Coalbed Methane Concentration Prediction and Early-Warning in Fully Mechanized Mining Face Based on Deep Learning
ENERGY(2023)
Abstract
Coalbed methane (CBM) disasters are a major safety problem in coal mining. CBM concentration prediction and early-warning technology play a vital role in the prevention and control of CBM disasters. Traditional prediction methods have some shortcomings such as unreasonable data analysis, inability to predict in real time, long prediction time, and overfitting. This study uses a large amount of coal mine CBM data and develops a fast and high-precision CBM concentration prediction method based on deep learning theory, which can be used for CBM disaster early-warning. The proposed method has the following advantages: (1) it combines three exponential smoothing methods, the autoregressive model, wavelet domain denoising method, and principal component analysis. This method optimizes data with outliers, missing values, and noise. (2) Particle swarm optimization and genetic algorithm are used to optimize the network parameters of the gated recurrent unit. The application and verification of the optimized model show that the running time and accuracy are significantly improved. (3) By combining the optimized prediction model with Spark streaming, an early-warning system is developed, which can complete the efficient early-warning of CBM concentration within 8 s. The proposed method provides decision-making support for mine safety and CBM disaster prevention and control.
MoreTranslated text
Key words
Coalbed methane disaster,Deep learning,Gated recurrent unit,Prediction model
AI Read Science
Must-Reading Tree
Example
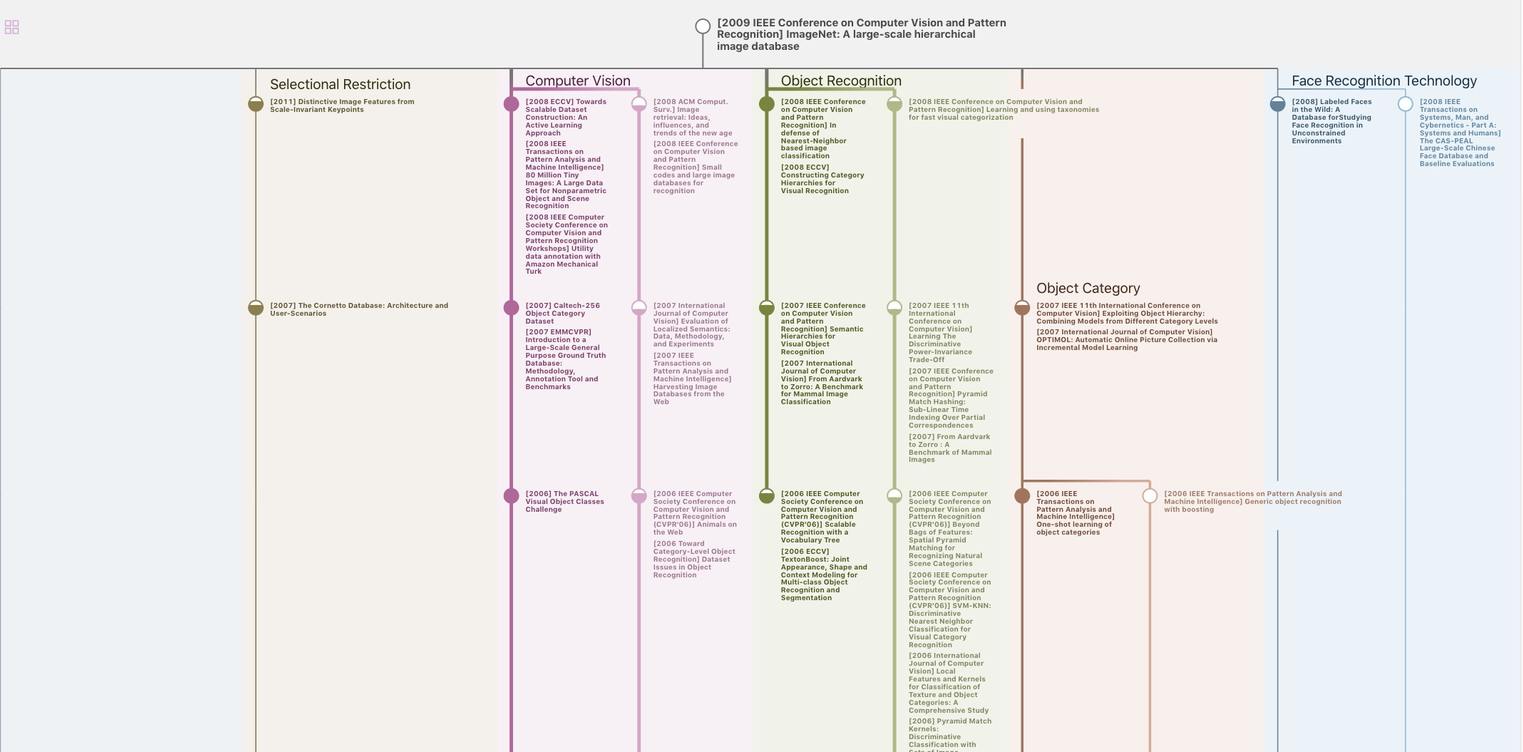
Generate MRT to find the research sequence of this paper
Chat Paper
Summary is being generated by the instructions you defined