Machine Health Management System Using Moving Average Feature With Bidirectional Long-Short Term Memory.
Journal of Computing and Information Science in Engineering(2023)
摘要
In today's highly competitive industrial environment, machine health management systems become a crucial factor for sustainability and success. The traditional feature extraction methods to reveal the health condition of the machine are labor-extensive. They usually depend on engineered design features, which require an expert knowledge level. Inspired by the successful results of deep-learning approaches that redefine representation learning from raw data, we propose moving-averaged features-based on Long-Short Term Memory (MaF-LSTM) networks. It is a hybrid approach that combines engineered features design with self-feature learning for the purpose of machine condition monitoring. First, features from overlapped sliding windows of the input time-series signals are extracted. Then, a moving-average filter is applied on the top of the generated features to enhance the feature's condition indicter's content. Next, a bidirectional LSTM is applied to learn the feature representation from the moving-averaged features. Two experiments, namely, bearing fault diagnosis and hydraulic accumulator fault detection, are implemented to verify the effectiveness of the proposed MaF-LSTM. The experimental results demonstrated that the proposed method outperforms all traditional condition monitoring methods in both use cases.
更多查看译文
关键词
moving average feature,memory,machine,long-short
AI 理解论文
溯源树
样例
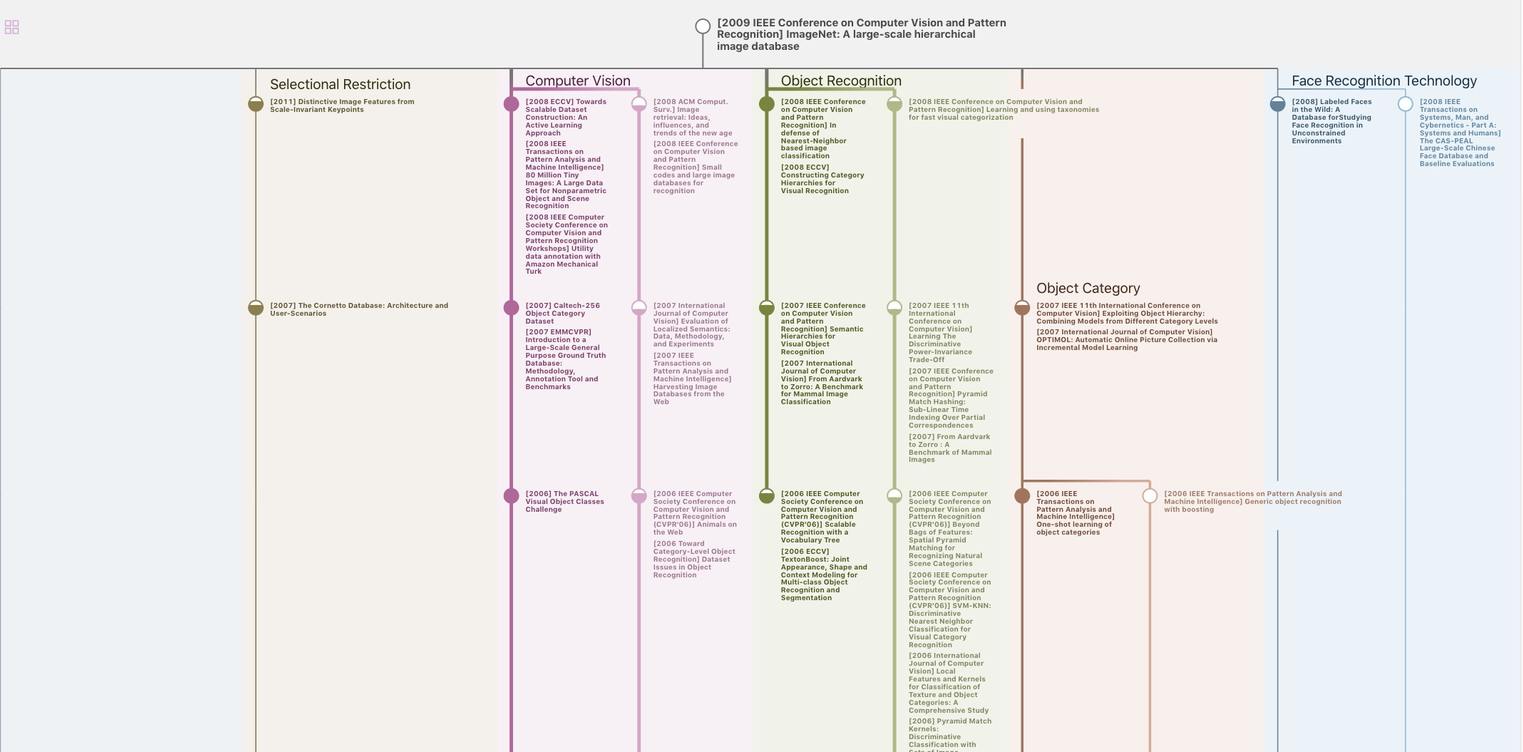
生成溯源树,研究论文发展脉络
Chat Paper
正在生成论文摘要