A dual-branch neural network for DeepFake video detection by detecting spatial and temporal inconsistencies
MULTIMEDIA TOOLS AND APPLICATIONS(2022)
摘要
It has become a research hotspot to detect whether a video is natural or DeepFake. However, almost all the existing works focus on detecting the inconsistency in either spatial or temporal. In this paper, a dual-branch (spatial branch and temporal branch) neural network is proposed to detect the inconsistency in both spatial and temporal for DeepFake video detection. The spatial branch aims at detecting spatial inconsistency by the effective EfficientNet model. The temporal branch focuses on temporal inconsistency detection by a new network model. The new temporal model considers optical flow as input, uses the EfficientNet to extract optical flow features, utilize the Bidirectional Long-Short Term Memory (Bi-LSTM) network to capture the temporal inconsistency of optical flow. Moreover, the optical flow frames are stacked before inputting into the EfficientNet. Finally, the softmax scores of two branches are combined with a binary-class linear SVM classifier. Experimental results on the compressed FaceForensics++ dataset and Celeb-DF dataset show that: (a) the proposed dual-branch network model performs better than some recent spatial and temporal models for the Celeb-DF dataset and all the four manipulation methods in FaceForensics++ dataset since these two branches can complement each other; (b) the use of optical flow inputs, Bi-LSTM and dual-branches can greatly improve the detection performance by the ablation experiments.
更多查看译文
关键词
DeepFake video detection,Optical flow,Convolution neural network,Long short-term memory network
AI 理解论文
溯源树
样例
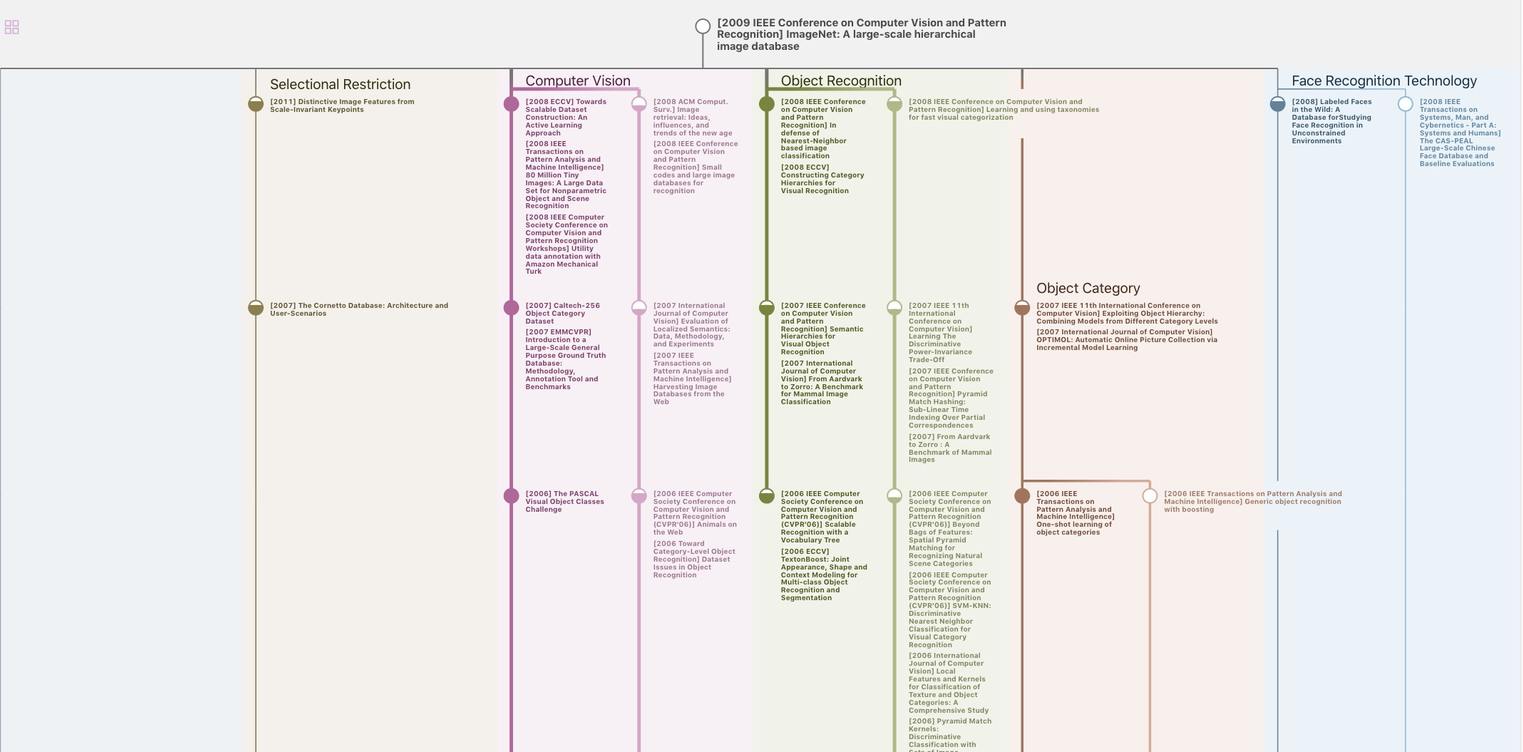
生成溯源树,研究论文发展脉络
Chat Paper
正在生成论文摘要