BUILDING FOOTPRINT EXTRACTION FROM SPACE- BORNE IMAGERY USING DEEP NEURAL NETWORKS
The International Archives of the Photogrammetry, Remote Sensing and Spatial Information Sciences(2022)
Abstract
One of the important and high-level detailing contained within basemaps is the 'building feature'. Though pre-trained Deep Learning (DL) models are available for Building Feature Extraction (BFE), they are not efficient in predicting the buildings in other locations. This study explores the need and the major issue of implementing DL models for BFE from Very High Resolution Remote Sensing (VHRS) satellite data for any given area. Though advanced DL models are invented, in order to implement them, huge amount of potential training data is demanded for feed in. the building typologies are highly subjected to the context of study area including soil characteristics, culture/lifestyle/economy, architectural style and the building byelaws. The study believes that availability of enough training data of contextual buildings as one of the concern for effective model training. The study aims to extract the buildings present in the study area from Pleiades 1A (2019) RGB VHRS data using simple Mask R-CNN instance segmentation model which is training on the native contextual buildings. Here, an automated method of generating the location-specific training data for a given area is followed using Google Maps API (2021). The generated training data when trained on a deep learning architecture and predicted by the input data yielded promising results. The prediction accuracy of about 98.41% specificity, 96.20% predictive accuracy and 0.89 F1 score are achieved. The methods adopted assist the planning/governing bodies to accelerate the qualitative urban map preparation.
MoreTranslated text
Key words
Deep Learning, Building foot-pint extraction, Mask RCNN, Open Source training data, Open Street Map, Remote Sensing, Satellite imagery
AI Read Science
Must-Reading Tree
Example
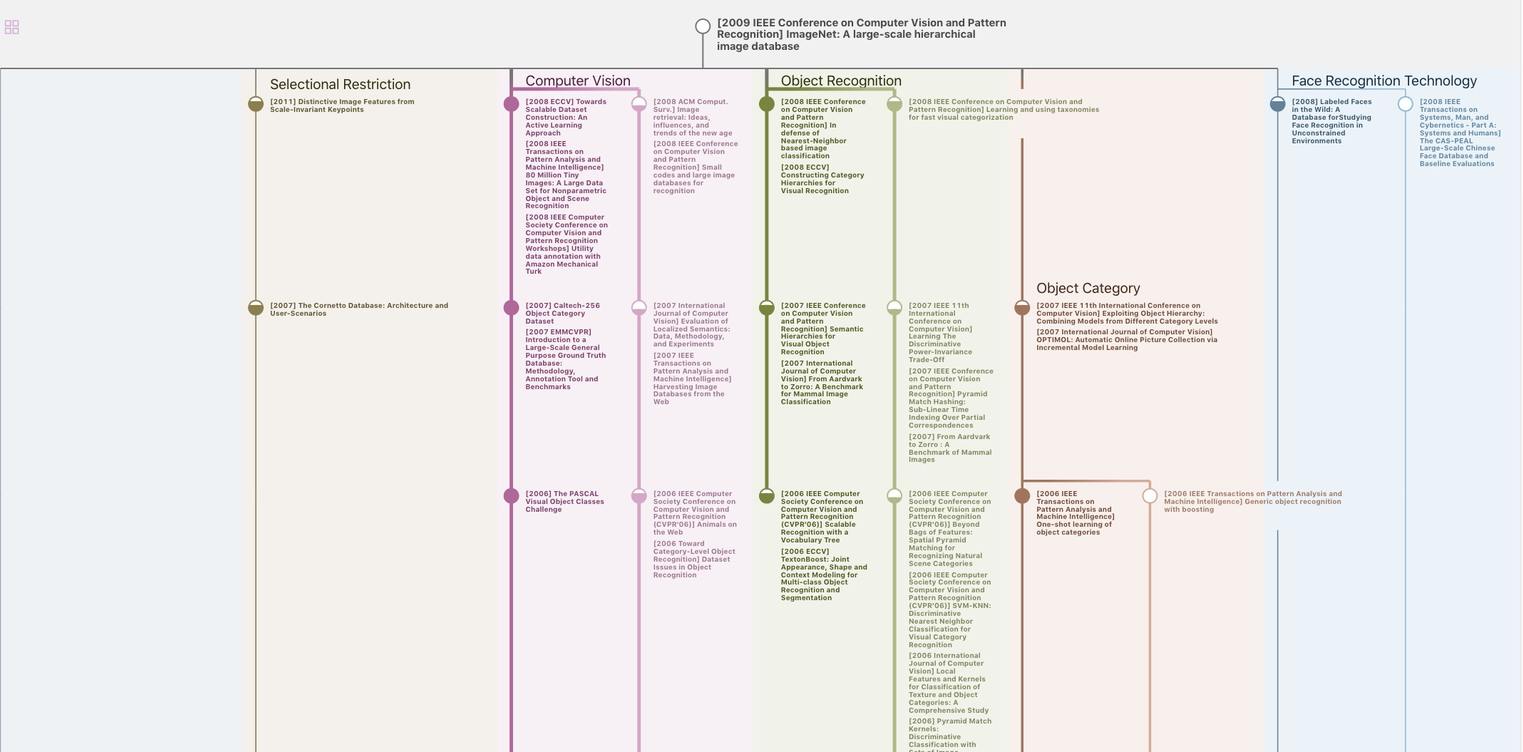
Generate MRT to find the research sequence of this paper
Chat Paper
Summary is being generated by the instructions you defined