Bayesian Interpretation of Regularization
Regularized System IdentificationCommunications and Control Engineering(2022)
摘要
AbstractIn the previous chapter, it has been shown that the regularization approach is particularly useful when information contained in the data is not sufficient to obtain a precise estimate of the unknown parameter vector and standard methods, such as least squares, yield poor solutions. The fact itself that an estimate is regarded as poor suggests the existence of some form of prior knowledge on the degree of acceptability of candidate solutions. It is this knowledge that guides the choice of the regularization penalty that is added as a corrective term to the usual sum of squared residuals. In the previous chapters, this design process has been described in a deterministic setting where only the measurement noises are random. In this chapter, we will see that an alternative formalization of prior information is obtained if a subjective/Bayesian estimation paradigm is adopted. The major difference is that the parameters, rather than being regarded as deterministic, are now treated as a random vector. This stochastic setting permits the definition of new powerful tools for both priors selection, e.g., through the maximum entropy principle, and for regularization parameters tuning, e.g., through the empirical Bayes approach and its connection with the concept of equivalent degrees of freedom.
更多查看译文
关键词
regularization
AI 理解论文
溯源树
样例
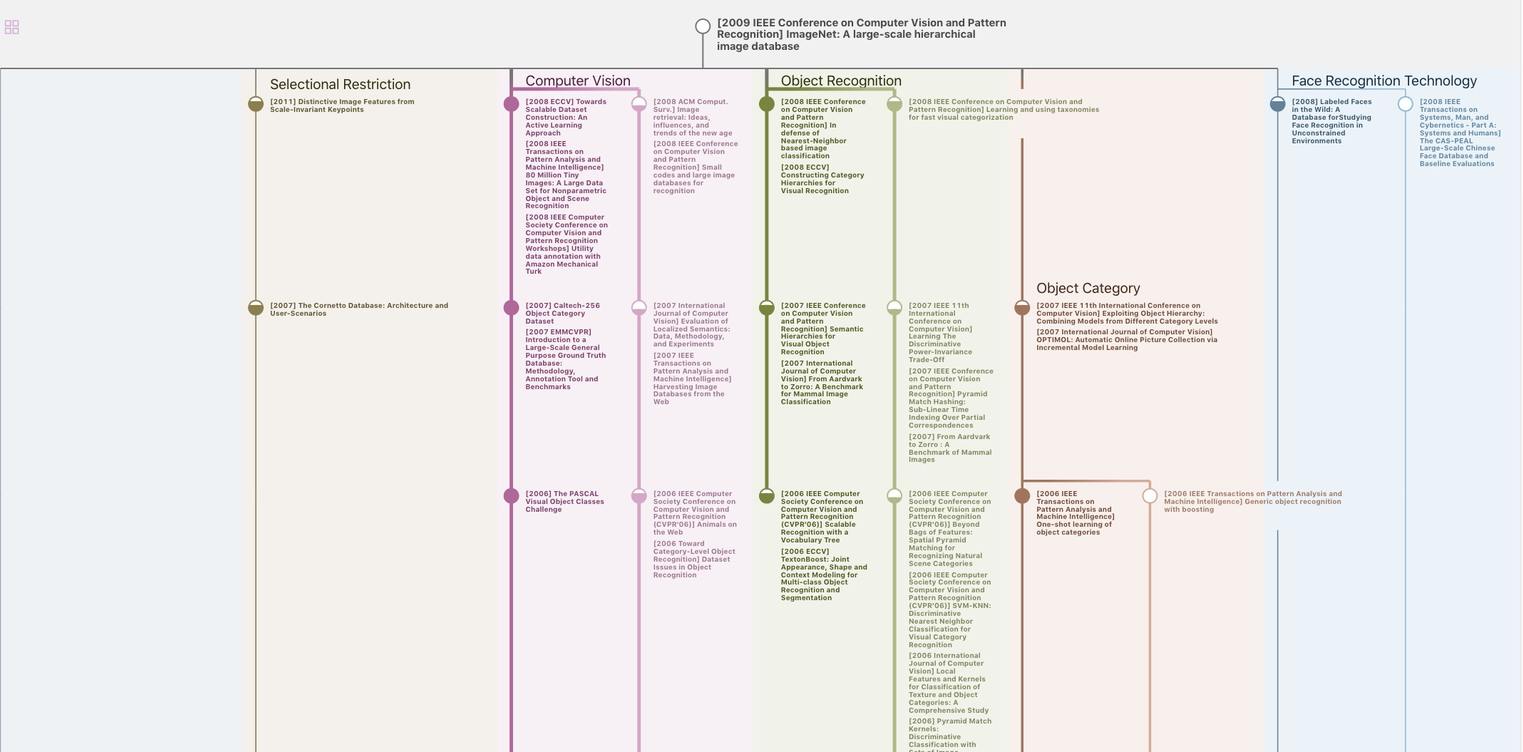
生成溯源树,研究论文发展脉络
Chat Paper
正在生成论文摘要