Unsupervised Semantic Segmentation with Self-supervised Object-centric Representations
ICLR 2023(2022)
摘要
In this paper, we show that recent advances in self-supervised feature learning enable unsupervised object discovery and semantic segmentation with a performance that matches the state of the field on supervised semantic segmentation 10 years ago. We propose a methodology based on unsupervised saliency masks and self-supervised feature clustering to kickstart object discovery followed by training a semantic segmentation network on pseudo-labels to bootstrap the system on images with multiple objects. We present results on PASCAL VOC that go far beyond the current state of the art (47.3 mIoU), and we report for the first time results on MS COCO for the whole set of 81 classes: our method discovers 34 categories with more than $20\%$ IoU, while obtaining an average IoU of 19.6 for all 81 categories.
更多查看译文
关键词
unsupervised semantic segmentation,object segmentation,object-centric learning
AI 理解论文
溯源树
样例
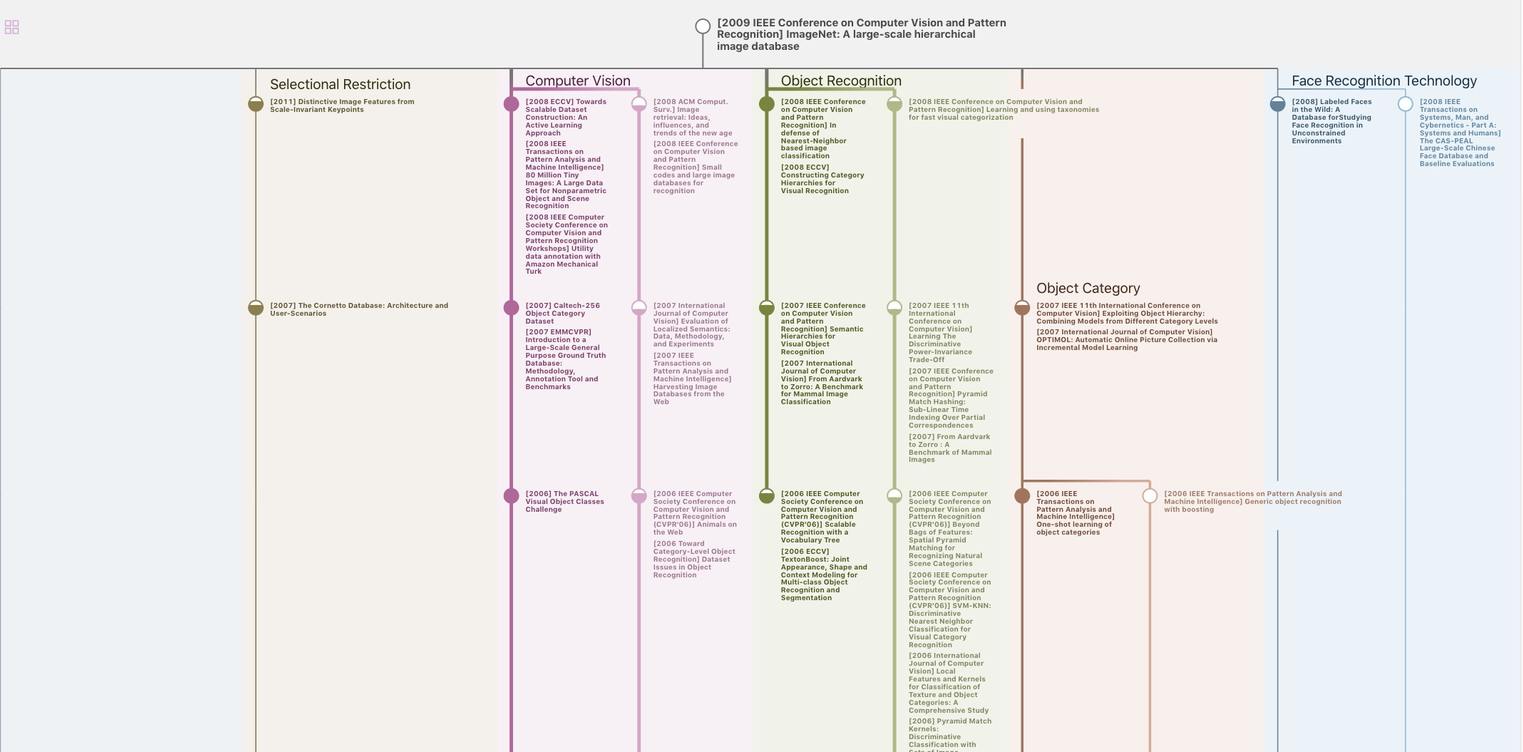
生成溯源树,研究论文发展脉络
Chat Paper
正在生成论文摘要