Man vs machine: Crowdsourcing vs deep learning of adaptive therapy strategies
Cancer Research(2022)
摘要
Abstract Cancer Crusade is a citizen science game in which players design treatment strategies to influence a virtual tumor growth model. The treatment regimens that the players construct are collected and sent to an online database for analysis, which may give insights into clinically effective adaptive strategies. In parallel, a machine learning-based approach was applied to the game to find optimal treatment policies, and the optimized therapy approaches generated are compared to those generated by the human players. For the core game engine in Cancer Crusade, we started with a previously published hybrid cellular automata model of tumor metabolism and growth. The original model was used to study how tumor cells evolve acid-mediated invasion and the impact of treatment on these metabolic processes. We expanded the model by adding more drugs and cell phenotypes, including drug-resistant cells. The game was released on mobile platforms and has been generating data from plays for several years. The treatment strategies were analyzed using dendrogram clustering to find key decision differences and profile their relative performance. We also performed network analysis to observe treatment transitions. These analyses indicated several effective adaptive strategies, which tended to oscillate between chemotherapy and a pro-angiogenic drug, or chemotherapy and a hypoxia-activated prodrug, or combinations of all three of these drugs. These results parallel a machine learning (Q-learning) approach to the problem, which yielded a preferred strategy based on chemotherapy combined with a pro-angiogenic drug. The existence of several human-discovered alternative strategies suggests that in general human players may offer greater variety of successful strategies via citizen science games than Q-learning. The human and machine learning approaches also excelled in different areas. Human players tended to use less drug overall, and perform best on less aggressive tumors, while the Q-learning approach used more drug overall and performed best on more aggressive tumors. Citation Format: Rafael R. Bravo, Brenden K. Peterson, Mark Robertson-Tessi, Daniel M. Faissol, Alexander R.A. Anderson. Man vs machine: Crowdsourcing vs deep learning of adaptive therapy strategies [abstract]. In: Proceedings of the AACR Special Conference on the Evolutionary Dynamics in Carcinogenesis and Response to Therapy; 2022 Mar 14-17. Philadelphia (PA): AACR; Cancer Res 2022;82(10 Suppl):Abstract nr B008.
更多查看译文
AI 理解论文
溯源树
样例
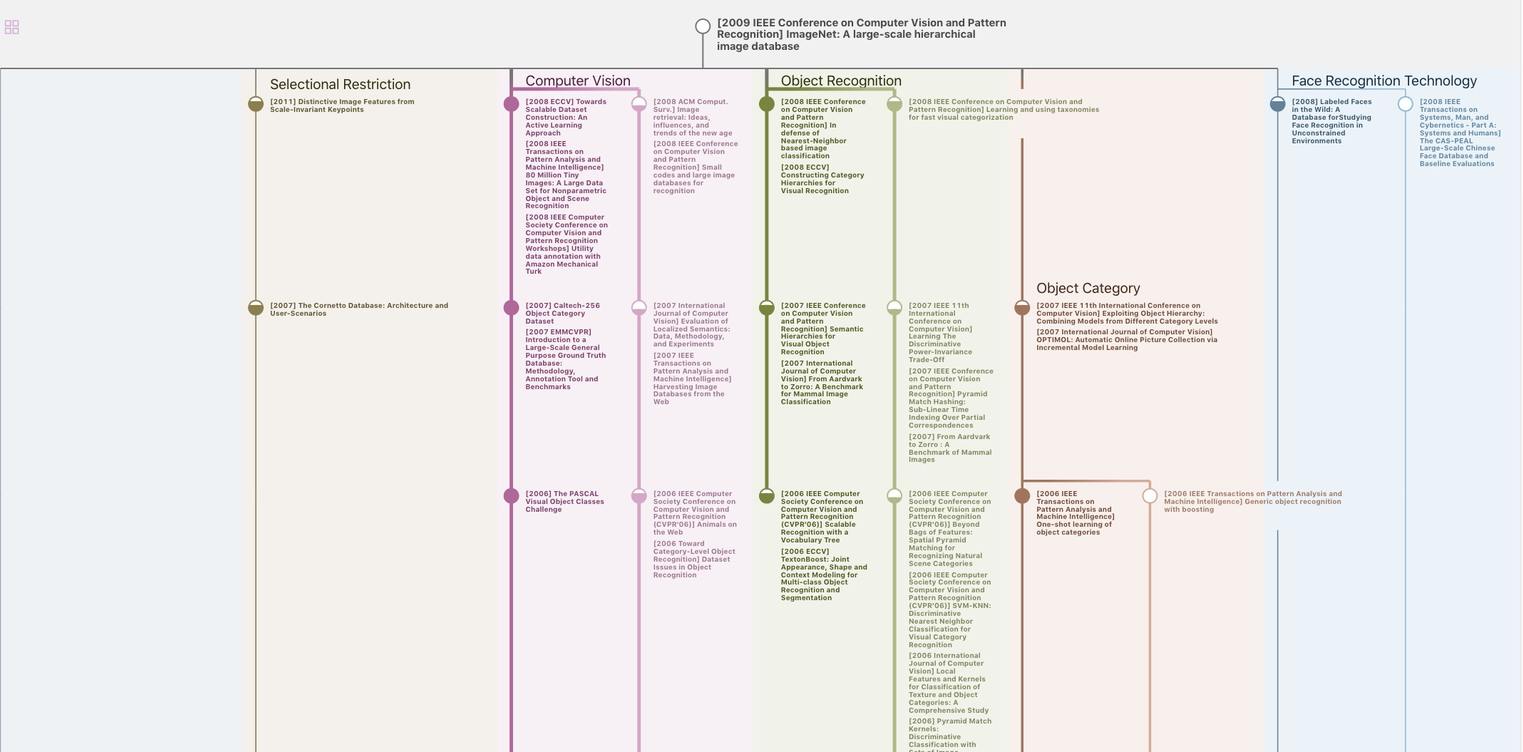
生成溯源树,研究论文发展脉络
Chat Paper
正在生成论文摘要