Modeling Working Memory Using Convolutional Neural Networks for Knowledge Tracing.
SSRN Electronic Journal(2023)
摘要
Recently, working memory models have been used in knowledge tracing to improve prediction performance, which is a cognitive system with limited processing capacity enabling short-term storage of information. However, existing versions do not model limited capacity adequately or consider individual differences in processing capacity. To resolve these problems, we simulate working memory for knowledge tracking (MCKT) by utilizing a Convolutional Neural Network (CNN). To this end, multiple CNNs with convolution kernels of different scales are used to process a single interaction sequence, where each scale of convolution kernels represents a certain processing capacity of the current learner. MCKT utilizes a multi-scale convolution kernel approach to achieve personalized simulation of processing capacities of different learners. Moreover, long-term memory is simulated to predict future learner responses. The results of experiments on several benchmark datasets from the real world reveals that MCKT performs better than multiple classical models.
更多查看译文
关键词
working memory,convolutional neural networks,knowledge,neural networks
AI 理解论文
溯源树
样例
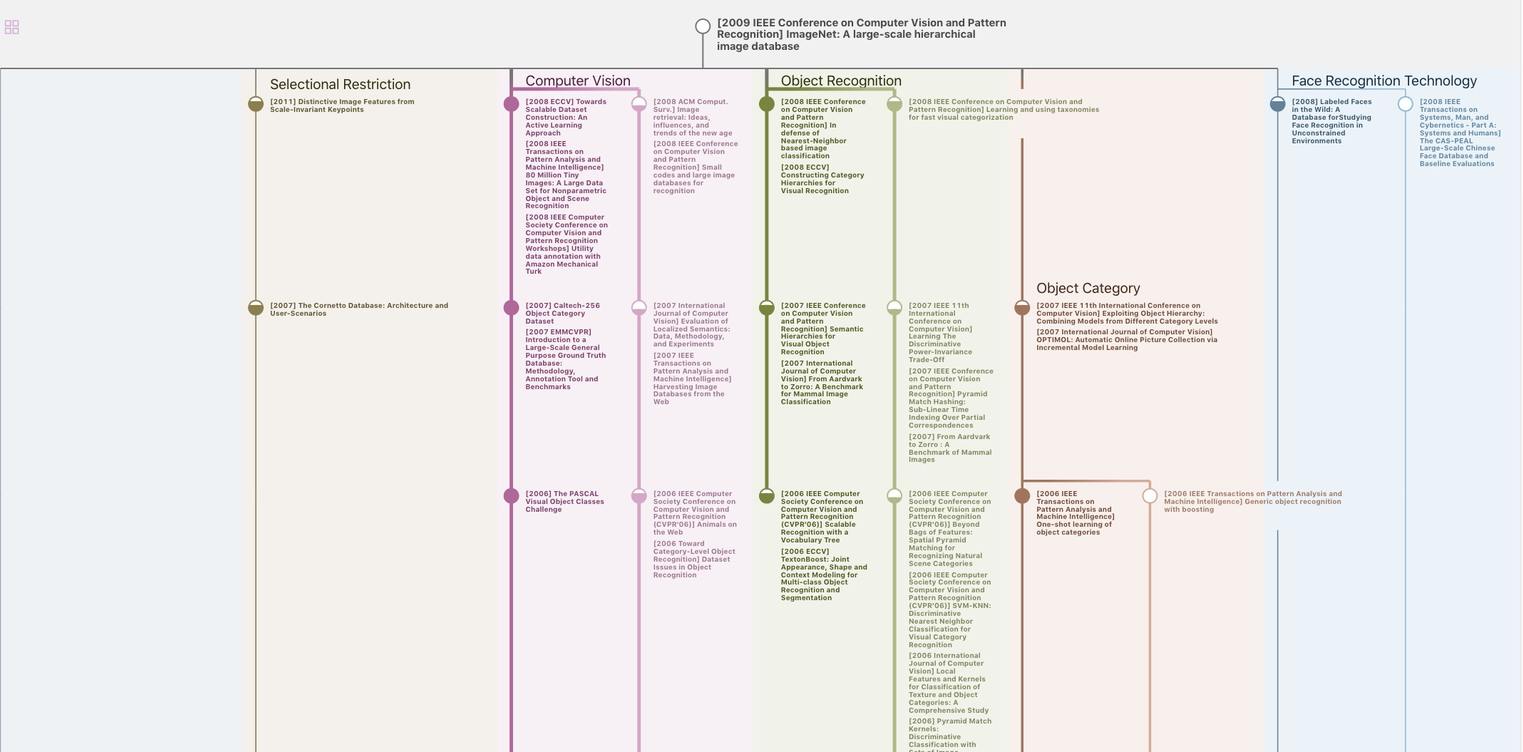
生成溯源树,研究论文发展脉络
Chat Paper
正在生成论文摘要