A Memory-Based Filter for Long-Term Error De-Noising of MEMS-Gyros
IEEE TRANSACTIONS ON INSTRUMENTATION AND MEASUREMENT(2022)
摘要
The navigation algorithms which use inertial measurement units (IMUs), such as inertial navigation systems (INSs), always suffer from intrinsic accumulated errors. Bias in gyros induces a significant drift in navigation output especially when micro-electro-mechanical sensor (MEMS) type is used. This error has high- and low-frequency components. De-noising of the long-term error (LTE) (the low-frequency component) is more challenging due to undeterministic behavior and overlapping with carrier motion in the low-frequency band. In this article, a method for de-noising of long-term MEMS-based gyro is presented. In this approach, an auto-regressive (AR) model for the LTE is developed which is being used as the process part of a Kalman filter. To separate the low-band motion dynamic from LTE in the measurement part of the Kalman Filter, the last time epoch of gyro data is subtracted from the current time epoch (memory-based filter). Some static and dynamic experiments have been done for the algorithm evaluation. The static test shows the reduction of LTE by 50%. Also, the method is invoked in INS/Doppler velocity log (DVL) integrated navigation system as a gyro prefilter. The results show that drift and point-to-point final error in position are reduced between 4% and 70% by invoking the de-noising method for gyros.
更多查看译文
关键词
Auto-regressive (AR) model,bias de-noising,drift reduction,gyro de-noising,long-term errors (LTEs),memory-based filtering,micro-electro-mechanical sensor (MEMS)
AI 理解论文
溯源树
样例
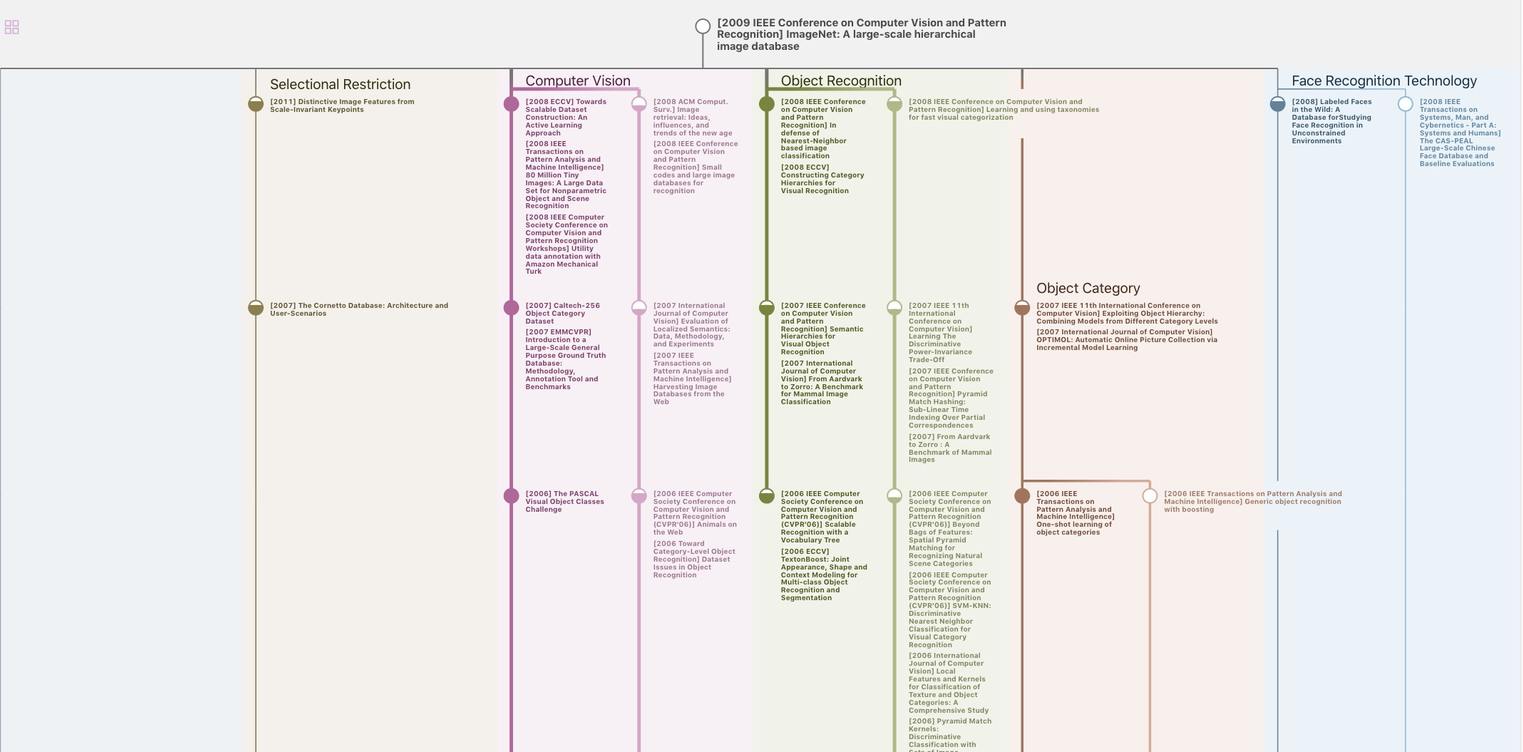
生成溯源树,研究论文发展脉络
Chat Paper
正在生成论文摘要