HELPHED: Hybrid Ensemble Learning PHishing Email Detection
Journal of Network and Computer Applications(2023)
摘要
Phishing email attack is a dominant cyber-criminal strategy for decades. Despite its longevity, it has evolved during the COVID-19 pandemic, indicating that adversaries exploit critical situations to lure victims. Plenty of detectors have been proposed over the years, which mainly focus on the contents or the textual information of emails; however, to cope with the evolution of phishing emails more sophisticated approaches should be introduced that will exploit all the emails’ traits to enhance the detection capability of Machine Learning/Deep Learning classifiers. To tackle the limitations of existing works, this paper proposes a phishing email detection methodology, named HELPHED that focuses on the detection of phishing emails by combining Ensemble Learning methods with hybrid features. The hybrid features provide an accurate representation of emails by fusing their content and textual traits. We propose two methods of HELPHED, the first one employs the Stacking Ensemble Learning method, while the second method utilizes the Soft Voting Ensemble Learning. Both methods deploy two different Machine Learning algorithms to handle the hybrid features separately, yet in parallel, minimizing the features’ complexity and improving the model’s performance. A thorough evaluation analysis is carried out considering innovative guidelines that aim to prevent partial and misleading results. Experimental tests verified that the combination of hybrid features with Ensemble Learning, overall, accomplishes better detection performance than when employing only content-based or text-based features. Numerical results on a rich imbalanced dataset (i.e., 32,051 benign and 3,460 phishing email samples) that considers the evolution of phishing emails show that Soft Voting Ensemble Learning outperforms other prominent Machine Learning/Deep Learning algorithms and existing works yielding F1-score equal to 0.9942.
更多查看译文
关键词
Phishing email detection,Machine Learning,Ensemble Learning,Hybrid features,Natural Language Processing
AI 理解论文
溯源树
样例
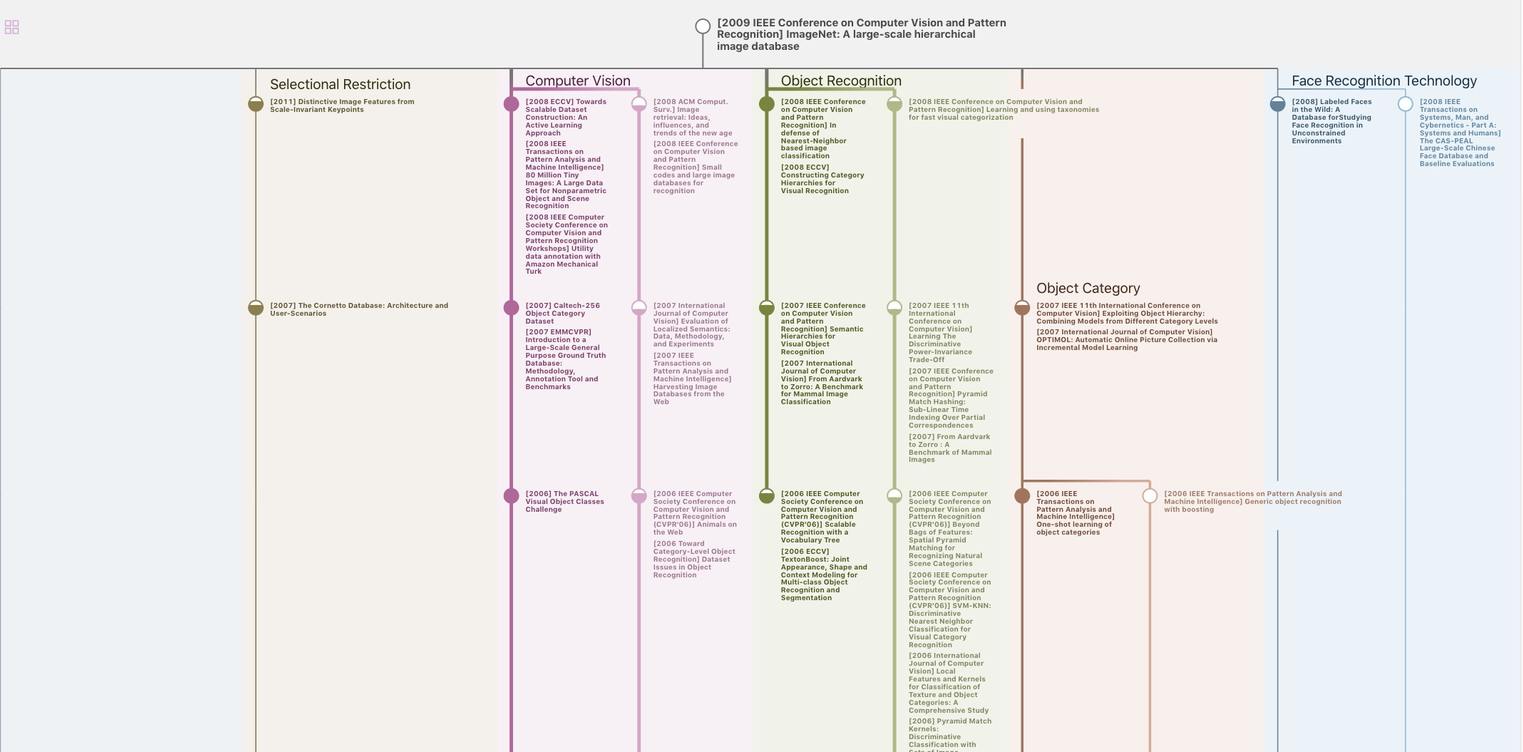
生成溯源树,研究论文发展脉络
Chat Paper
正在生成论文摘要