MLFF-GAN: A Multilevel Feature Fusion With GAN for Spatiotemporal Remote Sensing Images
IEEE TRANSACTIONS ON GEOSCIENCE AND REMOTE SENSING(2022)
摘要
Due to the limitation of technology and budget, it is often difficult for sensors of a single remote sensing satellite to have both high-temporal and high-spatial (HTHS) resolution at the same time. In this article, we proposed a new multilevel feature fusion with generative adversarial network (MLFF-GAN) for generating fusion HTHS images. The MLFF-GAN mainly uses U-net-like architecture, and its generator is composed of three stages: feature extraction, feature fusion, and image reconstruction. In the feature extraction and reconstruction stage, the generator employs the encoding and decoding structure to extract three groups of multilevel features (MLFs), which can cope with the huge difference of resolution between high-resolution images and low-resolution images. In the feature fusion stage, adaptive instance normalization (AdaIN) block is designed to learn the global distribution relationship between multitemporal images, and an attention module (AM) is used to learn the local information weights for the change of small areas. The proposed MLFF-GAN was tested on two Landsat and Moderate-Resolution Imaging Spectroradiometer (MODIS) datasets. Some state-of-the-art algorithms are comprehensively compared with MLFF-GAN. We also carried on the ablation experiment to test the effectiveness of different submodules in the MLFF-GAN. The experiment results and ablation analysis show the better performances of the proposed method when compared with other methods. The code is available at https://github.com/songbingze/MLFF-GAN.
更多查看译文
关键词
Spatial resolution,Feature extraction,Generators,Generative adversarial networks,Spatiotemporal phenomena,Image resolution,Image reconstruction,Adaptive instance normalization (AdaIN),generative adversarial network (GAN),spatial attention mechanism,U-net
AI 理解论文
溯源树
样例
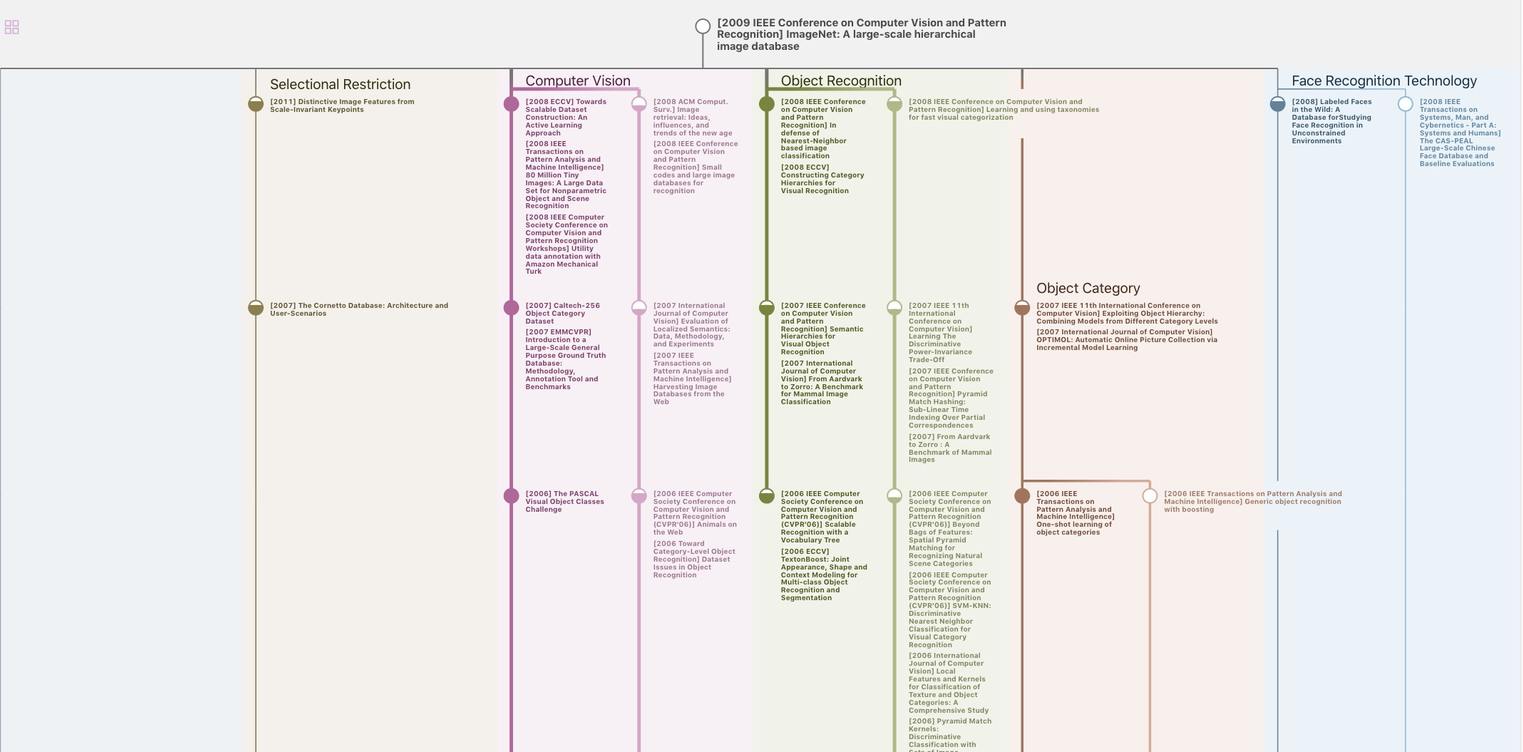
生成溯源树,研究论文发展脉络
Chat Paper
正在生成论文摘要