Selective kernel convolution deep residual network based on channel-spatial attention mechanism and feature fusion for mechanical fault diagnosis.
ISA transactions(2023)
Abstract
This paper proposes a selective kernel convolution deep residual network based on the channel-spatial attention mechanism and feature fusion for mechanical fault diagnosis. First, adjacent channel attention modules are connected with the spatial attention mechanism module, then all channel features and spatial features are fused and a channel-spatial attention mechanism is constructed to form the feature enhancement module. Second, the feature enhancement module is embedded in a series model based on selective kernel convolution and deep residual network and combined with multi-layer feature fusion information. The model can more effectively extract fault features from the vibration signal, compared with traditional deep learning methods, and the fault recognition efficiency is improved. Finally, the proposed method was used to experimentally diagnose bearing and gear faults, and identification accuracies of 99.87% and 97.77%, respectively, were achieved. Compared with similar algorithms, the proposed method has higher fault identification ability, thereby demonstrating the advantages of the channel-spatial attention mechanism network. In addition, the accuracy and robustness of the model were verified.
MoreTranslated text
Key words
Channel-spatial attention mechanism,Feature fusion,Mechanical fault diagnosis,Residual network,Selective kernel convolution
AI Read Science
Must-Reading Tree
Example
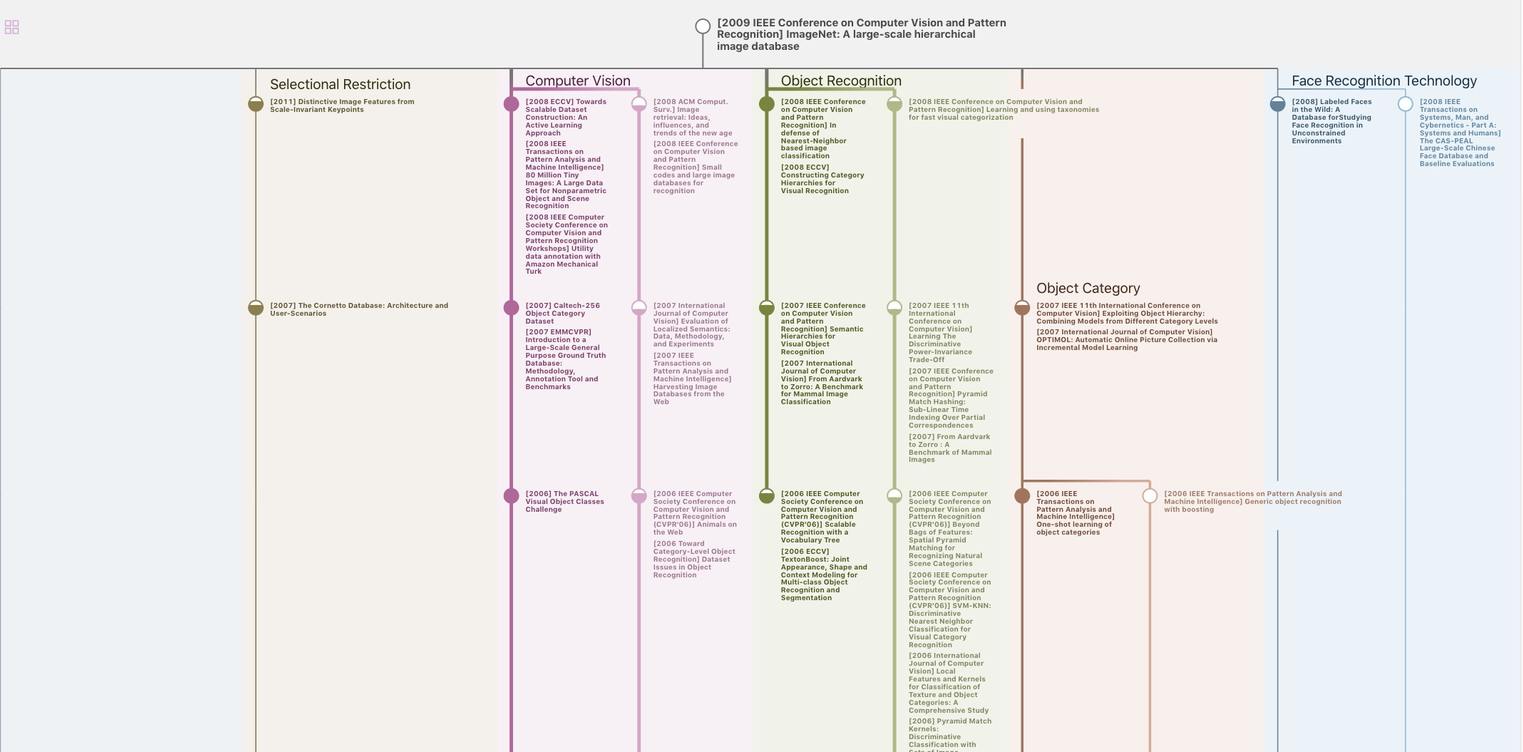
Generate MRT to find the research sequence of this paper
Chat Paper
Summary is being generated by the instructions you defined