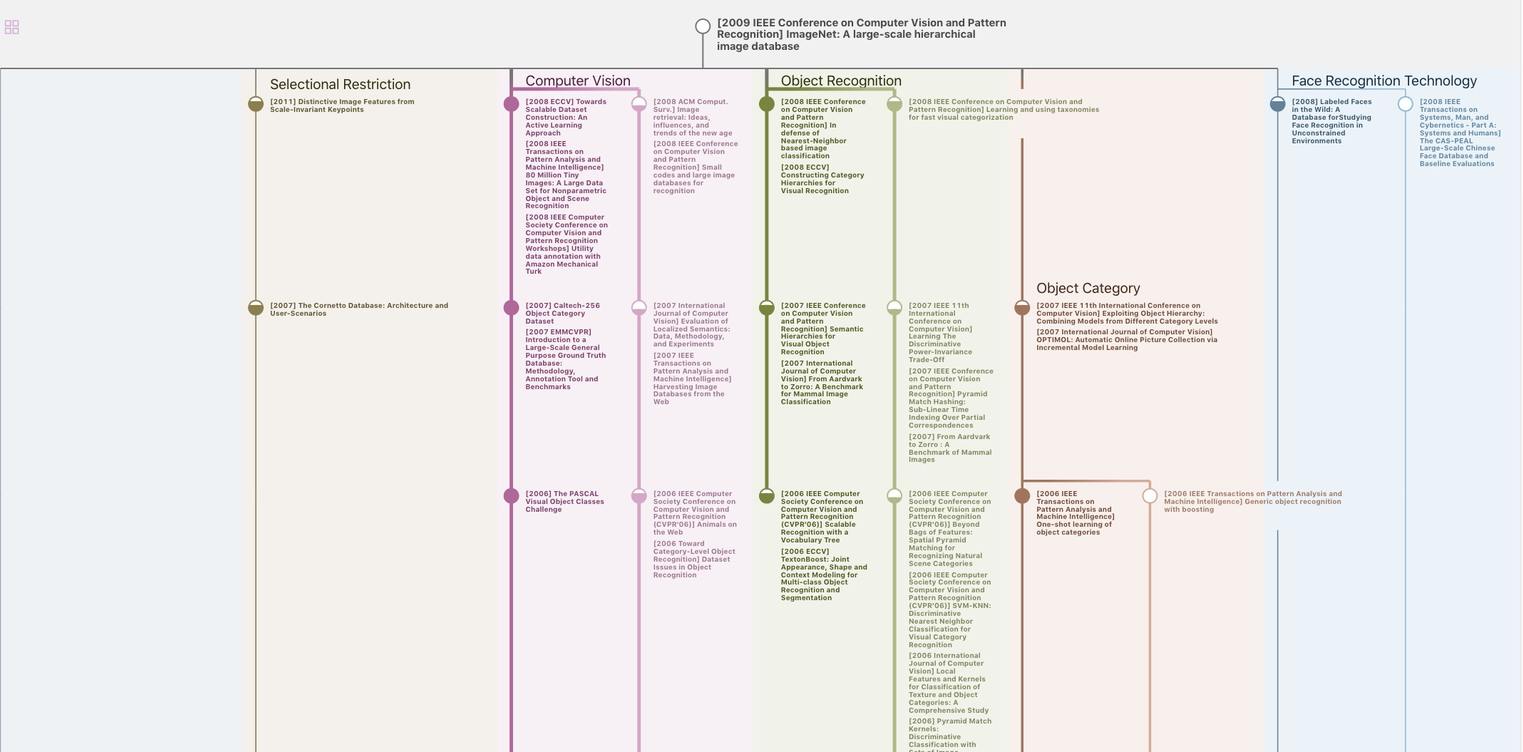
生成溯源树,研究论文发展脉络
Chat Paper
正在生成论文摘要