Lightweight Indoor Personnel Detection Algorithm Based on Improved YOLOv4-tiny
JOURNAL OF ELECTRONICS & INFORMATION TECHNOLOGY(2022)
摘要
Deep learning has been widely applied to the field of indoor personnel detection. However, the traditional convolutional neural networks have a high complexity and require the support of highly computational GPU. It is difficult to accomplish the implementation in the embedded devices. For the above problems, a lightweight network model based on improved YOLOv4- tiny network is proposed for indoor personnel detection. Firstly, an improved Ghost convolution feature extraction module is designed to reduce effectively the model complexity. Simultaneously, to reduce network parameters, a depth-wise separable convolution with channel shuffle mechanism is adopted in this paper. Secondly, a multi- scale dilated convolution module is developed in this paper to obtain more discriminative feature information, which combines the improved dilated space pyramid pooling module and the attention mechanism with location information for effective feature fusion, thereby improving inference accuracy and inference speed, simultaneously. The experiments on multiple datasets and hardware platforms show that the proposed model is superior to the original YOLOv4-tiny network in terms of accuracy, speed, model parameters and volume. Therefore, the proposed model is more suitable for deployment in resource-limited embedded devices.
更多查看译文
关键词
Indoor personnel detection,Deep learning,YOLOv4-tiny,Ghost convolution
AI 理解论文
溯源树
样例
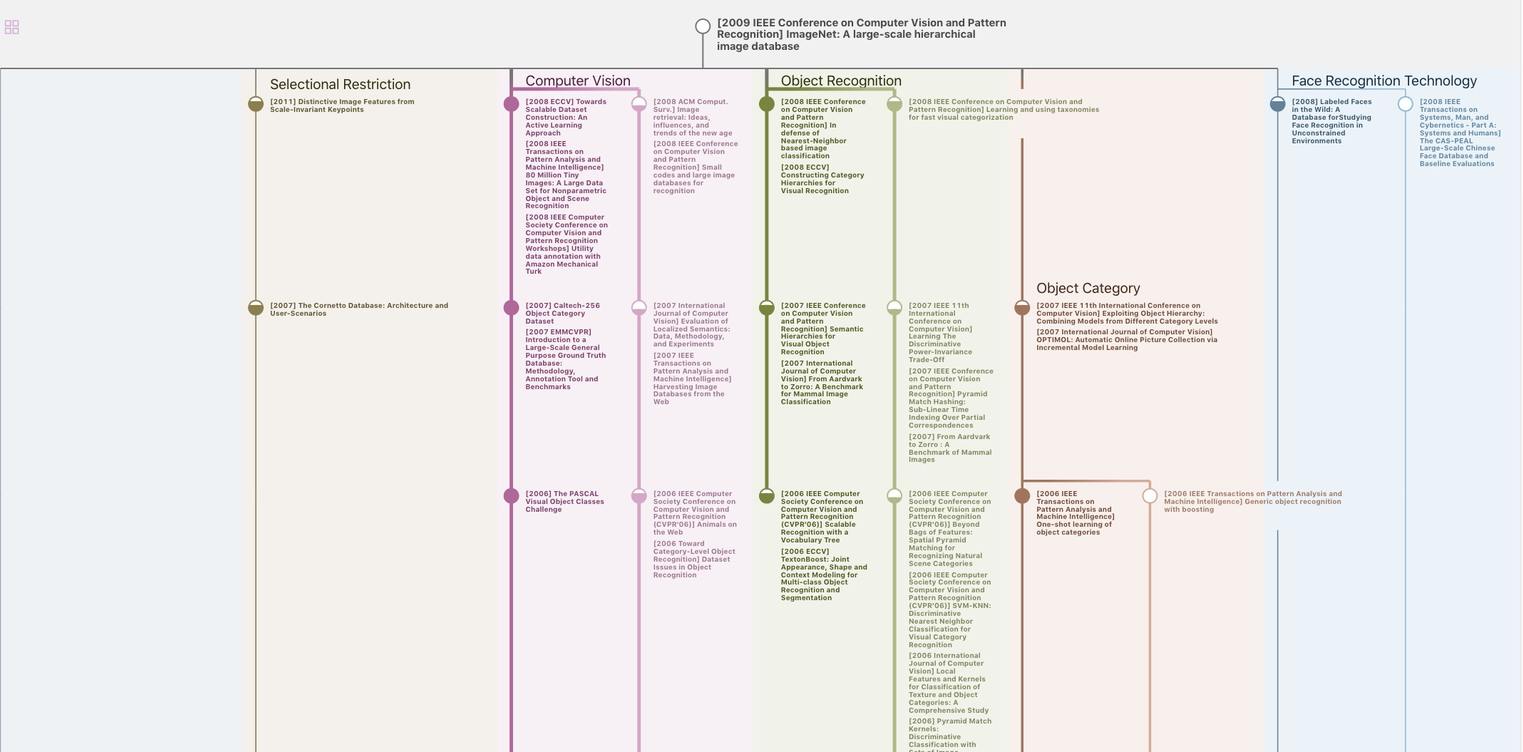
生成溯源树,研究论文发展脉络
Chat Paper
正在生成论文摘要