Covariate-adaptive randomization inference in matched designs
JOURNAL OF THE ROYAL STATISTICAL SOCIETY SERIES B-STATISTICAL METHODOLOGY(2024)
Abstract
It is common to conduct causal inference in matched observational studies by proceeding as though treatment assignments within matched sets are assigned uniformly at random and using this distribution as the basis for inference. This approach ignores observed discrepancies in matched sets that may be consequential for the distribution of treatment, which are succinctly captured by within-set differences in the propensity score. We address this problem via covariate-adaptive randomization inference, which modifies the permutation probabilities to vary with estimated propensity score discrepancies and avoids requirements to exclude matched pairs or model an outcome variable. We show that the test achieves type I error control arbitrarily close to the nominal level when large samples are available for propensity score estimation. We characterize the large-sample behaviour of the new randomization test for a difference-in-means estimator of a constant additive effect. We also show that existing methods of sensitivity analysis generalize effectively to covariate-adaptive randomization inference. Finally, we evaluate the empirical value of combining matching and covariate-adaptive randomization procedures using simulations and analyses of genetic damage among welders and right-heart catheterization in surgical patients.
MoreTranslated text
Key words
causal inference,matching,permutation test,propensity score,sensitivity analysis
AI Read Science
Must-Reading Tree
Example
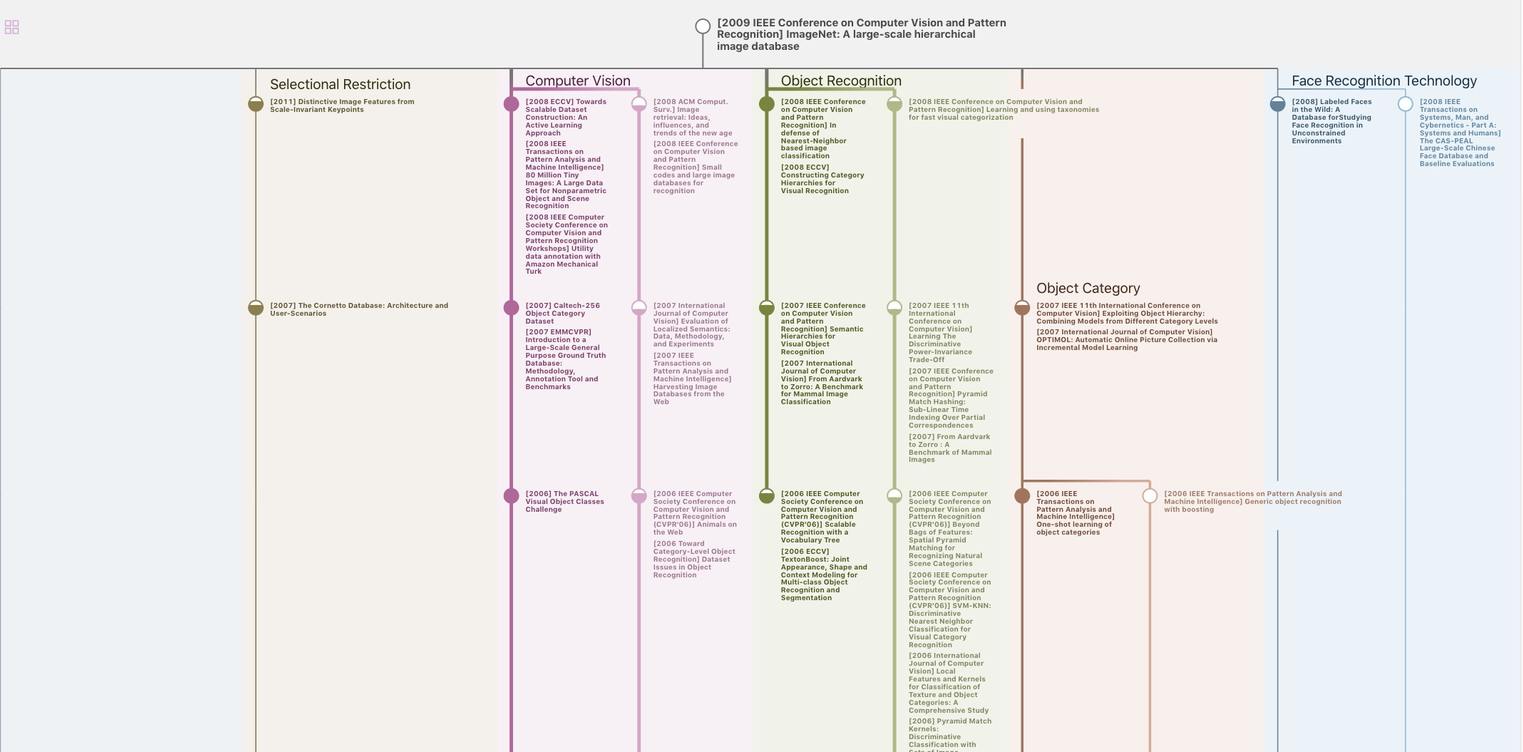
Generate MRT to find the research sequence of this paper
Chat Paper
Summary is being generated by the instructions you defined