Sentiment Classification with Supervised Sequence Embedding.
ECML/PKDD (1)(2012)
摘要
In this paper, we introduce a novel approach for modeling n -grams in a latent space learned from supervised signals. The proposed procedure uses only unigram features to model short phrases ( n -grams) in the latent space. The phrases are then combined to form document-level latent representation for a given text, where position of an n -gram in the document is used to compute corresponding combining weight. The resulting two-stage supervised embedding is then coupled with a classifier to form an end-to-end system that we apply to the large-scale sentiment classification task. The proposed model does not require feature selection to retain effective features during pre-processing, and its parameter space grows linearly with size of n -gram. We present comparative evaluations of this method using two large-scale datasets for sentiment classification in online reviews (Amazon and TripAdvisor). The proposed method outperforms standard baselines that rely on bag-of-words representation populated with n -gram features.
更多查看译文
关键词
latent space,document-level latent representation,n-gram feature,parameter space,proposed method,proposed model,proposed procedure,bag-of-words representation,large-scale datasets,large-scale sentiment classification task,supervised sequence embedding
AI 理解论文
溯源树
样例
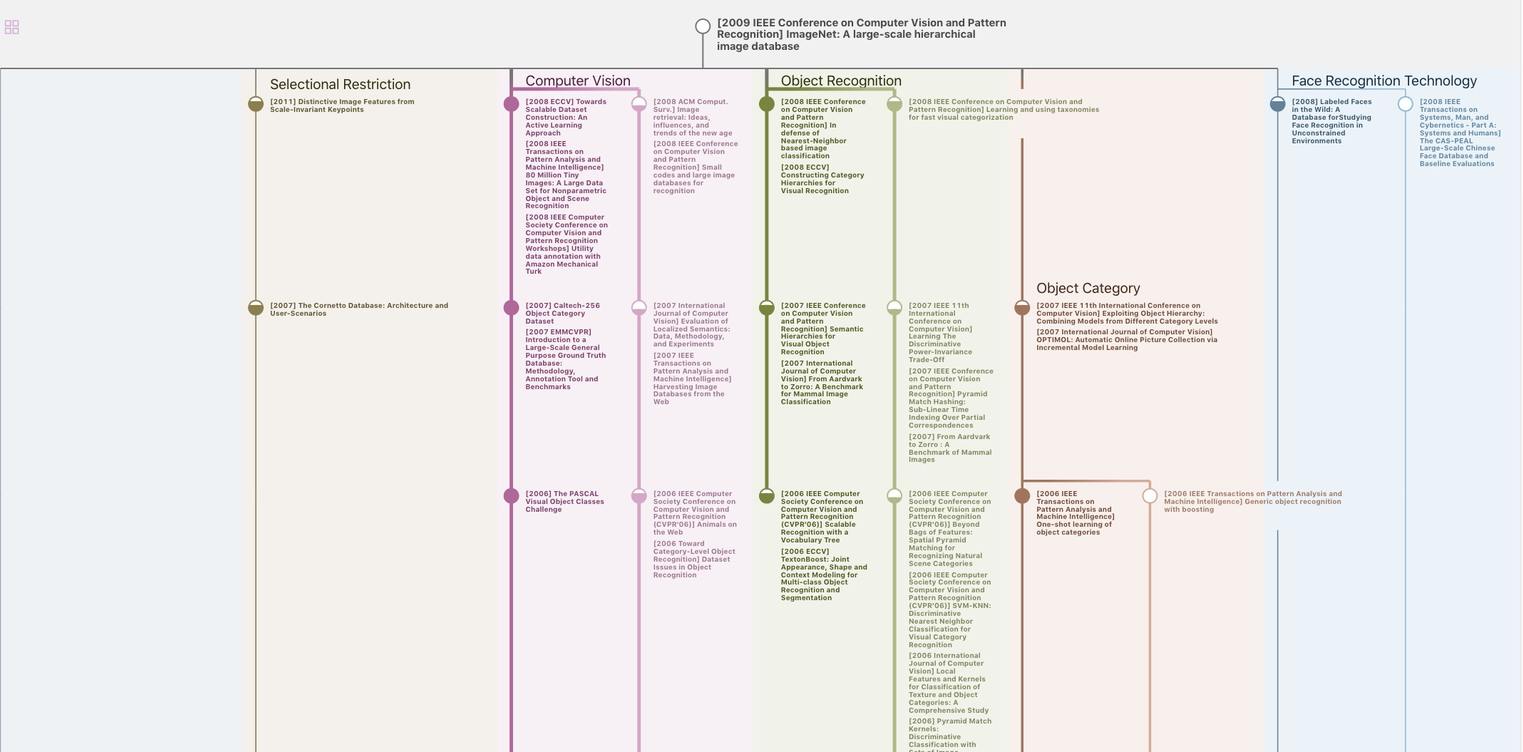
生成溯源树,研究论文发展脉络
Chat Paper
正在生成论文摘要