Supervised Machine Learning for Effective Missile Launch Based on Beyond Visual Range Air Combat Simulations
arXiv (Cornell University)(2022)
摘要
This work compares supervised machine learning methods using reliable data from constructive simulations to estimate the most effective moment for launching missiles during air combat. We employed resampling techniques to improve the predictive model, analyzing accuracy, precision, recall, and f1-score. Indeed, we could identify the remarkable performance of the models based on decision trees and the significant sensitivity of other algorithms to resampling techniques. The models with the best f1-score brought values of 0.379 and 0.465 without and with the resampling technique, respectively, which is an increase of 22.69%. Thus, if desirable, resampling techniques can improve the model's recall and f1-score with a slight decline in accuracy and precision. Therefore, through data obtained through constructive simulations, it is possible to develop decision support tools based on machine learning models, which may improve the flight quality in BVR air combat, increasing the effectiveness of offensive missions to hit a particular target.
更多查看译文
关键词
BVR air combat,decision support tools,decision trees,missile launch,predictive model,resampling technique,supervised machine learning,visual range air combat constructive simulations
AI 理解论文
溯源树
样例
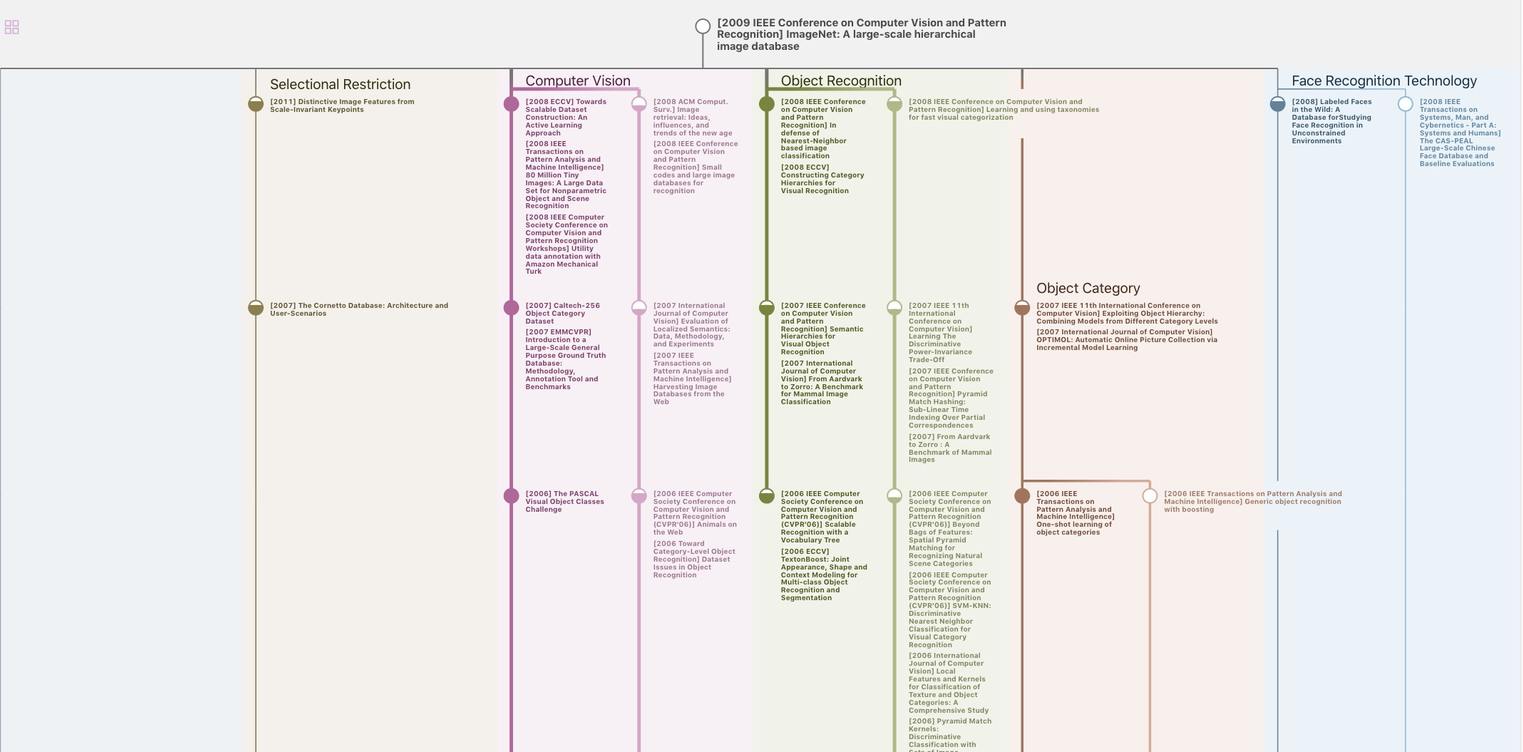
生成溯源树,研究论文发展脉络
Chat Paper
正在生成论文摘要