A machine learning-based optimization for end-to-end latency in TSN networks
Computer Communications(2022)
摘要
Ultra-low latency (ULL) is a challenging but nonetheless essential network requirement to achieve in many contexts. Industrial automation (IEC/IEEE 60802), in-vehicle communications (IEEE P802.1DG), audio and video bridging (IEEE Std 802.1BA), aerospace, and 5G fronthaul (IEEE 802.1CM) applications demand a low network latency in the order of few milliseconds or even microseconds. ULL services target a more controlled latency for end-to-end device communication and, in critical environments, near real-time connections. Time-Sensitive Networks (TSN) aim at providing deterministic connectivity over IEEE 802 networks guaranteeing packet transport with bounded latency, low packet delay variation, and low packet loss. This work analyzes TSN latency mechanisms and examines how they influence E2E latency. Moreover, it builds a regression-based surrogate model that enables the creation of optimization models to find optimal configurations for a given TSN network. Our findings span the discussion of the characteristics of each individual TSN latency mechanism to the benefits achieved by the proposed optimization models and using these to achieve optimal E2E latency.
更多查看译文
关键词
TSN,End-to-end latency,Network optimization,ULL
AI 理解论文
溯源树
样例
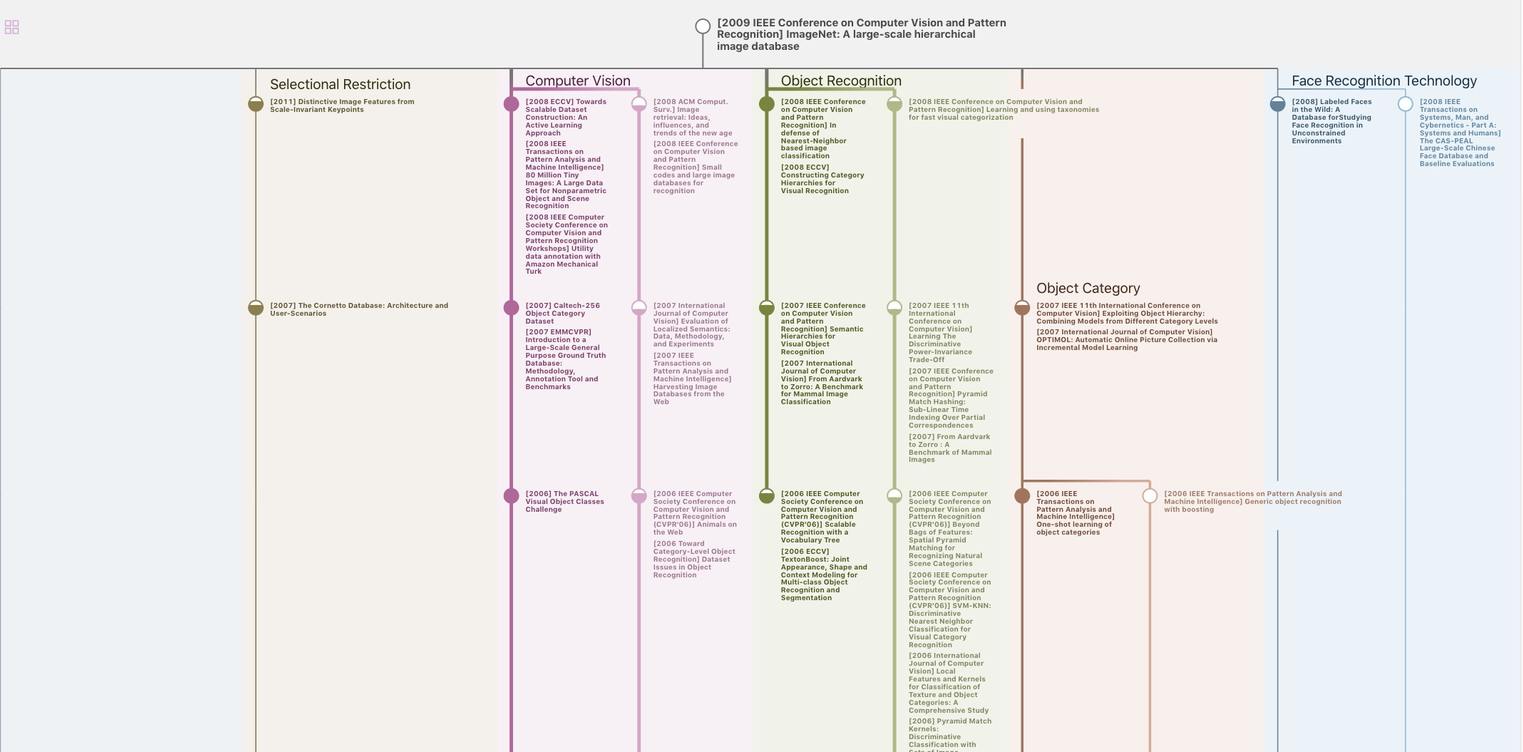
生成溯源树,研究论文发展脉络
Chat Paper
正在生成论文摘要