Future Co‐Occurrences of Hot Days and Ozone‐Polluted Days Over China Under Scenarios of Shared Socioeconomic Pathways Predicted Through a Machine‐Learning Approach
Earth's Future(2022)
摘要
The warming climate increases the probability of hot days, which leads to a penalty effect of increasing ozone (O-3)-polluted days in polluted regions. Here, we established a random forest algorithm to predict future probabilities of O-3 exceedance (P) during hot days and further examined the future co-occurrences of O-3-polluted days and hot days under two different scenarios of Shared Socioeconomic Pathways (SSPs) 1-2.6 and 5-8.5 for 2030-2050s. Ground-level observations, simulated O-3-temperature sensitivities in the GEOS-Chem model, multimodel seasonal-mean O-3 concentrations and daily maximum temperature from the sixth Coupled Model Intercomparison Project (CMIP6), leaf area index from reanalyzed data, and local geographical information were comprehensively utilized. Evaluations showed that the algorithm captured the spatial patterns of present-day P values well with a correlation coefficient of 0.92 over China. Results showed that the strong reductions in anthropogenic emissions under SSP 1-2.6 significantly reduced the risks of O-3 exceedance during hot days nationwide from 3.7 days in the 2030s to 3.0 days in the 2050s. However, the SSP 5-8.5 scenario witnessed more frequent co-occurrences of O-3-polluted days and hot days over the 2030-2050s with nationally averaged values from 4.5 to 6.4 days. Our results highlight the co-benefits of reducing anthropogenic emissions to alleviate the composite risks of extreme weather events and air-polluted days in the future.
更多查看译文
关键词
ozone polluted days, hot days, random forest algorithm
AI 理解论文
溯源树
样例
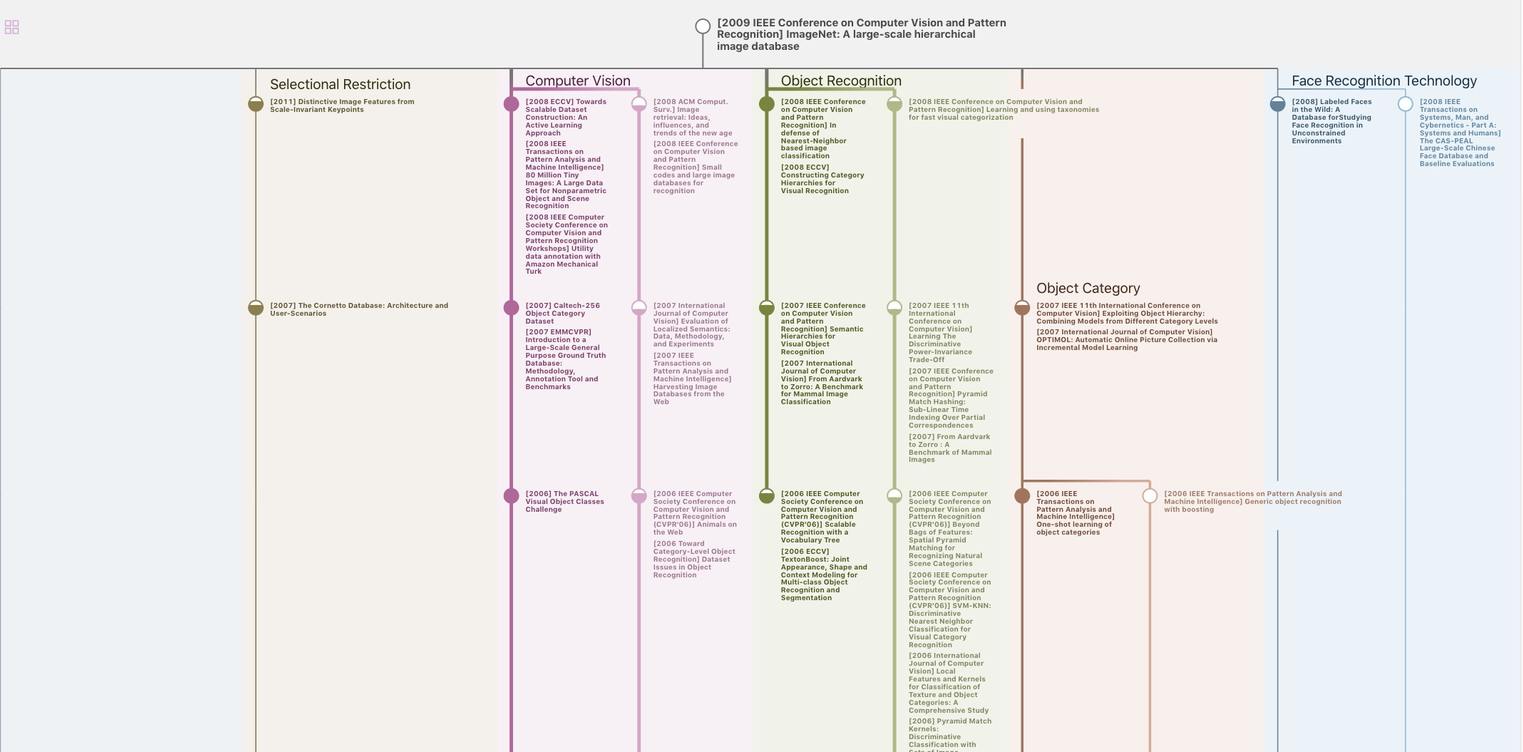
生成溯源树,研究论文发展脉络
Chat Paper
正在生成论文摘要