Multi-scale Sinusoidal Embeddings Enable Learning on High Resolution Mass Spectrometry Data
CoRR(2022)
摘要
Small molecules in biological samples are studied to provide information about disease states, environmental toxins, natural product drug discovery, and many other applications. The primary window into the composition of small molecule mixtures is tandem mass spectrometry (MS2), which produces data that are of high sensitivity and part per million resolution. We adopt multi-scale sinusoidal embeddings of the mass data in MS2 designed to meet the challenge of learning from the full resolution of MS2 data. Using these embeddings, we provide a new state of the art model for spectral library search, the standard task for initial evaluation of MS2 data. We also introduce a new task, chemical property prediction from MS2 data, that has natural applications in high-throughput MS2 experiments and show that an average $R^2$ of 80\% for novel compounds can be achieved across 10 chemical properties prioritized by medicinal chemists. We use dimensionality reduction techniques and experiments with different floating point resolutions to show the essential role multi-scale sinusoidal embeddings play in learning from MS2 data.
更多查看译文
关键词
Cheminformatics for Life Sciences,Tandem Mass Spectrometry,Transformers
AI 理解论文
溯源树
样例
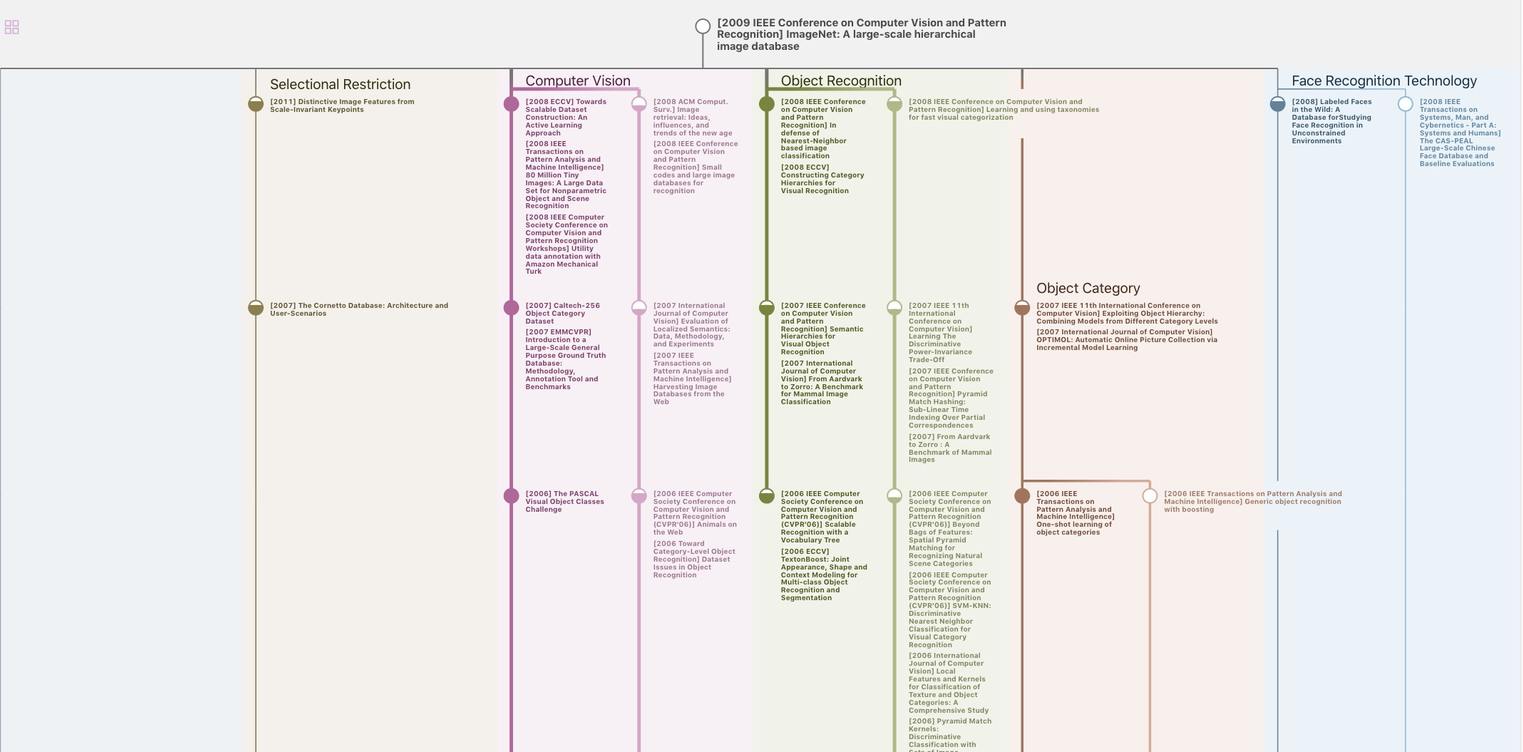
生成溯源树,研究论文发展脉络
Chat Paper
正在生成论文摘要