Multiple Spectral-Spatial Representation Based on Tensor Decomposition for HSI Anomaly Detection
IEEE JOURNAL OF SELECTED TOPICS IN APPLIED EARTH OBSERVATIONS AND REMOTE SENSING(2022)
摘要
To exploit the spectral-spatial information of hyperspectral image (HSI) and achieve higher the detection accuracy, a novel multiple spectral-spatial representation based on tensor decomposition method is proposed for HSI anomaly detection (AD) in this article. First, tensor decomposition is employed to separate the original HSI dataset into anomalous tensor from background tensor to, respectively, utilize the priors of background and anomaly. Then, the segmented smoothing prior is characterized by l0-l1 hybrid total variation regularization for the spatial dimensions of the background tensor, which can not only complement the image details but also fully consider the global spectral-spatial structure. Moreover, the low-rank prior is represented with truncated nuclear norm regularization for the spectral dimension of the background tensor to make full utilization of global information in the background and reduce data redundancy. Next, l2,1-norm regularization is applied to characterize the sparse prior for the anomaly tensor, which is beneficial to inscribe the local spectral structure of each image element. Finally, all these priors are fuses to model a unified convex optimization problem and obtain the final results by alternating direction method of multipliers, which can fully explore the discriminative spectral-spatial priors and improve the AD accuracy significantly. Experiments carried out on several real data indicate that our presented method reaches excellent performance in comparison to a few advanced AD methods.
更多查看译文
关键词
Licenses,1-norm,hyperspectral image (HIS) anomaly detection,l0-l1 hybrid TV (l0-l1HTV),l2,truncated nuclear norm (TNN)
AI 理解论文
溯源树
样例
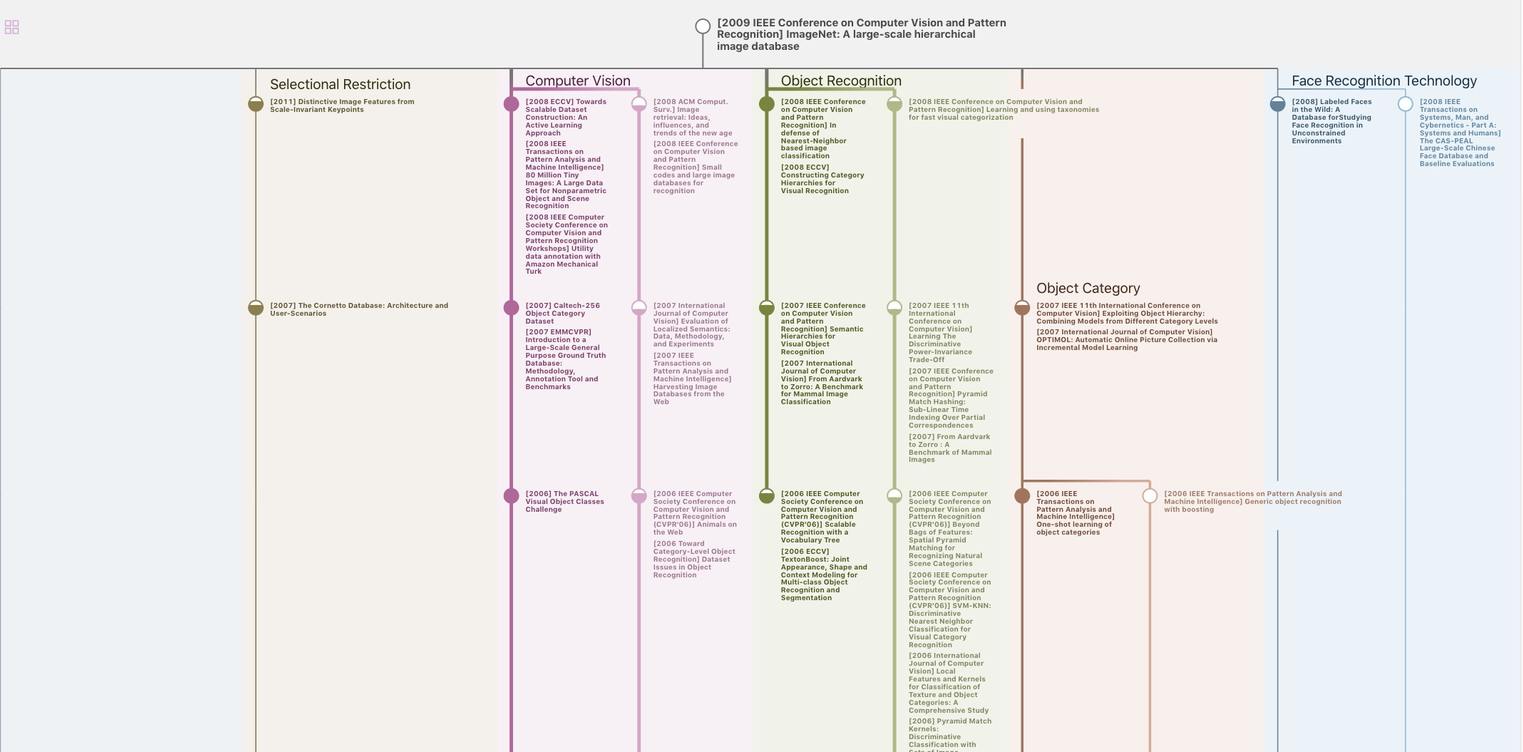
生成溯源树,研究论文发展脉络
Chat Paper
正在生成论文摘要