Machine learning the trilinear and light-quark Yukawa couplings from Higgs pair kinematic shapes
arxiv(2022)
摘要
Revealing the Higgs pair production process is the next big challenge in high energy physics. In this work, we explore the use of interpretable machine learning and cooperative game theory for extraction of the trilinear Higgs self-coupling in Higgs pair production. In particular, we show how a topological decomposition of the gluon-gluon fusion Higgs pair production process can be used to simplify the machine learning analysis flow. Furthermore, we extend the analysis to include qq → hh production, which is strongly suppressed in the Standard Model, to extract the trilinear Higgs coupling and to bound large deviations of the light-quark Yukawa couplings from the Standard Model values. The constraints on the rescaling of the trilinear Higgs self-coupling, κ λ , and the rescaling of light-quark Yukawa couplings, κ u and κ d , at HL-LHC (FCC-hh) from single parameter fits are: [ κ_λ=[0.53,1.7] ([0.97,1.03]); κ_u=[-470,430] ([-58,55]); κ_d=[-360,360] ([-26,28]) ] We show that the simultaneous modification of the Yukawa couplings can dilute the constraints on the trilinear coupling significantly. We perform similar analyses for FCC-hh. We discuss some motivated flavourful new physics scenarios where such an analysis prevails.
更多查看译文
关键词
Anomalous Higgs Couplings,SMEFT
AI 理解论文
溯源树
样例
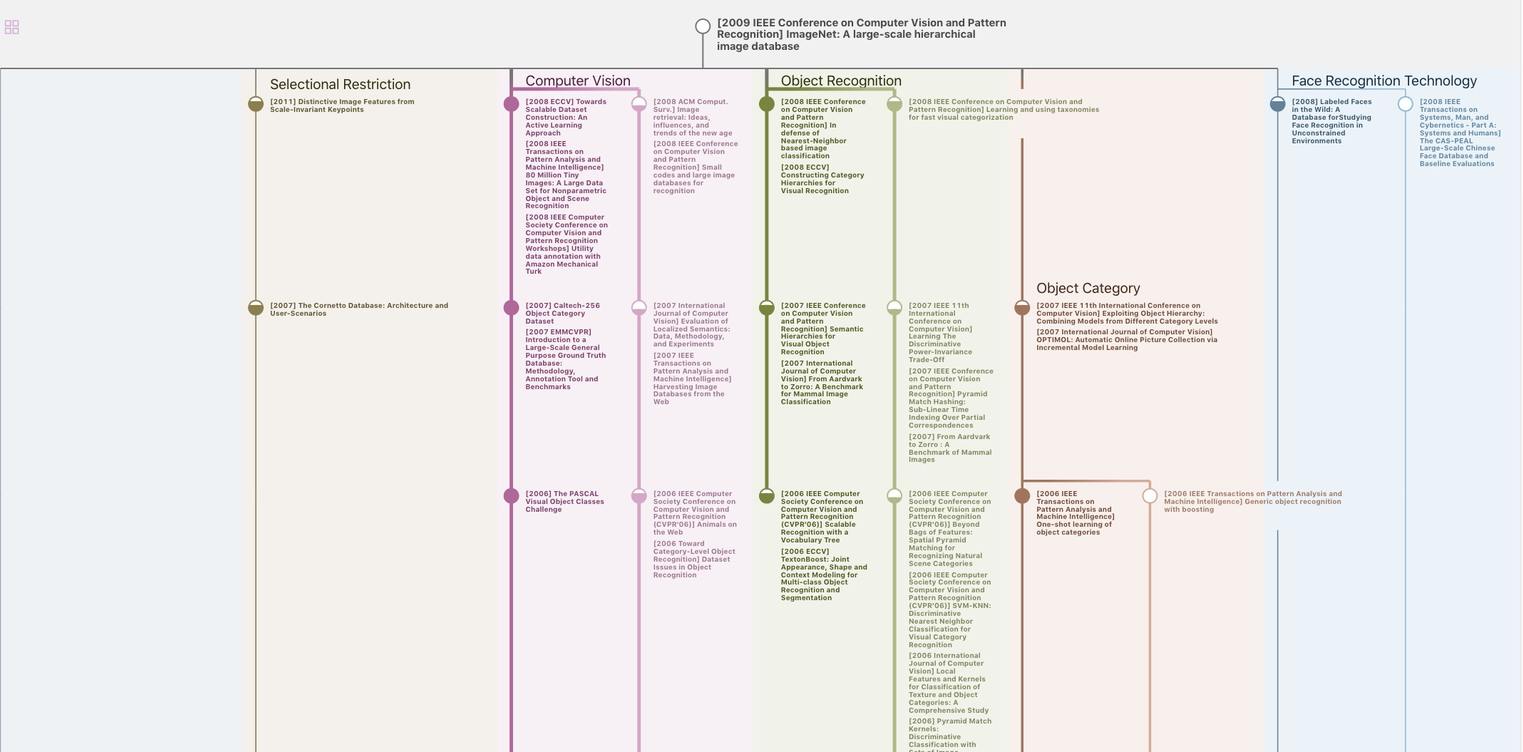
生成溯源树,研究论文发展脉络
Chat Paper
正在生成论文摘要